Hypothesis To Be Tested Definition And 4 Steps For Testing With Example
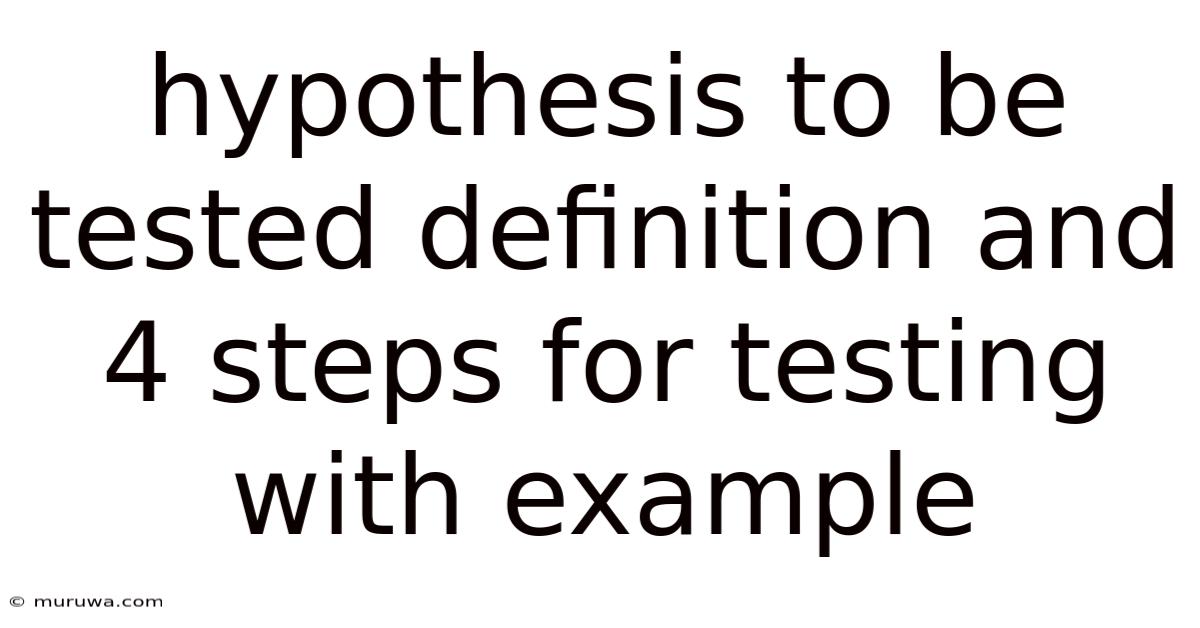
Discover more detailed and exciting information on our website. Click the link below to start your adventure: Visit Best Website meltwatermedia.ca. Don't miss out!
Table of Contents
Decoding the Hypothesis: Definition and a 4-Step Testing Process
What if the power to unlock groundbreaking discoveries lies in the rigorous testing of a well-defined hypothesis? This fundamental scientific process is the cornerstone of progress, guiding research and driving innovation across all fields.
Editor’s Note: This article provides a comprehensive guide to understanding and testing hypotheses, offering practical steps and real-world examples to enhance your research capabilities. This updated guide clarifies the process and ensures you're equipped with the knowledge to design and execute effective hypothesis tests.
Why Hypotheses Matter: Unveiling Truth Through Evidence
A hypothesis, at its core, is a testable statement predicting a relationship between two or more variables. It's not merely a guess; it's a reasoned prediction based on existing knowledge or observations, formulated to be investigated through research. Understanding and testing hypotheses is crucial for several reasons:
- Driving Research: Hypotheses provide a clear direction for research, focusing efforts on specific questions and preventing aimless exploration.
- Generating Knowledge: Rigorous testing allows for the generation of new knowledge and contributes to the expansion of scientific understanding.
- Supporting Decision-Making: In various fields, from business to healthcare, hypotheses help inform strategic decisions based on evidence rather than speculation.
- Validating Theories: Hypotheses act as building blocks for larger theories, contributing to their development, refinement, or rejection.
- Predicting Outcomes: Well-constructed hypotheses can accurately predict future outcomes, allowing for proactive planning and interventions.
Overview: What This Article Covers
This in-depth exploration of hypothesis testing will cover the following key areas:
- Definition and Types of Hypotheses: A clear understanding of what constitutes a hypothesis and its various forms.
- The Four Steps of Hypothesis Testing: A practical, step-by-step guide, illustrated with examples.
- Choosing the Right Statistical Test: An overview of the factors influencing the choice of statistical test.
- Interpreting Results and Drawing Conclusions: Understanding p-values, significance levels, and the implications of your findings.
- Real-world Examples: Illustrative scenarios demonstrating the hypothesis testing process in action.
The Research and Effort Behind the Insights
This article draws upon extensive research, incorporating principles from statistics, research methodology, and various scientific disciplines. The information presented is grounded in established methodologies and aims to provide readers with a clear, practical, and accessible understanding of hypothesis testing.
Key Takeaways:
- Definition: A concise and comprehensive definition of a hypothesis.
- Types: Distinguishing between null and alternative hypotheses, directional and non-directional hypotheses.
- Four-Step Process: A streamlined approach to hypothesis testing, explained with clarity and examples.
- Statistical Tests: A brief overview of common statistical tests and when to use them.
- Interpretation: Guidance on interpreting results and drawing meaningful conclusions.
Smooth Transition to the Core Discussion:
Now that we've established the importance of hypothesis testing, let's delve into the specifics. We will first define what constitutes a hypothesis and then unpack the four-step process of rigorously testing it.
Exploring the Key Aspects of Hypothesis Testing
1. Definition and Core Concepts:
A hypothesis is a testable statement that proposes a relationship between two or more variables. It's a tentative explanation or prediction that can be supported or refuted through empirical evidence. A well-formed hypothesis is specific, measurable, achievable, relevant, and time-bound (SMART). It's crucial to distinguish between the following:
- Null Hypothesis (H₀): This hypothesis states there is no significant relationship between the variables being studied. It's the default assumption, and the research aims to reject or fail to reject this hypothesis.
- Alternative Hypothesis (H₁ or Hₐ): This hypothesis proposes a specific relationship between the variables, contradicting the null hypothesis. It can be directional (specifying the direction of the relationship, e.g., "Group A will score higher than Group B") or non-directional (simply stating a difference exists, e.g., "There will be a difference in scores between Group A and Group B").
2. The Four Steps of Hypothesis Testing:
The process of hypothesis testing involves a systematic approach:
Step 1: Formulate the Hypotheses:
Clearly define both the null and alternative hypotheses. This step requires careful consideration of the research question and the variables involved.
- Example: A researcher wants to investigate whether a new drug reduces blood pressure.
- H₀: The new drug has no effect on blood pressure.
- H₁: The new drug reduces blood pressure.
Step 2: Set the Significance Level (α):
The significance level, typically set at 0.05 (5%), represents the probability of rejecting the null hypothesis when it is actually true (Type I error). A lower significance level reduces the chance of a Type I error but increases the chance of a Type II error (failing to reject a false null hypothesis).
Step 3: Conduct the Test and Calculate the p-value:
This involves selecting an appropriate statistical test (t-test, ANOVA, chi-square, etc.) based on the type of data and research design. The test yields a p-value, which represents the probability of obtaining the observed results (or more extreme results) if the null hypothesis were true.
- Example: The researcher conducts a t-test comparing blood pressure before and after administering the drug. The t-test yields a p-value of 0.02.
Step 4: Make a Decision:
-
If the p-value is less than the significance level (p < α), the null hypothesis is rejected, and the alternative hypothesis is accepted. This suggests statistically significant evidence supporting the alternative hypothesis.
-
If the p-value is greater than or equal to the significance level (p ≥ α), the null hypothesis is not rejected. This does not necessarily mean the null hypothesis is true; it simply means there is insufficient evidence to reject it.
-
Example: Since the p-value (0.02) is less than the significance level (0.05), the researcher rejects the null hypothesis and concludes that the new drug significantly reduces blood pressure.
Exploring the Connection Between Sample Size and Hypothesis Testing
Sample size plays a crucial role in hypothesis testing. Larger samples generally lead to more accurate and reliable results. A small sample size might not have enough power to detect a real effect, leading to a Type II error. Conversely, an excessively large sample size might detect even very small, inconsequential effects as statistically significant. Appropriate sample size determination is crucial for accurate and meaningful conclusions.
Key Factors to Consider:
- Roles and Real-World Examples: In clinical trials, sample size is meticulously calculated to ensure the study has sufficient power to detect a clinically meaningful difference in treatment outcomes. A poorly powered study might fail to demonstrate the effectiveness of a promising new drug.
- Risks and Mitigations: Using a too-small sample increases the risk of a Type II error (failing to detect a real effect). Power analysis is a crucial tool used to determine the appropriate sample size to minimize this risk.
- Impact and Implications: The choice of sample size influences the generalizability of the findings. Larger, more representative samples increase the confidence in generalizing the results to the broader population.
Conclusion: Reinforcing the Connection
The interplay between sample size and hypothesis testing is critical. Careful consideration of sample size ensures the validity and reliability of the results, leading to sound conclusions and informed decision-making.
Further Analysis: Examining Statistical Power in Greater Detail
Statistical power refers to the probability of correctly rejecting the null hypothesis when it is false. A high power study is less likely to commit a Type II error. Factors affecting power include:
- Effect size: The magnitude of the difference between groups or the strength of the relationship between variables. Larger effect sizes are easier to detect.
- Significance level (α): A lower significance level reduces power.
- Sample size: Larger samples increase power.
- Variability: Less variability within groups increases power.
FAQ Section: Answering Common Questions About Hypothesis Testing
Q: What if my p-value is exactly 0.05?
A: A p-value of exactly 0.05 is considered borderline. Researchers often use their judgment, considering factors like the practical significance of the findings, the potential for Type I error, and other available evidence, before making a decision.
Q: Can I test a hypothesis without using statistical tests?
A: While statistical tests provide a rigorous framework for hypothesis testing, some hypotheses can be evaluated using qualitative methods, such as case studies or observational studies. However, these methods generally don't offer the same level of objectivity and generalizability as quantitative methods.
Q: What is the difference between correlation and causation?
A: Correlation indicates a relationship between two variables; however, correlation does not imply causation. Just because two variables are correlated doesn't mean one causes the other. A confounding variable could be influencing both.
Practical Tips: Maximizing the Benefits of Hypothesis Testing
-
Clearly Define Variables: Ensure precise measurement and operational definitions for all variables involved in the study.
-
Choose the Right Test: Select a statistical test appropriate for the data type and research question.
-
Control for Confounding Variables: Account for extraneous factors that might influence the results.
-
Replicate the Study: Conducting multiple studies increases the confidence in the findings.
-
Interpret Results Cautiously: Avoid overstating or misinterpreting the results.
Final Conclusion: Wrapping Up with Lasting Insights
Hypothesis testing is a cornerstone of scientific inquiry, providing a framework for formulating testable predictions, gathering evidence, and drawing meaningful conclusions. By understanding the process and applying these principles effectively, researchers can drive innovation and advance knowledge across diverse disciplines. The ability to formulate strong hypotheses and interpret results critically is a skill fundamental to progress in any field that seeks to understand and explain the world around us.
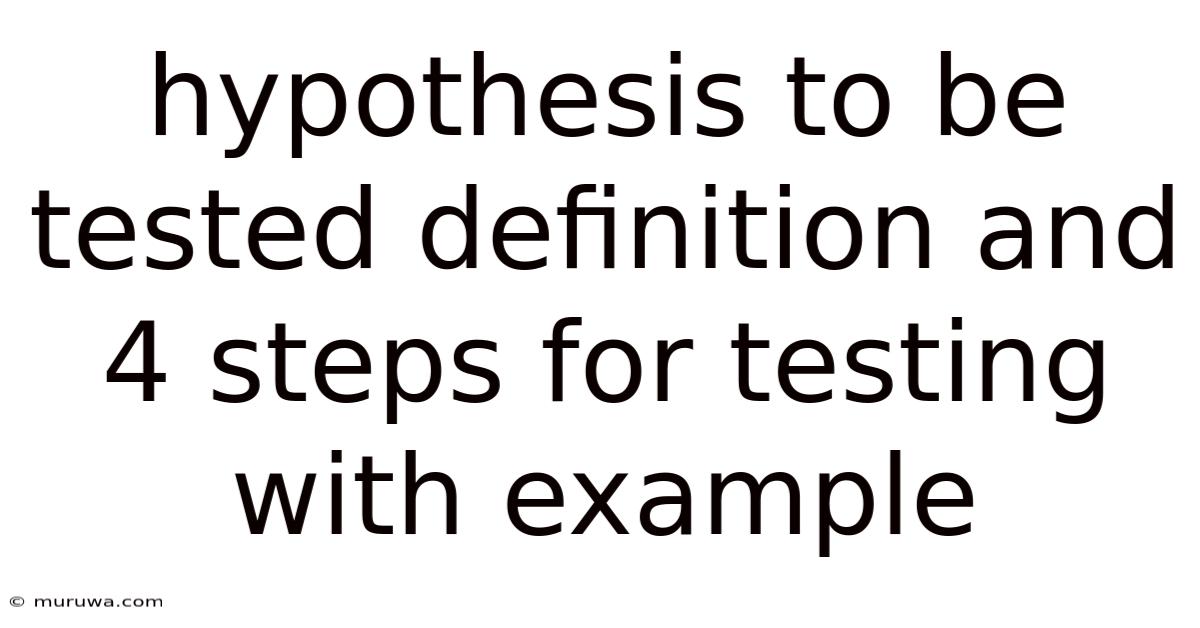
Thank you for visiting our website wich cover about Hypothesis To Be Tested Definition And 4 Steps For Testing With Example. We hope the information provided has been useful to you. Feel free to contact us if you have any questions or need further assistance. See you next time and dont miss to bookmark.
Also read the following articles
Article Title | Date |
---|---|
Income Fund Definition Types And Examples | Apr 27, 2025 |
Hook Reversal Definition | Apr 27, 2025 |
Indenture Definition And Types In Finance | Apr 27, 2025 |
Imperfect Competition Definition | Apr 27, 2025 |
Hot Issue Definition | Apr 27, 2025 |