Information Coefficient Ic Definition Example And Formula
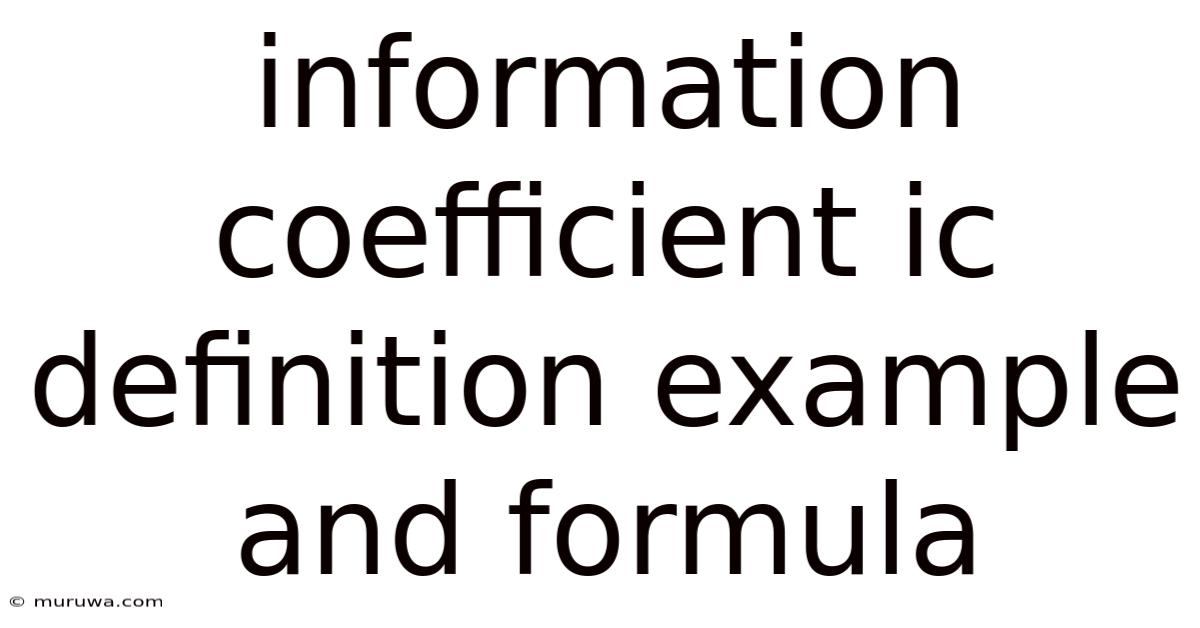
Discover more detailed and exciting information on our website. Click the link below to start your adventure: Visit Best Website meltwatermedia.ca. Don't miss out!
Table of Contents
Decoding the Information Coefficient (IC): Definition, Formula, Examples, and Applications
What if accurately predicting the future of an investment hinged on understanding a single, powerful metric? The Information Coefficient (IC), a fundamental concept in quantitative finance, provides precisely that – a measurable gauge of a predictive model's accuracy.
Editor’s Note: This article on the Information Coefficient (IC) provides a comprehensive guide to understanding its definition, formula, practical applications, and limitations. It aims to equip readers with the knowledge necessary to interpret and utilize IC effectively in investment management and other predictive modeling contexts.
Why the Information Coefficient Matters:
The Information Coefficient is a crucial metric in evaluating the predictive power of various models used in finance, particularly in quantitative investment strategies. It quantifies the correlation between a predictive signal (e.g., a model's output score) and the actual outcome (e.g., future stock returns). Understanding IC allows investors and analysts to:
- Assess model performance: A higher IC indicates a more accurate and reliable predictive model.
- Compare different models: IC facilitates the objective comparison of multiple forecasting models.
- Optimize model parameters: By analyzing IC, model parameters can be fine-tuned to enhance predictive accuracy.
- Manage risk: Understanding the IC of different factors helps in constructing diversified and well-managed portfolios.
- Improve decision-making: IC provides a quantitative basis for investment decisions, reducing reliance on intuition and subjective judgments.
Overview: What This Article Covers:
This article provides a detailed exploration of the Information Coefficient (IC), covering its definition, formula, calculation methods, practical examples, and interpretation. We will delve into its significance in various applications, discuss its limitations, and offer practical insights into its effective utilization. The article will also explore the relationship between IC and other performance metrics like the Sharpe Ratio and the information ratio.
The Research and Effort Behind the Insights:
This article is based on extensive research drawing from academic literature on quantitative finance, industry best practices, and real-world applications of the Information Coefficient. The information presented is supported by established statistical concepts and methodologies, ensuring accuracy and reliability.
Key Takeaways:
- Definition and Core Concepts: A clear understanding of the IC's fundamental principles.
- Formula and Calculation: Step-by-step guidance on calculating the IC using different methods.
- Practical Applications: Real-world examples illustrating the IC's use in various contexts.
- Interpretation and Limitations: Guidance on interpreting IC values and understanding its limitations.
- Relationship with Other Metrics: Exploring the connection between IC and other performance indicators.
Smooth Transition to the Core Discussion:
Now that we've established the importance of the Information Coefficient, let's delve into its core aspects, starting with a precise definition.
Exploring the Key Aspects of the Information Coefficient (IC):
1. Definition and Core Concepts:
The Information Coefficient (IC) measures the correlation between a predicted variable and its actual outcome. It essentially quantifies how much information a predictive model provides about the future. A higher IC indicates a stronger correlation and, consequently, a more accurate model. Unlike correlation, which ranges from -1 to +1, the IC is typically presented as an absolute value, representing the strength of the predictive signal regardless of its direction. An IC of 0.1, for example, suggests that the model provides a weak but positive predictive signal.
2. Formula and Calculation:
The IC is calculated as the correlation between the model's predicted values and the actual observed values. This is typically done using Pearson's correlation coefficient. The formula is:
IC = corr(Predicted Value, Actual Value)
Where:
corr()
denotes the Pearson correlation coefficient.Predicted Value
represents the model's predicted value for a specific outcome.Actual Value
represents the actual observed value of the outcome.
It's important to note that the actual and predicted values should be standardized (e.g., z-scored) before calculating the correlation to ensure a fair comparison, especially when dealing with different scales or units. Standardization involves converting the data to have a mean of 0 and a standard deviation of 1.
3. Practical Applications:
The IC finds widespread application in several areas of quantitative finance, including:
- Stock Selection: Models predicting future stock returns can be evaluated using IC. A high IC indicates a strong relationship between the model's predictions and actual returns.
- Factor Models: The IC of individual factors within a multi-factor model helps determine their predictive power and contribution to overall portfolio performance.
- Portfolio Optimization: IC can be incorporated into portfolio optimization algorithms to enhance diversification and risk-adjusted returns.
- Risk Management: Predictive models for assessing credit risk or market risk can be evaluated using IC.
- Algorithmic Trading: IC is crucial in evaluating the performance of algorithmic trading strategies.
4. Interpretation and Limitations:
Interpreting IC requires understanding its context. A high IC (e.g., >0.1) suggests a strong predictive signal, while a low IC (e.g., <0.05) might indicate a weak or negligible signal. However, the interpretation must consider factors like the dataset's size, the model's complexity, and the specific application.
Limitations of IC include:
- Overfitting: Complex models might achieve a high IC on the training data but fail to generalize to unseen data.
- Data Snooping: Repeatedly testing and refining a model on the same data can lead to an overly optimistic IC.
- Time Dependency: The IC of a model can vary over time due to changes in market conditions or the underlying data-generating process.
- Correlation ≠ Causation: A high IC merely indicates a correlation; it doesn't necessarily imply causality.
5. Relationship with Other Metrics:
The IC is closely related to other performance metrics, particularly the Sharpe Ratio and the Information Ratio. While the Sharpe Ratio measures risk-adjusted return, the Information Ratio compares the excess return of a strategy to its benchmark. The IC is a component contributing to the Information Ratio. A higher IC generally contributes to a higher Information Ratio, indicating better investment performance relative to a benchmark.
Exploring the Connection Between 'Signal Noise Ratio' and 'Information Coefficient':
The signal-to-noise ratio (SNR) and the Information Coefficient (IC) are closely related concepts in evaluating the effectiveness of a predictive model. The SNR represents the ratio of the power of the relevant signal to the power of background noise. In the context of investment modeling, the signal is the predictive signal from the model, and the noise represents random fluctuations or errors. A higher SNR indicates a clearer signal relative to the noise.
The IC, on the other hand, directly measures the correlation between the predicted and actual values. A higher IC implies a stronger relationship between the model's predictions and the actual outcomes.
Key Factors to Consider:
Roles and Real-World Examples: A high SNR generally translates into a higher IC. For example, in a stock selection model, a high SNR (clear signal from the model) results in a higher correlation (IC) between the model's predictions and the actual stock returns. Conversely, a low SNR (weak signal amidst significant noise) translates into a low IC.
Risks and Mitigations: Overfitting can lead to a high IC but a low SNR out-of-sample. Regularization techniques and robust model selection procedures can mitigate this risk.
Impact and Implications: Understanding both SNR and IC provides a more comprehensive assessment of a predictive model's performance. A high IC alone might be misleading if it's driven by overfitting or data snooping. Considering the SNR gives a more realistic picture of the model's ability to generalize to new data.
Conclusion: Reinforcing the Connection:
The relationship between SNR and IC is essential for assessing the robustness and generalizability of predictive models. A model with a high SNR is likely to exhibit a high IC, but the converse is not always true. Thorough model evaluation should involve both metrics to ensure a more reliable and informed assessment of predictive power.
Further Analysis: Examining 'Signal Noise Ratio' in Greater Detail:
The signal-to-noise ratio (SNR) is calculated as the ratio of the variance of the signal to the variance of the noise. In the context of financial modeling, it can be estimated using techniques such as regression analysis or time series decomposition. A higher SNR implies that the model's predictive signal is dominant over the random noise in the data, thereby improving the model's reliability and predictive accuracy.
FAQ Section: Answering Common Questions About Information Coefficient:
Q: What is the ideal Information Coefficient (IC) value?
A: There's no universally "ideal" IC value. The interpretation depends heavily on the context. An IC above 0.1 is generally considered good, while an IC above 0.25 is excellent. However, even a smaller IC can be valuable if the trading strategy has a high Sharpe Ratio or Information Ratio.
Q: How can I improve the IC of my predictive model?
A: Improving the IC requires improving the model's ability to predict the future accurately. This involves techniques like feature engineering (selecting relevant variables), model selection (choosing an appropriate model structure), and parameter tuning (optimizing the model's parameters). Addressing overfitting is also crucial.
Q: What are the limitations of using the IC as the sole metric for model evaluation?
A: The IC should not be the sole metric for evaluating a predictive model. Other considerations include the model's stability over time, its robustness to different data samples, and its potential for overfitting. It's essential to consider risk-adjusted performance metrics along with the IC.
Practical Tips: Maximizing the Benefits of the Information Coefficient:
- Data Preprocessing: Ensure your data is clean, consistent, and appropriately standardized before calculating the IC.
- Model Selection: Choose a model suitable for your data and predictive task.
- Regularization: Employ regularization techniques to prevent overfitting and improve generalization.
- Cross-Validation: Use cross-validation to assess the model's performance on unseen data.
- Out-of-Sample Testing: Evaluate the model's performance on a separate hold-out sample to avoid overly optimistic estimates.
- Contextual Interpretation: Interpret the IC within the specific context of your application.
Final Conclusion: Wrapping Up with Lasting Insights:
The Information Coefficient (IC) is a powerful tool for evaluating the predictive accuracy of models in quantitative finance. Understanding its calculation, interpretation, and limitations is crucial for developing effective investment strategies. However, it's crucial to remember that the IC is just one piece of the puzzle. Combining it with other performance metrics, risk management considerations, and sound judgment is essential for making informed investment decisions. By leveraging the IC effectively, investors and analysts can enhance their ability to predict market movements, manage risk more efficiently, and ultimately achieve better investment outcomes.
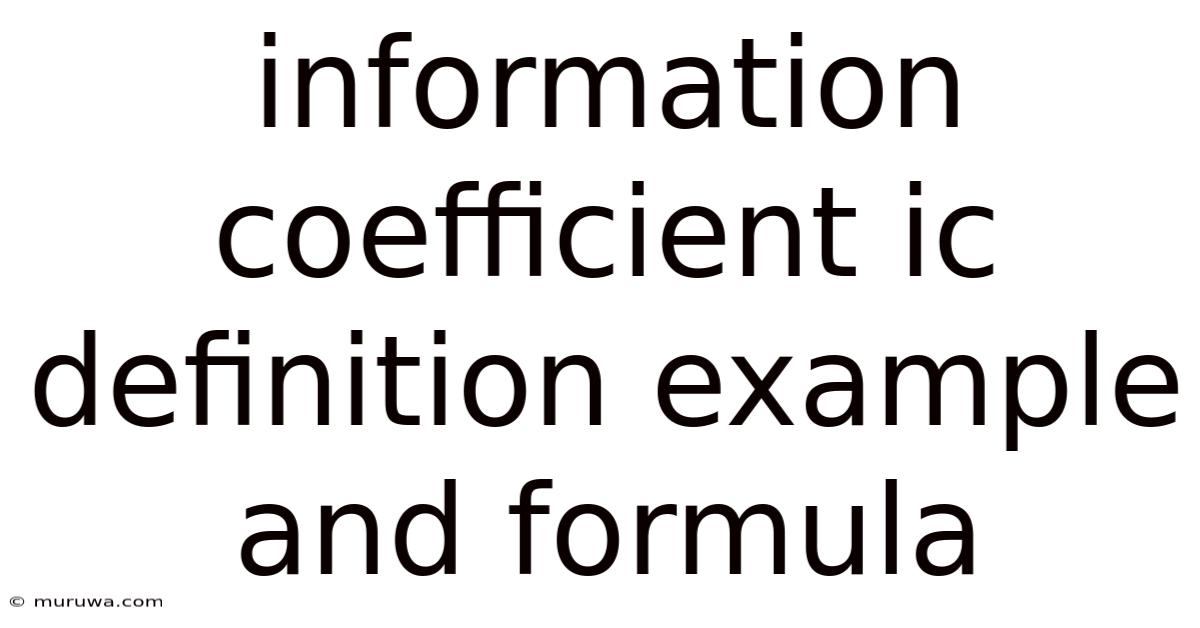
Thank you for visiting our website wich cover about Information Coefficient Ic Definition Example And Formula. We hope the information provided has been useful to you. Feel free to contact us if you have any questions or need further assistance. See you next time and dont miss to bookmark.
Also read the following articles
Article Title | Date |
---|---|
International Foreign Exchange Master Agreement Ifema Definition | Apr 27, 2025 |
Hook Reversal Definition | Apr 27, 2025 |
International Securities Exchange Ise Definition | Apr 27, 2025 |
Imprest Definition Uses And How It Works | Apr 27, 2025 |
In The Money Definition Call Put Options And Example | Apr 27, 2025 |