What Is Supply Chain Forecasting
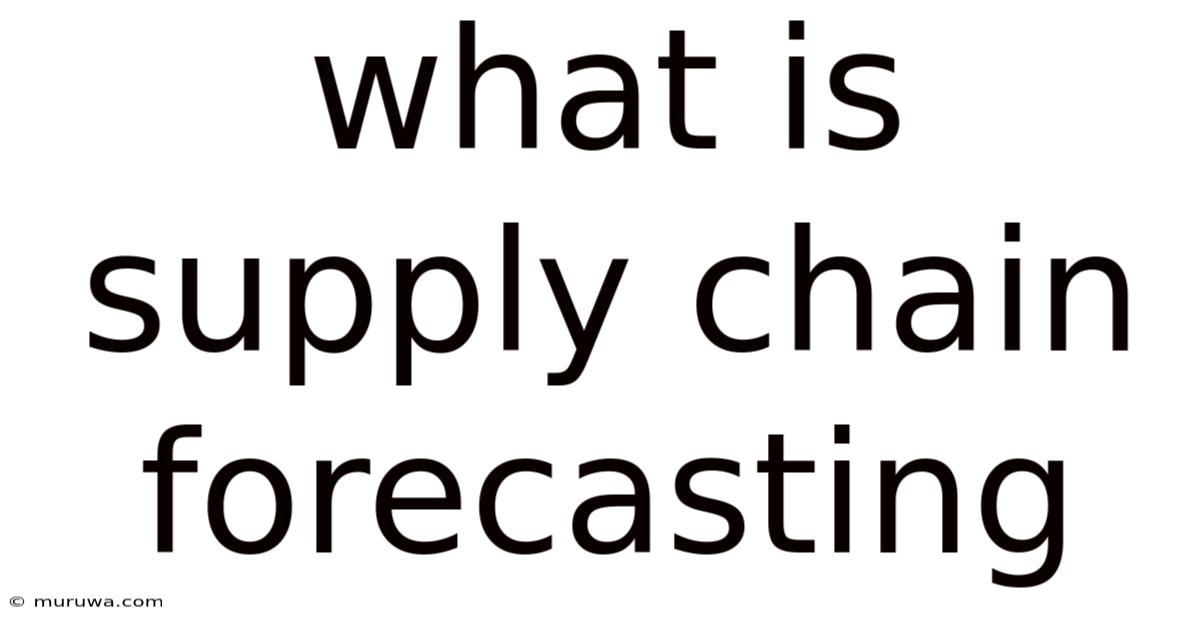
Discover more detailed and exciting information on our website. Click the link below to start your adventure: Visit Best Website meltwatermedia.ca. Don't miss out!
Table of Contents
Decoding the Crystal Ball: A Deep Dive into Supply Chain Forecasting
What if businesses could accurately predict future demand, ensuring optimal inventory levels and avoiding costly disruptions? Supply chain forecasting, a powerful analytical tool, offers precisely this capability, transforming how organizations manage their operations and gain a competitive edge.
Editor’s Note: This comprehensive article on supply chain forecasting provides up-to-date insights and strategies for businesses seeking to optimize their operations and navigate the complexities of modern supply chains. Published [Date], this resource offers a practical and in-depth understanding of this crucial business function.
Why Supply Chain Forecasting Matters: Relevance, Practical Applications, and Industry Significance
Supply chain forecasting is no longer a luxury; it's a necessity in today's dynamic business environment. The ability to accurately predict future demand directly impacts a company's profitability, efficiency, and resilience. Accurate forecasting minimizes stockouts, reduces waste from excess inventory, optimizes production schedules, and enables proactive risk management. Its applications are far-reaching, influencing decisions across various departments, from procurement and production to logistics and sales. Industries ranging from manufacturing and retail to healthcare and technology rely heavily on effective forecasting methodologies to maintain competitiveness and satisfy customer expectations.
Overview: What This Article Covers
This article explores the multifaceted world of supply chain forecasting, covering fundamental concepts, various forecasting techniques, crucial considerations, and best practices. Readers will gain a practical understanding of how to select appropriate methodologies, interpret results, and integrate forecasting into their overall supply chain strategy. We will also analyze the role of technology and data analytics in enhancing forecasting accuracy and efficiency.
The Research and Effort Behind the Insights
This article is the product of extensive research, incorporating insights from leading supply chain management experts, academic publications, industry reports, and real-world case studies. The information presented is supported by credible sources, ensuring accuracy and providing readers with a robust and reliable understanding of supply chain forecasting.
Key Takeaways:
- Definition and Core Concepts: A clear definition of supply chain forecasting and its underlying principles.
- Forecasting Methods: An exploration of various quantitative and qualitative forecasting techniques.
- Data Sources and Quality: The importance of reliable and accurate data for effective forecasting.
- Technological Advancements: The role of AI, machine learning, and big data analytics in modern forecasting.
- Challenges and Solutions: Addressing common obstacles and strategies for overcoming them.
- Integrating Forecasting into Supply Chain Strategy: Developing a holistic approach to forecasting and its integration within the broader supply chain.
Smooth Transition to the Core Discussion:
Having established the importance of supply chain forecasting, let's delve into the core concepts and methods that underpin this critical business function.
Exploring the Key Aspects of Supply Chain Forecasting
1. Definition and Core Concepts:
Supply chain forecasting is the process of predicting future demand for goods and services across the entire supply chain. It involves analyzing historical data, market trends, seasonal patterns, and other relevant factors to generate accurate demand projections. The goal is to optimize inventory levels, production schedules, and logistics operations, thereby minimizing costs and maximizing customer satisfaction. Accurate forecasting reduces the bullwhip effect—the amplification of demand variability as it moves upstream through the supply chain.
2. Forecasting Methods:
A variety of forecasting methods exist, categorized broadly as qualitative and quantitative:
-
Qualitative Forecasting: Relies on expert judgment, intuition, and subjective opinions. Methods include Delphi method, market research, and sales force composite. These are useful when historical data is limited or unreliable.
-
Quantitative Forecasting: Employs mathematical models and statistical techniques to analyze historical data and predict future demand. Common methods include:
-
Time Series Analysis: Analyzes historical demand data to identify patterns and trends, projecting them into the future. Techniques include moving averages, exponential smoothing, ARIMA models.
-
Causal Forecasting: Identifies relationships between demand and other factors (e.g., price, promotions, economic indicators) to predict future demand. Methods include regression analysis and econometric modeling.
-
Simulation: Uses computer models to simulate various scenarios and assess the impact of different factors on demand.
-
3. Data Sources and Quality:
The accuracy of any forecast depends heavily on the quality and reliability of the input data. Key data sources include:
- Historical Sales Data: Provides a foundation for understanding past demand patterns.
- Market Research: Offers insights into consumer preferences and future market trends.
- Economic Indicators: Provides a broader context for understanding overall economic conditions.
- Point-of-Sale (POS) Data: Offers real-time information on sales and customer behavior.
- Social Media Data: Can reveal emerging trends and consumer sentiment.
Data cleansing and validation are crucial steps to ensure accuracy. Outliers and errors must be identified and addressed before applying forecasting models.
4. Technological Advancements:
Technology plays a pivotal role in modern supply chain forecasting. Artificial intelligence (AI), machine learning (ML), and big data analytics are transforming the field, enabling:
- Improved Accuracy: AI and ML algorithms can identify complex patterns and relationships in large datasets that traditional methods might miss.
- Increased Efficiency: Automated forecasting processes reduce manual effort and streamline workflows.
- Real-Time Insights: Real-time data analysis allows for dynamic adjustments to forecasts based on current market conditions.
- Predictive Analytics: Forecasting models can now predict not just demand but also potential disruptions and risks.
5. Challenges and Solutions:
Several challenges can hinder the effectiveness of supply chain forecasting:
- Data Inaccuracy and Incompleteness: Addressing this requires robust data management practices and data cleansing techniques.
- Demand Volatility: Unexpected events (e.g., pandemics, natural disasters) can significantly impact demand, requiring flexible forecasting methods.
- Lead Time Variability: Fluctuations in lead times can complicate inventory management and necessitate more sophisticated forecasting models.
- Lack of Collaboration: Effective forecasting requires collaboration across different departments and even with suppliers.
Solutions involve implementing robust data management systems, using advanced forecasting techniques, fostering cross-functional collaboration, and employing scenario planning to address uncertainty.
6. Integrating Forecasting into Supply Chain Strategy:
Supply chain forecasting shouldn't exist in isolation; it must be integrated into the broader supply chain strategy. This involves:
- Defining clear objectives: What are the specific goals of the forecasting process (e.g., minimizing inventory costs, improving service levels)?
- Selecting appropriate methods: Choosing forecasting techniques that align with the specific characteristics of the product, industry, and data available.
- Establishing key performance indicators (KPIs): Measuring the accuracy and effectiveness of the forecasting process (e.g., forecast error, inventory turnover).
- Regular monitoring and evaluation: Continuously monitoring the performance of the forecasting process and making adjustments as needed.
Closing Insights: Summarizing the Core Discussion
Supply chain forecasting is a dynamic and evolving field, crucial for optimizing operations and maintaining competitiveness. By understanding the various methods, challenges, and technological advancements, businesses can develop robust forecasting processes that enhance efficiency, reduce costs, and improve customer satisfaction.
Exploring the Connection Between Data Analytics and Supply Chain Forecasting
Data analytics plays a pivotal role in enhancing the accuracy and effectiveness of supply chain forecasting. It allows businesses to analyze vast amounts of data from various sources, identify hidden patterns and trends, and develop more accurate demand predictions.
Key Factors to Consider:
-
Roles and Real-World Examples: Data analytics helps identify seasonality, trends, and correlations between different variables impacting demand. For example, a retailer might use sales data, weather patterns, and social media sentiment to predict demand for seasonal clothing.
-
Risks and Mitigations: Poor data quality, inaccurate data cleaning, and the lack of appropriate analytical skills can lead to inaccurate forecasts. Mitigation involves investing in robust data infrastructure, data cleaning processes, and skilled data analysts.
-
Impact and Implications: Effective data-driven forecasting reduces inventory costs, improves customer service levels, and optimizes production planning, ultimately increasing profitability.
Conclusion: Reinforcing the Connection
The synergistic relationship between data analytics and supply chain forecasting is undeniable. By leveraging the power of data analytics, organizations can move beyond simplistic forecasting models to build more accurate, responsive, and resilient supply chains.
Further Analysis: Examining Big Data in Greater Detail
Big data, with its volume, velocity, and variety, presents both opportunities and challenges for supply chain forecasting. Its vast datasets allow for the development of sophisticated predictive models capable of handling complexity and uncertainty. However, effective utilization requires robust infrastructure, advanced analytical skills, and careful data governance.
FAQ Section: Answering Common Questions About Supply Chain Forecasting
-
What is the most accurate forecasting method? There's no single "most accurate" method. The best approach depends on factors like data availability, forecast horizon, and demand variability.
-
How often should forecasts be updated? The frequency of updates depends on the product and industry. Some industries require daily or even hourly updates, while others might update weekly or monthly.
-
What is the role of collaboration in forecasting? Effective forecasting requires collaboration between various departments (e.g., sales, marketing, operations) and even external partners (e.g., suppliers).
Practical Tips: Maximizing the Benefits of Supply Chain Forecasting
- Invest in robust data infrastructure: Ensure access to high-quality, reliable data from various sources.
- Employ a variety of forecasting methods: Combine quantitative and qualitative methods to leverage different strengths.
- Regularly evaluate forecast accuracy: Track KPIs to identify areas for improvement and make adjustments as needed.
- Foster collaboration across departments: Ensure open communication and information sharing across the supply chain.
Final Conclusion: Wrapping Up with Lasting Insights
Supply chain forecasting is a cornerstone of effective supply chain management. By leveraging advancements in data analytics, technology, and collaborative strategies, businesses can unlock significant benefits, improve efficiency, and gain a competitive edge in today's dynamic market. The journey towards accurate and impactful forecasting is an ongoing process of refinement, adaptation, and continuous improvement. Embrace the power of prediction, and watch your supply chain transform.
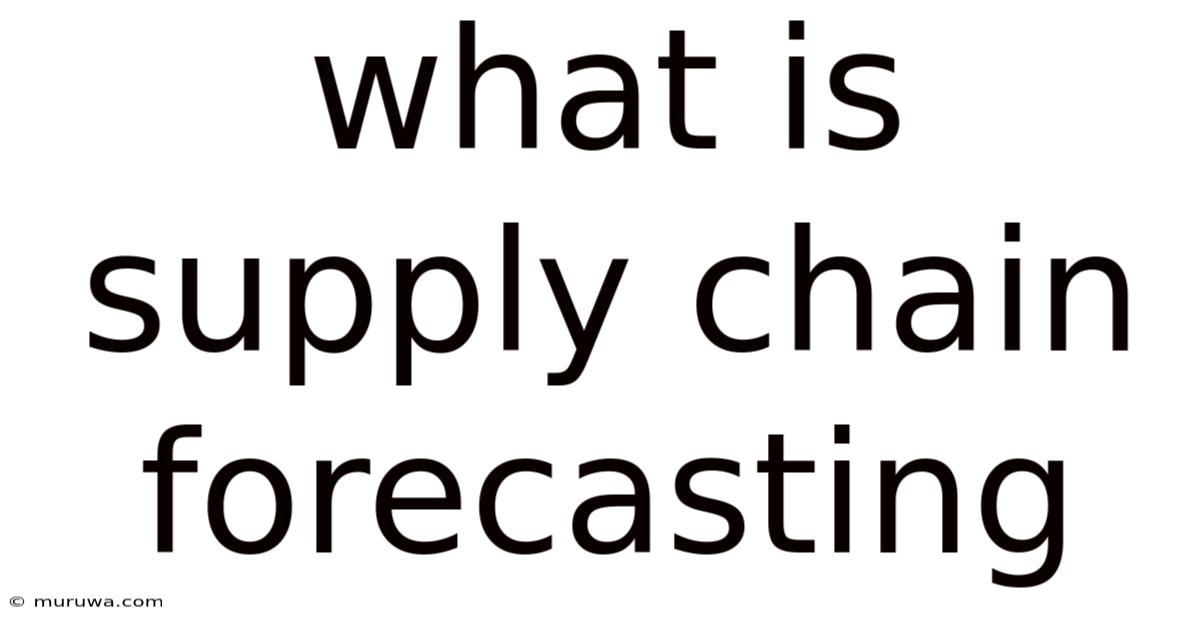
Thank you for visiting our website wich cover about What Is Supply Chain Forecasting. We hope the information provided has been useful to you. Feel free to contact us if you have any questions or need further assistance. See you next time and dont miss to bookmark.
Also read the following articles
Article Title | Date |
---|---|
What Is A Lirp Investment | Apr 22, 2025 |
Boolean Algebra Definition | Apr 22, 2025 |
How To Save Money In Inflation | Apr 22, 2025 |
What Is Investment Analysis | Apr 22, 2025 |
Book To Ship Ratio Definition | Apr 22, 2025 |