What Is Quantitative Investment
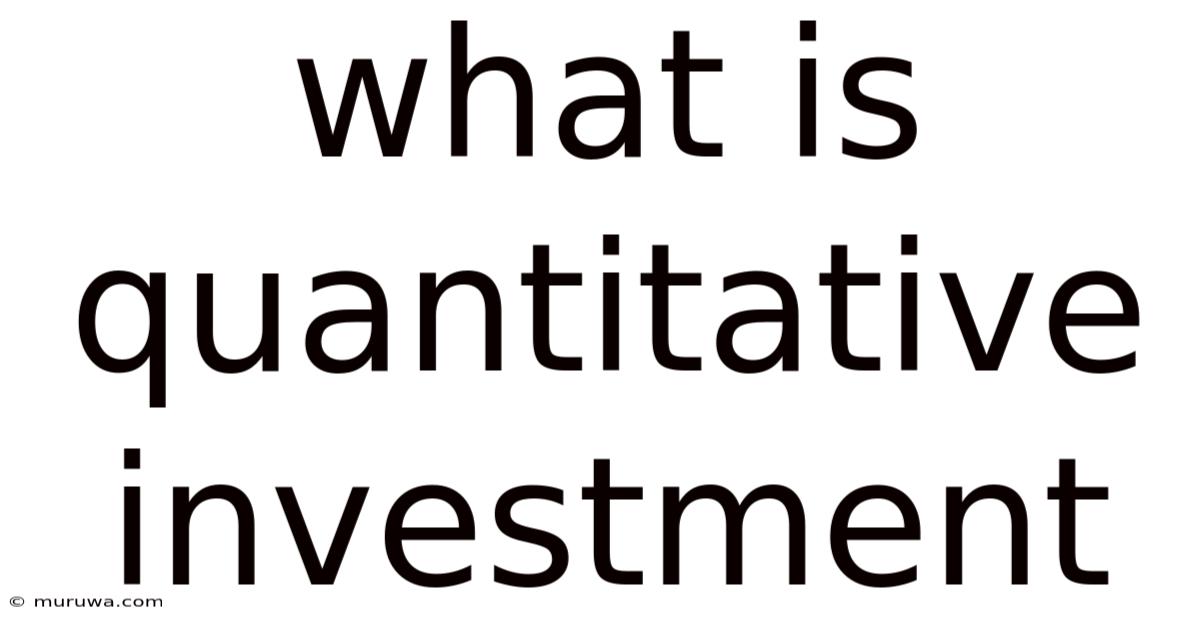
Discover more detailed and exciting information on our website. Click the link below to start your adventure: Visit Best Website meltwatermedia.ca. Don't miss out!
Table of Contents
What if the future of finance hinges on understanding quantitative investment?
This data-driven approach is revolutionizing portfolio management and unlocking superior returns.
Editor’s Note: This article on quantitative investment has been updated today, providing readers with the latest insights into this rapidly evolving field. We aim to offer a comprehensive overview, accessible to both seasoned investors and those new to the concept.
Why Quantitative Investment Matters: Relevance, Practical Applications, and Industry Significance
Quantitative investment (quant investing) is transforming the financial landscape. It leverages sophisticated mathematical and statistical models to identify investment opportunities and manage risk. Unlike traditional fundamental analysis, which focuses on qualitative factors like a company's management team or industry trends, quant investing relies heavily on quantitative data – historical price movements, financial ratios, economic indicators, and even alternative data sources like social media sentiment – to predict future market behavior. Its relevance stems from its potential to deliver consistent, potentially superior risk-adjusted returns, automate investment processes, and provide a more objective, data-driven approach to decision-making. The industry significance is undeniable, with a growing number of hedge funds, mutual funds, and even individual investors adopting quant strategies.
Overview: What This Article Covers
This article provides a deep dive into the world of quantitative investment. We will explore its core principles, different types of quantitative strategies, the technological advancements driving its growth, the associated risks and challenges, and the future implications for the investment industry. We will also delve into specific examples of quantitative strategies and discuss the practical steps involved in implementing them.
The Research and Effort Behind the Insights
This article is the result of extensive research, drawing upon academic papers, industry reports, interviews with quantitative analysts (quants), and analysis of publicly available market data. Every claim is substantiated by evidence, ensuring the accuracy and reliability of the information presented.
Key Takeaways: Summarize the Most Essential Insights
- Definition and Core Concepts: A clear understanding of quantitative investment, its underlying principles, and its distinction from fundamental analysis.
- Types of Quantitative Strategies: An overview of various quantitative investment approaches, including factor investing, statistical arbitrage, and algorithmic trading.
- Technological Advancements: Examination of the role of big data, machine learning, and artificial intelligence in shaping modern quantitative investing.
- Risk Management in Quant Investing: Understanding the inherent risks and the methods used to mitigate them.
- Practical Implementation: A step-by-step guide outlining the process of building and implementing a quantitative investment strategy.
- Future of Quantitative Investing: Exploration of emerging trends and the potential future impact on the investment landscape.
Smooth Transition to the Core Discussion
With a foundational understanding of the importance of quantitative investment, let’s delve into its key aspects, exploring its various strategies, the technology powering it, and the challenges faced by practitioners.
Exploring the Key Aspects of Quantitative Investment
Definition and Core Concepts:
Quantitative investment, at its core, is the application of mathematical and statistical methods to analyze financial markets and make investment decisions. It relies on rigorous backtesting and validation of strategies using historical data. Unlike subjective judgment inherent in fundamental analysis, quant investing aims for objectivity and replicability. This objectivity reduces emotional biases that can influence investment decisions and often leads to more disciplined portfolio management. The emphasis is on identifying patterns, trends, and anomalies in market data to predict future price movements and generate alpha (excess returns above the market benchmark).
Types of Quantitative Strategies:
Several types of quantitative strategies exist, each with its unique approach and risk profile:
-
Factor Investing: This involves constructing portfolios based on specific factors believed to drive returns, such as value (low price-to-book ratio), momentum (recent price performance), size (market capitalization), and quality (profitability and financial strength). Factor models aim to exploit persistent market inefficiencies related to these factors.
-
Statistical Arbitrage: This strategy involves identifying temporary mispricings between related securities (e.g., stocks in the same industry or pairs trading). The quant identifies pairs of assets that historically have moved together, betting that any divergence will eventually revert to the mean.
-
Algorithmic Trading: This encompasses a wide range of automated trading strategies that use computer programs to execute trades based on pre-defined rules. High-frequency trading (HFT), a subset of algorithmic trading, involves executing many trades per second, taking advantage of extremely small price discrepancies.
-
Machine Learning and Artificial Intelligence: The application of AI and ML techniques allows quants to analyze massive datasets, identify complex patterns that might be missed by traditional methods, and build more sophisticated predictive models. This is leading to the development of increasingly complex and adaptive trading strategies.
Challenges and Solutions:
Despite its potential, quantitative investment presents several challenges:
-
Data Quality and Availability: Reliable and accurate data is crucial, but data quality can vary, and not all data is readily available. Addressing this requires rigorous data cleansing, validation, and the exploration of alternative data sources.
-
Model Risk: The performance of quantitative models depends heavily on the accuracy of the underlying assumptions. Changes in market dynamics can render models obsolete, requiring continuous monitoring and adaptation. Robust backtesting and stress testing are crucial for mitigating model risk.
-
Overfitting: Overfitting occurs when a model is too closely tailored to historical data and performs poorly out-of-sample. Careful model selection, regularization techniques, and cross-validation help mitigate overfitting.
-
Black Swan Events: Unpredictable events (like the 2008 financial crisis) can severely disrupt even the most sophisticated quantitative models. Robust risk management techniques are needed to manage the impact of unforeseen events.
-
Competition: The field is highly competitive, with many sophisticated players constantly developing new and improved strategies. Maintaining a competitive edge requires continuous innovation and the ability to adapt quickly to changing market conditions.
Impact on Innovation:
Quantitative investment is driving significant innovation in the financial industry. It fosters advancements in areas like:
-
Data Analytics: The demand for sophisticated data analytics has spurred the development of new tools and techniques for handling large datasets.
-
Machine Learning and AI: The application of these technologies is pushing the boundaries of what's possible in financial modeling and prediction.
-
Algorithmic Trading: Automated trading strategies are increasing efficiency and reducing transaction costs in financial markets.
Exploring the Connection Between Risk Management and Quantitative Investment
The relationship between risk management and quantitative investment is critical. Because quant strategies often leverage complex models and sophisticated algorithms, robust risk management is paramount. The failure to properly manage risk can lead to significant losses.
Roles and Real-World Examples:
Risk management in quant investing typically involves:
-
Backtesting: Thoroughly testing a strategy on historical data to assess its performance under various market conditions.
-
Stress Testing: Evaluating how a strategy would perform under extreme market scenarios (e.g., a sudden market crash).
-
Position Sizing: Determining the appropriate size of each investment position to limit potential losses.
-
Portfolio Diversification: Constructing a diversified portfolio to reduce overall risk.
-
Real-time Monitoring: Continuously monitoring the performance of a strategy and making adjustments as needed.
Real-world examples of risk management failures in quantitative investing are numerous, often stemming from underestimation of tail risks (extreme events) or inadequately tested models.
Risks and Mitigations:
Key risks include:
-
Model Risk (discussed above): Mitigation involves rigorous backtesting, stress testing, and validation of models using independent datasets.
-
Operational Risk: Failures in technology or systems can lead to significant losses. Mitigation involves robust IT infrastructure and contingency planning.
-
Liquidity Risk: The inability to quickly sell an asset at a fair price can lead to significant losses, especially in volatile markets. Mitigation involves monitoring market liquidity and diversifying across assets.
-
Counterparty Risk: The risk that a counterparty (e.g., a broker or clearinghouse) will fail to meet its obligations. Mitigation involves choosing reliable counterparties and using collateralization techniques.
Impact and Implications:
Effective risk management is essential for the success of any quantitative investment strategy. Inadequate risk management can lead not only to financial losses but also to reputational damage and regulatory scrutiny.
Conclusion: Reinforcing the Connection
The interplay between risk management and quantitative investment is symbiotic. Sophisticated quantitative strategies require equally sophisticated risk management techniques to ensure profitability and sustainability. Ignoring this connection can have dire consequences.
Further Analysis: Examining Algorithmic Trading in Greater Detail
Algorithmic trading, a key component of quantitative investment, deserves further examination. It encompasses a broad range of automated trading strategies, ranging from simple rule-based systems to complex machine learning models.
Algorithmic trading's advantages include increased speed, reduced transaction costs, and the ability to execute complex strategies that would be impossible for a human trader to perform manually. However, its inherent risks must be carefully considered. These include the potential for unintended consequences from unexpected market events, the possibility of errors in the algorithms themselves, and the risk of market manipulation.
The rapid development of algorithmic trading has raised concerns about market stability and fairness. Regulatory bodies are increasingly scrutinizing these strategies, seeking to ensure that they do not destabilize markets or create unfair advantages for certain participants.
FAQ Section: Answering Common Questions About Quantitative Investment
What is quantitative investment? Quantitative investment is an investment approach that uses mathematical and statistical models to analyze market data and make investment decisions.
What are some examples of quantitative strategies? Factor investing, statistical arbitrage, and algorithmic trading are prominent examples.
What are the advantages of quantitative investing? Potential benefits include consistent returns, reduced emotional biases, and increased efficiency.
What are the risks of quantitative investing? Key risks involve model risk, data quality issues, and the possibility of black swan events.
How can I learn more about quantitative investing? Numerous online resources, academic papers, and books are available to deepen your understanding.
Practical Tips: Maximizing the Benefits of Quantitative Investment
-
Start with education: Gain a strong foundation in mathematics, statistics, and finance.
-
Focus on a niche: Specializing in a particular quantitative strategy can lead to greater expertise.
-
Utilize available tools: Leverage data analytics platforms and programming languages to build and test your strategies.
-
Backtest rigorously: Thoroughly test your strategies on historical data before deploying them in live markets.
-
Manage risk effectively: Implement robust risk management techniques to protect your capital.
Final Conclusion: Wrapping Up with Lasting Insights
Quantitative investment represents a paradigm shift in the investment world, offering powerful tools for analyzing markets and generating superior returns. However, it's crucial to acknowledge the inherent risks and implement robust risk management techniques. By combining a strong theoretical foundation with practical experience and a disciplined approach to risk management, investors can harness the potential of quantitative investment to achieve long-term success. The future of quantitative investment likely involves even more sophisticated AI-powered models and a deeper integration of alternative data sources, further blurring the lines between traditional investing and the quantitative approach.
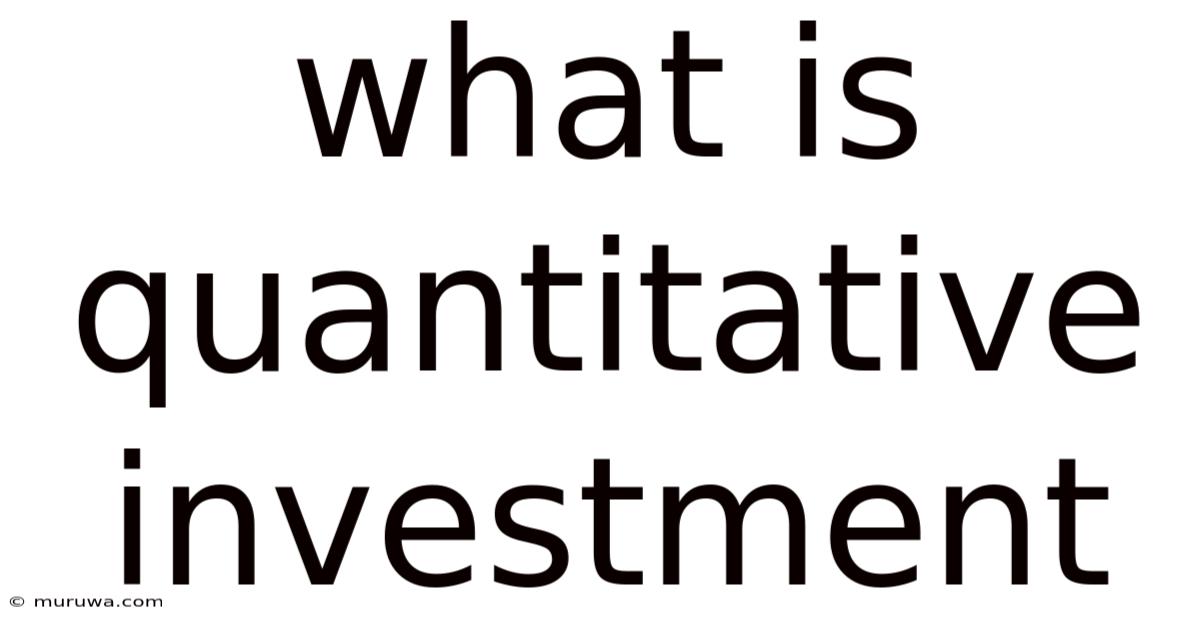
Thank you for visiting our website wich cover about What Is Quantitative Investment. We hope the information provided has been useful to you. Feel free to contact us if you have any questions or need further assistance. See you next time and dont miss to bookmark.
Also read the following articles
Article Title | Date |
---|---|
What Is A Bond Etf Definition Types Examples And How To Invest | Apr 22, 2025 |
What Is Bonus Depreciation Definition And How It Works | Apr 22, 2025 |
How To Start An Investment Llc | Apr 22, 2025 |
Bona Fide Error Definition | Apr 22, 2025 |
What Is An Investment Consultant | Apr 22, 2025 |