What Is Platykurtic Definition Examples And Other Distributions
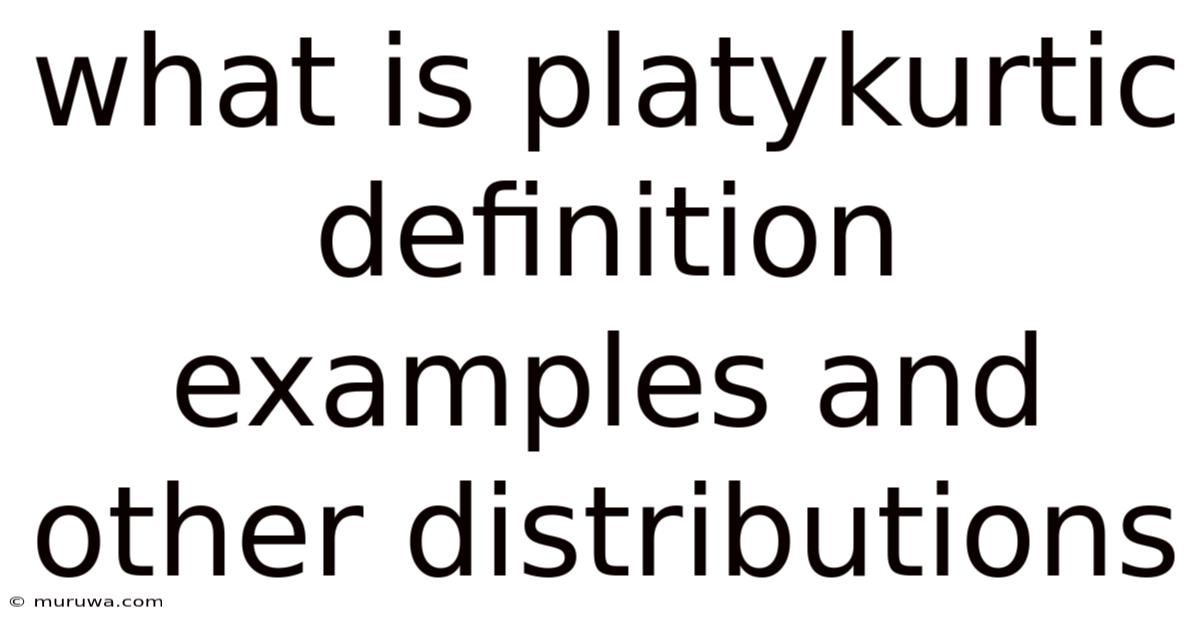
Discover more detailed and exciting information on our website. Click the link below to start your adventure: Visit Best Website meltwatermedia.ca. Don't miss out!
Table of Contents
Understanding Platykurtic Distributions: Definition, Examples, and Comparisons
What if the seemingly simple concept of "kurtosis" holds the key to unlocking deeper insights into data distributions? Platykurtic distributions, characterized by their low kurtosis, reveal unique patterns and behaviors that are crucial for accurate statistical analysis and informed decision-making.
Editor’s Note: This article on platykurtic distributions provides a comprehensive overview of this important statistical concept, including its definition, practical examples, and comparisons with other distribution types. The information presented is up-to-date and based on established statistical principles.
Why Platykurtic Distributions Matter:
Platykurtosis, a measure of the "flatness" of a distribution, is far from a mere statistical curiosity. Understanding platykurtic distributions is vital for several reasons:
- Accurate Risk Assessment: In finance, platykurtic distributions can indicate lower risk compared to leptokurtic distributions (distributions with high peaks and heavy tails), as extreme values are less frequent.
- Improved Model Building: Recognizing the shape of your data distribution informs the choice of appropriate statistical models. Applying an inappropriate model to platykurtic data can lead to inaccurate inferences.
- Enhanced Data Interpretation: Understanding platykurtosis allows for a more nuanced interpretation of data, leading to more reliable conclusions and better decision-making across various fields.
- Identifying Outliers: While not as prone to outliers as leptokurtic distributions, platykurtic distributions still benefit from outlier analysis to ensure data integrity and reliable statistical results.
Overview: What This Article Covers:
This article will thoroughly explore platykurtic distributions, starting with a clear definition and progressing to real-world examples. We'll compare and contrast platykurtic distributions with other common distributions (leptokurtic and mesokurtic), highlighting their key differences and implications. Finally, we will discuss the practical applications of understanding platykurtosis in various fields.
The Research and Effort Behind the Insights:
This article is the result of extensive research, drawing upon established statistical literature, textbooks, and online resources. The information presented is rigorously vetted to ensure accuracy and clarity, providing readers with a reliable understanding of platykurtic distributions.
Key Takeaways:
- Definition and Core Concepts: A clear explanation of platykurtosis and its mathematical representation.
- Examples of Platykurtic Distributions: Real-world illustrations of data sets exhibiting platykurtosis.
- Comparison with Other Distributions: A detailed comparison of platykurtic, leptokurtic, and mesokurtic distributions.
- Applications and Implications: The practical use of understanding platykurtosis in different fields.
Smooth Transition to the Core Discussion:
Now that we understand the importance of platykurtic distributions, let's delve deeper into their characteristics and applications.
Exploring the Key Aspects of Platykurtic Distributions:
1. Definition and Core Concepts:
Platykurtosis refers to a distribution with low kurtosis. Kurtosis is a measure of the "tailedness" and "peakedness" of a probability distribution. It quantifies the concentration of data around the mean and in the tails of the distribution. A platykurtic distribution is characterized by thin tails and a flatter peak compared to a normal distribution. The kurtosis of a normal distribution is 3. A platykurtic distribution will have a kurtosis less than 3. Sometimes, excess kurtosis is used, which is the kurtosis minus 3. A platykurtic distribution has negative excess kurtosis.
2. Examples of Platykurtic Distributions:
Finding perfectly platykurtic real-world datasets is rare. Most data distributions are approximations. However, certain scenarios can lead to distributions approximating platykurtosis:
- Uniform Distribution: The uniform distribution, where all values within a given range have equal probability, is a classic example of a platykurtic distribution. The probability density function is flat, lacking any significant peaks or tails.
- Data with Limited Extreme Values: A dataset where extreme values are suppressed or rarely occur will often exhibit platykurtosis. This could be due to constraints, truncation, or data censoring. For example, data on student test scores might be platykurtic if the test is designed such that very high or very low scores are uncommon.
- Averaging of data: If you take averages of samples from any distribution, the resulting distribution tends toward normality, and therefore, may be platykurtic, especially if the original distributions were leptokurtic.
3. Comparison with Other Distributions:
To better understand platykurtosis, it's essential to compare it with other types of distributions based on their kurtosis:
- Mesokurtic Distribution: A mesokurtic distribution has a kurtosis of approximately 3. The normal distribution is the quintessential example. Its peak is neither too sharp nor too flat, and its tails are neither too heavy nor too light.
- Leptokurtic Distribution: Leptokurtic distributions have high kurtosis (greater than 3). They are characterized by a sharp peak and heavy tails. This indicates a higher probability of observing extreme values compared to a normal distribution. Examples include the Laplace distribution and the Student's t-distribution with a small number of degrees of freedom.
4. Applications and Implications:
The understanding of platykurtic distributions has implications across various fields:
- Finance: In financial modeling, recognizing platykurtic distributions can lead to more accurate risk assessments. The lower probability of extreme events compared to leptokurtic distributions can affect portfolio optimization strategies and risk management techniques.
- Quality Control: In manufacturing, platykurtic distributions in quality control data might signal consistent product quality with minimal variations. This can inform production processes and improve efficiency.
- Environmental Science: In environmental studies, platykurtic distributions could reflect relatively stable environmental conditions with minimal extreme events. This can be valuable for understanding climate patterns and ecosystem stability.
- Healthcare: The distribution of certain health metrics might be platykurtic. Understanding this can influence the design of clinical trials and public health interventions.
Exploring the Connection Between Data Transformation and Platykurtic Distributions:
Data transformation techniques can significantly impact the kurtosis of a distribution. Transformations like taking logarithms or square roots can reduce the kurtosis of a leptokurtic distribution, potentially making it closer to a platykurtic or mesokurtic shape. This is particularly useful when applying statistical methods that assume normality. Conversely, some transformations might increase kurtosis, moving a distribution away from platykurtosis.
Key Factors to Consider:
- Roles and Real-World Examples: Data transformation is often employed in practice to achieve normality or improve the performance of statistical models. Consider scenarios where transforming data leads to a platykurtic distribution, improving the suitability for analysis.
- Risks and Mitigations: Inappropriate data transformations can lead to misinterpretations. Careful consideration of the data's nature and the goals of the analysis is crucial.
- Impact and Implications: The choice of data transformation has significant implications for the results and conclusions drawn from the analysis. It's vital to document all transformations and their rationale to ensure transparency and reproducibility.
Conclusion: Reinforcing the Connection:
The relationship between data transformation and platykurtic distributions demonstrates the crucial role of data preprocessing in statistical analysis. By carefully selecting and applying transformation techniques, researchers can improve the suitability of their data for various statistical methods and enhance the accuracy and reliability of their findings.
Further Analysis: Examining Data Transformation in Greater Detail:
A deeper examination of various data transformation methods – logarithmic, square root, Box-Cox – reveals their individual effects on kurtosis and their suitability for different types of data. Understanding these techniques empowers researchers to choose the most appropriate approach for achieving a distribution closer to platykurtosis or mesokurtosis as needed.
FAQ Section: Answering Common Questions About Platykurtic Distributions:
-
Q: What is the practical significance of a platykurtic distribution?
- A: A platykurtic distribution indicates less variability and fewer extreme values compared to a normal distribution. This is useful in many fields for risk assessment, quality control, and understanding the stability of processes.
-
Q: How can I determine if my data is platykurtic?
- A: You can calculate the kurtosis of your data using statistical software or programming languages like R or Python. If the kurtosis is less than 3 (or the excess kurtosis is negative), then the data is platykurtic.
-
Q: What statistical tests are suitable for platykurtic data?
- A: While many tests assume normality, some non-parametric tests are less sensitive to the distribution shape and can be used with platykurtic data. Robust statistical methods are also often a good choice.
-
Q: Can I force my data to become platykurtic?
- A: While you can transform your data to reduce kurtosis, artificially forcing it to be platykurtic may not be appropriate or meaningful. Consider whether the transformation aligns with the underlying nature of your data and the research questions.
Practical Tips: Maximizing the Benefits of Understanding Platykurtic Distributions:
- Understand the Data: Begin by carefully examining your data visually and calculating summary statistics, including kurtosis.
- Consider Transformations: Explore data transformations if your data is significantly non-platykurtic and the transformation is justified.
- Choose Appropriate Methods: Select statistical methods that are robust to deviations from normality or use non-parametric alternatives when necessary.
- Interpret Carefully: Always interpret results in the context of your data and the chosen analytical methods.
Final Conclusion: Wrapping Up with Lasting Insights:
Platykurtic distributions, although less frequently discussed than leptokurtic distributions, offer valuable insights into data variability and risk assessment. Understanding platykurtosis empowers researchers and analysts to select appropriate statistical methods, interpret results accurately, and draw more informed conclusions across various fields. By acknowledging the unique characteristics of platykurtic data, one can enhance the reliability and meaningfulness of statistical analyses.
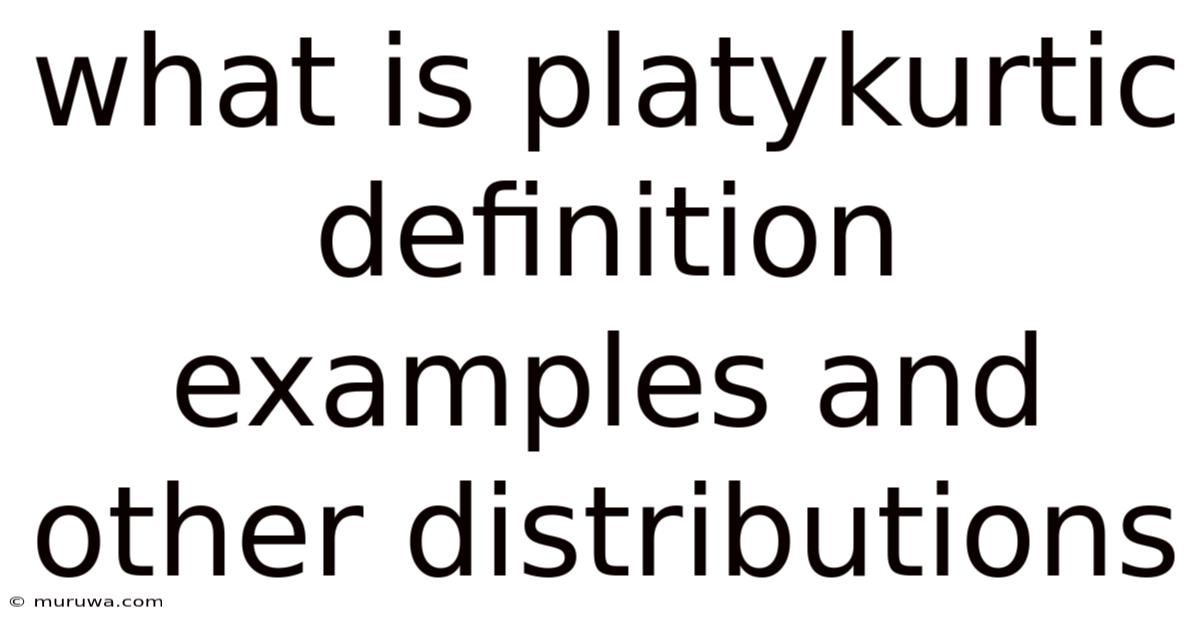
Thank you for visiting our website wich cover about What Is Platykurtic Definition Examples And Other Distributions. We hope the information provided has been useful to you. Feel free to contact us if you have any questions or need further assistance. See you next time and dont miss to bookmark.
Also read the following articles
Article Title | Date |
---|---|
Permissioned Blockchain Definition Examples Vs Permissionless | Mar 10, 2025 |
How To Add Tradelines To Business Credit | Mar 10, 2025 |
Per Share Basis Definition | Mar 10, 2025 |
What Is A Ready Line Of Credit | Mar 10, 2025 |
How To Make A Credit Memo In Quickbooks | Mar 10, 2025 |