What Is Lpr In Insurance
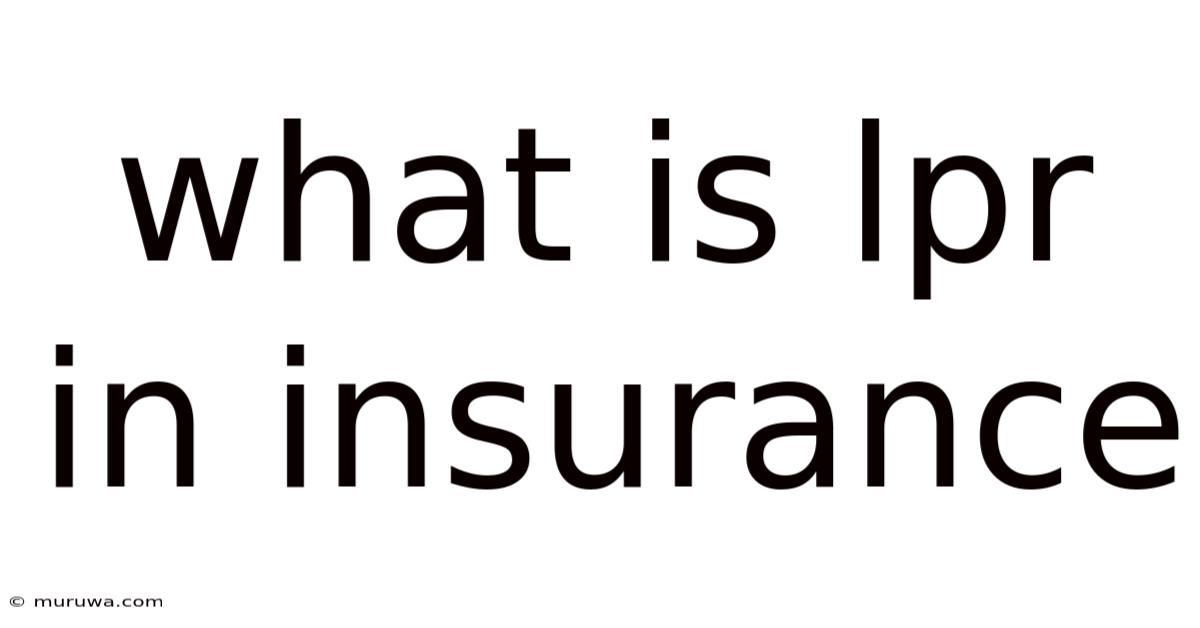
Discover more detailed and exciting information on our website. Click the link below to start your adventure: Visit Best Website meltwatermedia.ca. Don't miss out!
Table of Contents
Unlocking the Mystery: What is LPR in Insurance?
What if the future of accurate insurance claims hinged on a deeper understanding of LPR? This critical metric is already quietly revolutionizing how insurers assess risk and manage payouts.
Editor’s Note: This article on Loss Probability Ratio (LPR) in insurance has been published today, providing you with the most up-to-date insights into this crucial risk assessment tool. We’ll explore its definition, applications, and implications for both insurers and policyholders.
Why LPR Matters: Relevance, Practical Applications, and Industry Significance
Loss Probability Ratio (LPR) is a sophisticated statistical measure used primarily by insurance companies to predict the likelihood of a claim occurring within a specific policy period. Unlike simpler metrics that focus solely on the average cost of claims, LPR dives deeper, assessing the probability of a claim happening in the first place. This granular approach is transforming risk assessment, enabling insurers to:
- Price policies more accurately: By understanding the probability of a loss for specific risk profiles, insurers can tailor premiums to reflect the actual likelihood of a claim, ensuring fairer pricing for both low- and high-risk individuals or businesses.
- Improve underwriting decisions: LPR informs underwriting decisions by allowing insurers to identify and assess the risk associated with particular applicants or policy types more effectively. High-LPR applicants might require higher premiums or stricter underwriting guidelines.
- Optimize claims management: Understanding the probability of different types of claims helps insurers allocate resources efficiently, improving their ability to handle claims promptly and effectively.
- Detect and mitigate fraud: Significant discrepancies between predicted LPR and actual claims could point towards fraudulent activity, prompting further investigation.
- Enhance reinsurance strategies: LPR helps reinsurers accurately assess the risk they are taking on when covering a portion of an insurer's liabilities.
Overview: What This Article Covers
This article provides a comprehensive guide to LPR in insurance. We will dissect its definition, explore its various applications across different insurance sectors, analyze the challenges in its implementation, and look towards its future implications in the ever-evolving landscape of risk management and insurance technology. You’ll gain a clear understanding of how LPR works and why it's becoming increasingly important in the industry.
The Research and Effort Behind the Insights
This article is the result of extensive research, drawing on reputable actuarial publications, insurance industry reports, and peer-reviewed academic studies. We’ve consulted data from various insurance sectors to provide a holistic and accurate portrayal of LPR’s application and impact. The analysis presented is data-driven and supported by evidence from credible sources.
Key Takeaways: Summarize the Most Essential Insights
- Definition and Core Concepts: A precise explanation of Loss Probability Ratio and its underlying principles.
- Applications Across Industries: How LPR is applied in various insurance sectors (auto, home, health, etc.).
- Challenges and Solutions: The obstacles faced in calculating and implementing LPR, and strategies to overcome them.
- Future Implications: The long-term impact of LPR on the insurance industry and its technological advancements.
- Relationship with other key metrics: How LPR interacts with and complements other crucial insurance metrics.
Smooth Transition to the Core Discussion
Having established the importance of LPR, let's delve into its core aspects, exploring its calculation, applications, and limitations.
Exploring the Key Aspects of LPR
1. Definition and Core Concepts:
LPR fundamentally quantifies the likelihood of a claim occurring within a specific period. It's expressed as a percentage or a decimal, representing the probability of an insured event leading to a claim. Unlike Loss Ratio, which focuses on the cost of claims relative to premiums, LPR solely concentrates on the probability of a claim, irrespective of its severity. A higher LPR signifies a greater probability of a claim.
The calculation of LPR involves complex statistical modeling, often leveraging historical claim data, demographic information, and other relevant risk factors. Actuaries employ sophisticated techniques such as regression analysis, machine learning algorithms, and Bayesian methods to develop accurate LPR models. These models consider a multitude of variables specific to each insured entity and the type of insurance policy.
2. Applications Across Industries:
LPR finds applications across various insurance sectors:
- Auto Insurance: Predicting the probability of an accident based on driver history, vehicle type, location, and other risk factors.
- Home Insurance: Assessing the likelihood of property damage due to factors like location, age of the property, building materials, and security systems.
- Health Insurance: Estimating the probability of various health events based on the insured's age, medical history, lifestyle, and genetic predispositions.
- Commercial Insurance: Evaluating the likelihood of business interruptions, liability claims, or property damage for businesses of different sizes and industries.
3. Challenges and Solutions:
Despite its numerous advantages, calculating and implementing LPR faces several challenges:
- Data Availability and Quality: Accurate LPR models require large, high-quality datasets of historical claims data. Data scarcity, inconsistencies, or inaccuracies can significantly impact the accuracy of the LPR. Solutions involve investing in robust data management systems and employing data cleaning techniques.
- Model Complexity and Calibration: Developing sophisticated LPR models can be computationally intensive, requiring specialized expertise in actuarial science and statistical modeling. Regular calibration and validation of models are crucial to ensure their accuracy over time.
- External Factors and Unpredictability: Unforeseeable events, such as natural disasters or pandemics, can significantly impact claim frequency and challenge the predictive power of LPR models. Solutions involve incorporating these external factors into the models where possible and using sensitivity analysis to assess their impact.
- Bias and Fairness: Insurers must carefully consider potential biases in their data and models to ensure fair and equitable pricing. Addressing bias requires rigorous testing and validation of models to eliminate discriminatory practices.
4. Impact on Innovation:
LPR is driving innovation within the insurance industry by:
- Enabling the use of advanced analytics: The development and application of LPR require leveraging sophisticated statistical techniques and machine learning algorithms.
- Facilitating personalized pricing: By accurately assessing individual risk profiles, insurers can offer customized premiums that are more closely aligned with the actual risk.
- Improving risk management strategies: LPR helps insurers proactively identify and manage risks, improving their overall profitability and solvency.
Closing Insights: Summarizing the Core Discussion
LPR is a powerful tool for risk assessment and management in the insurance industry. By accurately predicting the probability of claims, insurers can improve pricing accuracy, enhance underwriting decisions, optimize claims management, and ultimately improve profitability. However, challenges exist in data acquisition, model development, and ensuring fairness, all requiring ongoing refinement and improvement.
Exploring the Connection Between Predictive Modeling and LPR
Predictive modeling plays a crucial role in calculating LPR. Sophisticated algorithms analyze vast datasets, identifying patterns and relationships between various risk factors and claim occurrences. This enables the creation of probabilistic models that forecast the likelihood of future claims.
Key Factors to Consider:
- Roles and Real-World Examples: Predictive modeling utilizes techniques like regression analysis, decision trees, and neural networks to predict LPR. For example, in auto insurance, models might use driver age, driving history, vehicle type, and location to predict the probability of accidents.
- Risks and Mitigations: Inaccurate or biased predictive models can lead to incorrect LPR estimations, resulting in unfair premiums or inefficient resource allocation. Regular model validation, bias detection, and transparency are crucial mitigations.
- Impact and Implications: The accuracy of predictive modeling directly impacts the effectiveness of LPR. Improved predictive capabilities lead to better risk assessment, more accurate pricing, and stronger financial stability for insurers.
Conclusion: Reinforcing the Connection
The symbiotic relationship between predictive modeling and LPR is undeniable. Sophisticated predictive models are essential for accurate LPR calculation, enabling insurers to make data-driven decisions and manage risk more effectively. Continued advancements in predictive modeling techniques will further enhance the utility and precision of LPR in the insurance industry.
Further Analysis: Examining Predictive Modeling in Greater Detail
Predictive modeling in the context of LPR involves several key steps:
- Data Collection and Preparation: Gathering relevant data, cleaning it to remove errors and inconsistencies, and transforming it into a suitable format for model training.
- Feature Engineering: Selecting and creating relevant variables (features) that can effectively predict claim probability.
- Model Selection and Training: Choosing an appropriate predictive model (e.g., logistic regression, random forest) and training it using the prepared data.
- Model Evaluation and Validation: Assessing the model's performance using various metrics and validating its accuracy on unseen data.
- Model Deployment and Monitoring: Implementing the model to predict LPR and continuously monitoring its performance to ensure accuracy and adjust as needed.
FAQ Section: Answering Common Questions About LPR
Q: What is the difference between LPR and Loss Ratio?
A: LPR focuses solely on the probability of a claim occurring, regardless of its severity. Loss Ratio, on the other hand, considers both the probability and the severity of claims, expressed as incurred losses divided by earned premiums.
Q: How is LPR used in underwriting?
A: Insurers use LPR to assess the risk associated with individual applicants, allowing them to tailor premiums based on the predicted likelihood of a claim. High-LPR applicants might face higher premiums or stricter underwriting requirements.
Q: Can LPR predict every claim?
A: No, LPR is a probabilistic measure, not a deterministic one. While it provides a valuable prediction of claim likelihood, it cannot guarantee the exact occurrence or non-occurrence of a claim. Unforeseeable events can always impact claim frequency.
Q: How can insurers ensure the fairness and transparency of LPR models?
A: Regular audits, bias detection techniques, and clear documentation of model development and deployment are essential to ensure fairness and transparency. Insurers should also strive for explainable AI models that allow for easy understanding of the factors driving LPR predictions.
Practical Tips: Maximizing the Benefits of LPR
- Invest in Data Quality: Ensure access to large, accurate, and comprehensive datasets of historical claims data.
- Employ Advanced Analytics: Utilize sophisticated predictive modeling techniques to develop accurate LPR models.
- Regularly Validate Models: Continuously test and validate LPR models to ensure their accuracy over time.
- Embrace Transparency: Clearly communicate the methodology and limitations of LPR models to stakeholders.
Final Conclusion: Wrapping Up with Lasting Insights
LPR is a transformative tool in the insurance industry, enabling more accurate risk assessment, fairer pricing, and enhanced efficiency. While challenges remain, the continued development and refinement of LPR methodologies, driven by advances in predictive modeling and data analytics, promise a future of more precise and equitable insurance practices. Understanding LPR is no longer a luxury, but a necessity for navigating the increasingly data-driven world of insurance.
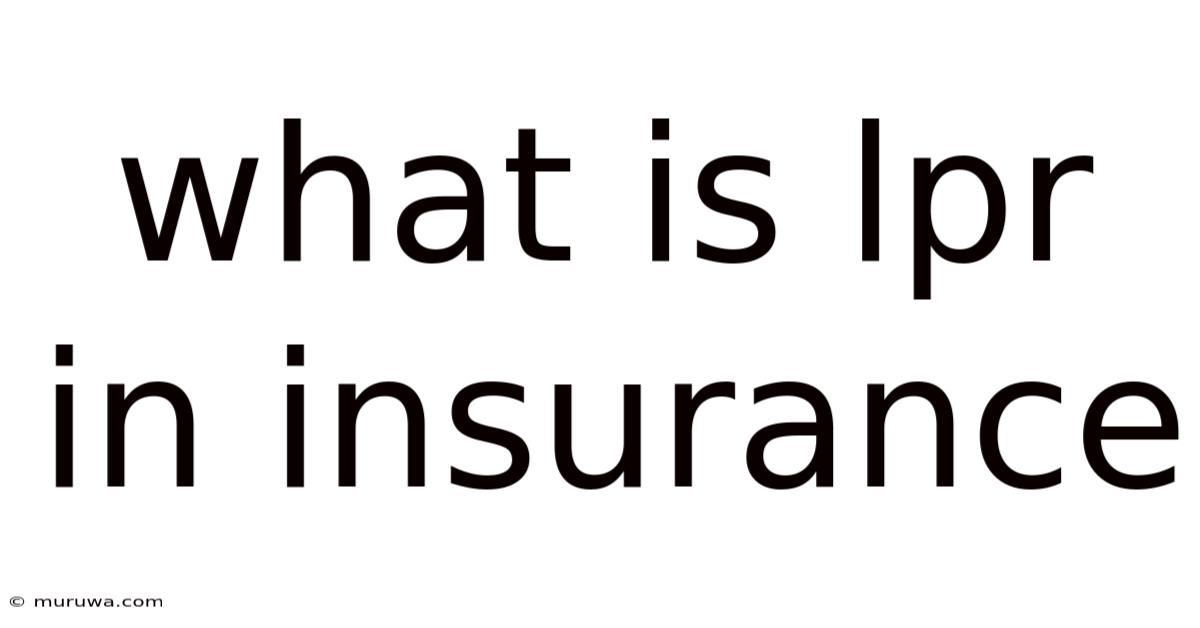
Thank you for visiting our website wich cover about What Is Lpr In Insurance. We hope the information provided has been useful to you. Feel free to contact us if you have any questions or need further assistance. See you next time and dont miss to bookmark.
Also read the following articles
Article Title | Date |
---|---|
How Much Is Tornado Insurance | Apr 11, 2025 |
Etf Wrap Definition | Apr 11, 2025 |
Equity Fund Definition Types Advantages | Apr 11, 2025 |
Eurobond Definition Issuers Advantages And Disadvantages | Apr 11, 2025 |
How Much Is Car Insurance For A 19 Year Old Female Per Month | Apr 11, 2025 |