Posterior Probability Definition Formula For Calculation
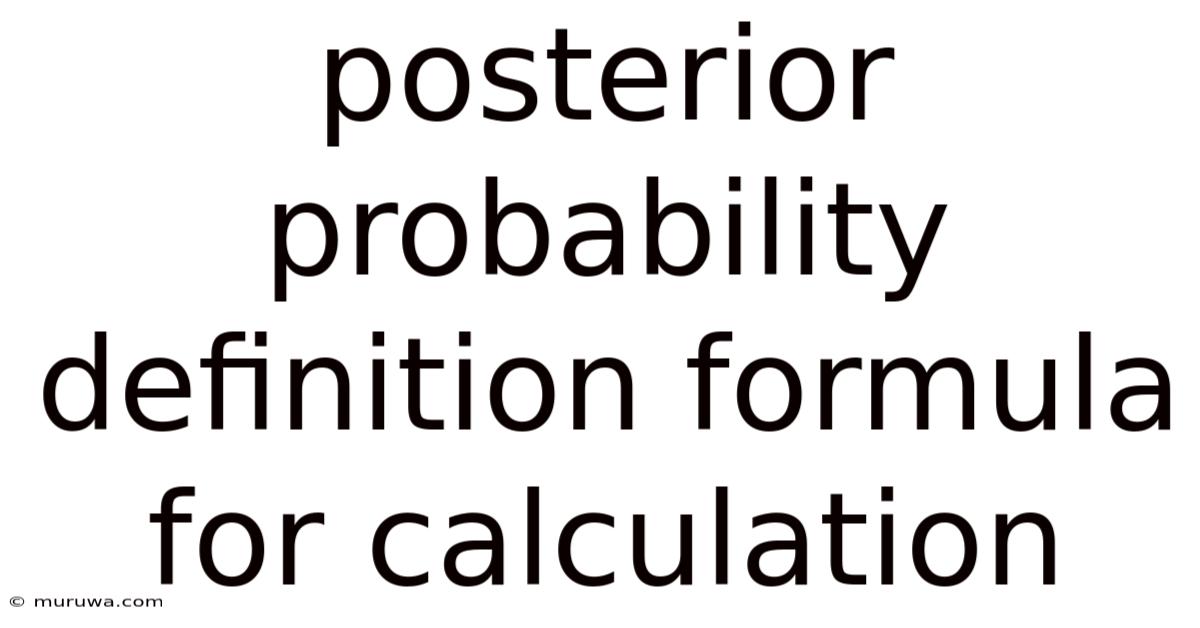
Discover more detailed and exciting information on our website. Click the link below to start your adventure: Visit Best Website meltwatermedia.ca. Don't miss out!
Table of Contents
Decoding Posterior Probability: Definition, Formula, and Calculation
What if unlocking the true power of Bayesian inference hinges on a deep understanding of posterior probability? This fundamental concept underpins many crucial advancements in machine learning, statistics, and decision-making, offering profound insights into uncertain events.
Editor’s Note: This article on posterior probability provides a comprehensive guide to its definition, calculation, and practical applications. We'll explore the underlying mathematical principles and demonstrate its use through illustrative examples. This updated resource aims to clarify this often-misunderstood yet vital statistical concept.
Why Posterior Probability Matters:
Posterior probability is the cornerstone of Bayesian inference, a powerful statistical framework used to update beliefs based on new evidence. Unlike frequentist statistics, which focuses on the frequency of events, Bayesian inference incorporates prior knowledge and updates it with observed data to arrive at a refined understanding of probabilities. This allows for a more nuanced and adaptive approach to decision-making in situations involving uncertainty, making it crucial across fields such as machine learning (especially in classification and spam filtering), medical diagnosis, risk assessment, and weather forecasting. The ability to quantify and update uncertainty is highly valuable in these domains.
Overview: What This Article Covers:
This article will delve into the core aspects of posterior probability, providing a clear definition, detailing the formula for its calculation, and illustrating its application through examples. We will explore the relationship between prior probability, likelihood, and evidence, showing how they combine to determine the posterior probability. Furthermore, we’ll examine practical considerations, potential challenges, and applications across different fields.
The Research and Effort Behind the Insights:
This article is the result of extensive research, drawing upon established statistical textbooks, peer-reviewed journal articles, and reputable online resources. Every formula and explanation is grounded in rigorous mathematical principles, ensuring accuracy and providing a reliable guide for readers seeking a comprehensive understanding of posterior probability.
Key Takeaways:
- Definition and Core Concepts: A precise definition of posterior probability and its role in Bayesian inference.
- Formula and Calculation: A step-by-step breakdown of the formula and its components, accompanied by illustrative examples.
- Applications Across Industries: Practical applications in various fields, showcasing the versatility of posterior probability.
- Challenges and Solutions: Potential difficulties encountered in calculating posterior probability and strategies to address them.
- Advanced Concepts: Brief exploration of more complex scenarios and extensions of the fundamental concepts.
Smooth Transition to the Core Discussion:
Having established the significance of posterior probability, let's now delve into the specifics of its definition, formula, and application.
Exploring the Key Aspects of Posterior Probability:
1. Definition and Core Concepts:
Posterior probability, denoted as P(A|B), represents the probability of an event A occurring given that another event B has already occurred. In the Bayesian context, A often represents a hypothesis or a parameter, while B represents observed data. The posterior probability reflects the updated belief about A after considering the evidence B. It's a conditional probability, meaning it depends on the occurrence of event B.
2. Bayes' Theorem: The Foundation of Posterior Probability Calculation:
Bayes' Theorem provides the mathematical framework for calculating the posterior probability. The formula is:
P(A|B) = [P(B|A) * P(A)] / P(B)
Where:
- P(A|B) is the posterior probability of A given B.
- P(B|A) is the likelihood – the probability of observing B given that A is true.
- P(A) is the prior probability – the initial belief about the probability of A before observing any data.
- P(B) is the marginal likelihood or evidence – the probability of observing B, regardless of whether A is true or false. This acts as a normalizing constant, ensuring the posterior probability sums to 1.
3. Calculating P(B): The Marginal Likelihood:
Calculating P(B), the marginal likelihood, can be more complex. It's often calculated using the law of total probability:
P(B) = Σ [P(B|A<sub>i</sub>) * P(A<sub>i</sub>)]
Where the summation is over all possible values of A (A<sub>i</sub>). In simpler cases with only two possible outcomes for A (e.g., A and not A), this simplifies to:
P(B) = P(B|A) * P(A) + P(B|¬A) * P(¬A)
4. Applications Across Industries:
-
Medical Diagnosis: Assessing the probability of a disease given certain symptoms. P(Disease|Symptoms) is the posterior probability calculated using Bayes' theorem, considering prior knowledge about disease prevalence (prior probability) and the likelihood of observing specific symptoms given the disease.
-
Spam Filtering: Determining whether an email is spam or not spam based on the words it contains. P(Spam|Words) is the posterior probability, incorporating the prior probability of an email being spam and the likelihood of those specific words appearing in spam emails.
-
Machine Learning: In Naive Bayes classifiers, posterior probabilities are used to predict the class of a data point based on its features. For example, classifying images as cats or dogs based on image features.
-
Financial Modeling: Assessing credit risk, predicting market trends, and evaluating investment opportunities often involve Bayesian methods and the calculation of posterior probabilities.
-
Weather Forecasting: Updating the probability of rain given new weather data (radar, temperature, etc.). The posterior probability incorporates prior weather patterns and the likelihood of observing specific weather phenomena given different rain scenarios.
5. Challenges and Solutions:
-
Prior Probability Selection: Choosing an appropriate prior probability can be subjective. Different priors can lead to different posterior probabilities. Techniques like using uninformative priors (which don't strongly influence the posterior) or incorporating expert knowledge can mitigate this.
-
Computational Complexity: For complex models with many variables, calculating the marginal likelihood P(B) can be computationally intensive. Approximation methods like Markov Chain Monte Carlo (MCMC) are often used to overcome this.
-
Data Sparsity: If the dataset is small, the likelihood estimates might be unreliable, leading to inaccurate posterior probabilities. Techniques like regularization or smoothing can help address this issue.
6. Advanced Concepts (Brief Overview):
-
Bayesian Networks: Represent probabilistic relationships between multiple variables using directed acyclic graphs. These networks enable the efficient calculation of posterior probabilities for complex systems.
-
Hierarchical Bayesian Models: Incorporate multiple layers of prior distributions, allowing for more flexible and nuanced modeling of uncertainty.
Exploring the Connection Between Prior Probability and Posterior Probability:
The relationship between prior probability and posterior probability is fundamental to Bayesian inference. The prior probability represents the initial belief about the likelihood of an event before any data is observed. The posterior probability is the updated belief after considering the evidence. The strength of the prior influences how much the posterior shifts from the prior. A strong prior will result in a posterior that is relatively close to the prior, even with significant evidence. A weak prior will allow the data to strongly influence the posterior, leading to a larger shift.
Key Factors to Consider:
-
Roles and Real-World Examples: In medical diagnosis, the prior probability might reflect the prevalence of a disease in a particular population. After observing symptoms, the posterior probability gives a refined estimate of the likelihood of the disease.
-
Risks and Mitigations: Over-reliance on a strong prior can lead to bias if the prior is inaccurate. Using objective and well-justified priors and incorporating sensitivity analysis (testing different priors) can help mitigate this risk.
-
Impact and Implications: The choice of prior significantly impacts the posterior probability and subsequent decisions. A poorly chosen prior can lead to inaccurate conclusions and suboptimal actions.
Conclusion: Reinforcing the Connection:
The interplay between prior and posterior probability highlights the iterative and adaptive nature of Bayesian inference. By carefully selecting priors and incorporating evidence effectively, the process leads to increasingly refined estimations of uncertainty and improved decision-making.
Further Analysis: Examining Prior Probability in Greater Detail:
Understanding the selection of appropriate prior probabilities is crucial for accurate Bayesian inference. Different methods exist, including:
-
Informative Priors: Reflect prior knowledge or expert opinion. These can be based on past data, theoretical models, or subjective judgment.
-
Uninformative Priors: Express a lack of strong prior belief. These priors aim to let the data speak for itself, minimizing the influence of the prior on the posterior. Examples include uniform priors and Jeffreys priors.
-
Conjugate Priors: Simplifies calculations by ensuring the posterior probability has the same form as the prior. This significantly reduces computational complexity.
FAQ Section: Answering Common Questions About Posterior Probability:
-
Q: What is the difference between prior and posterior probability?
- A: Prior probability is the initial belief before data is observed, while posterior probability is the updated belief after incorporating evidence.
-
Q: How do I choose a prior probability?
- A: The choice depends on the context. Consider using informative priors if substantial prior knowledge exists or uninformative priors if prior knowledge is limited.
-
Q: What if I don't have any prior knowledge?
- A: In such cases, use an uninformative prior that minimizes the prior's influence on the posterior.
-
Q: What are the limitations of using posterior probability?
- A: The accuracy depends on the quality of the prior and the data. Computational complexity can be an issue for large datasets.
Practical Tips: Maximizing the Benefits of Posterior Probability:
- Clearly define the problem: Identify the events of interest and the available data.
- Choose appropriate priors: Select priors that reflect prior knowledge or the lack thereof.
- Calculate the likelihood: Determine the probability of observing the data given different hypotheses.
- Calculate the marginal likelihood: Use the law of total probability to compute P(B).
- Apply Bayes' Theorem: Compute the posterior probability using the formula.
- Interpret the results: Analyze the posterior probability to draw conclusions and make informed decisions.
Final Conclusion: Wrapping Up with Lasting Insights:
Posterior probability is a fundamental concept in Bayesian statistics, offering a powerful framework for updating beliefs in the face of new evidence. By understanding its definition, calculating it using Bayes' Theorem, and considering the nuances of prior probability selection, one can harness its power across numerous fields to make more informed and reliable decisions in the presence of uncertainty. Its capacity for adapting to new information makes it an invaluable tool in navigating the complexities of an uncertain world.
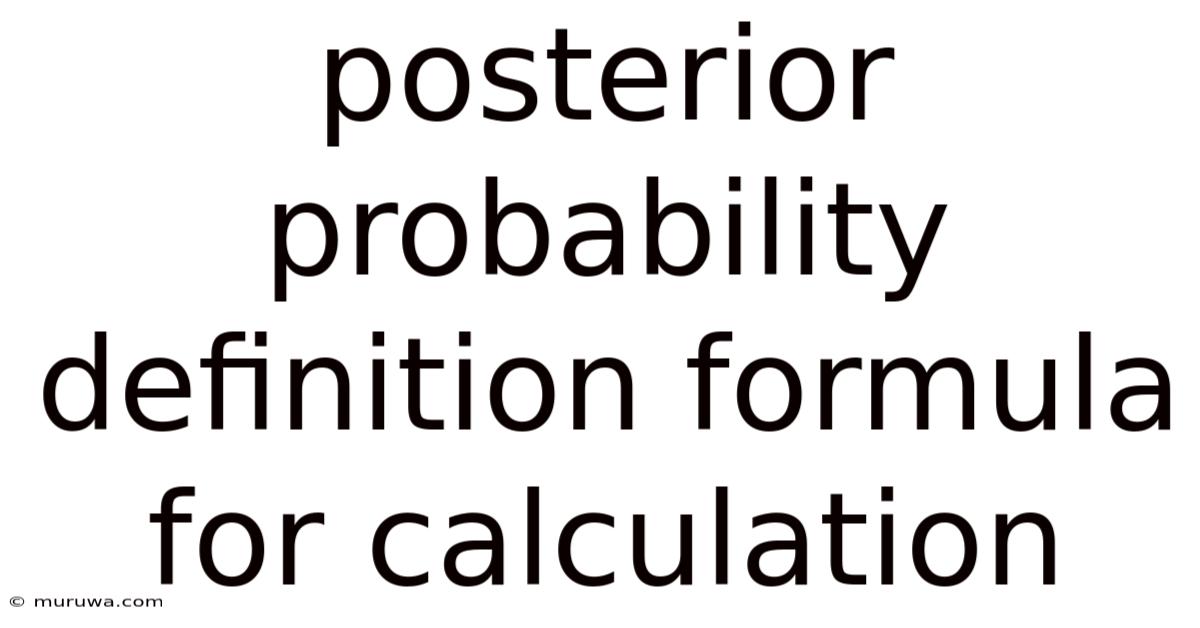
Thank you for visiting our website wich cover about Posterior Probability Definition Formula For Calculation. We hope the information provided has been useful to you. Feel free to contact us if you have any questions or need further assistance. See you next time and dont miss to bookmark.
Also read the following articles
Article Title | Date |
---|---|
How To Get An Audible Credit | Mar 10, 2025 |
Permanent Income Hypothesis Definition How It Works And Impact | Mar 10, 2025 |
What Is Non Traditional Credit | Mar 10, 2025 |
What Places Take Paypal Credit | Mar 10, 2025 |
Where Do I Find My Member Number For Credit Union | Mar 10, 2025 |