Positive Correlation Definition Measurement Examples
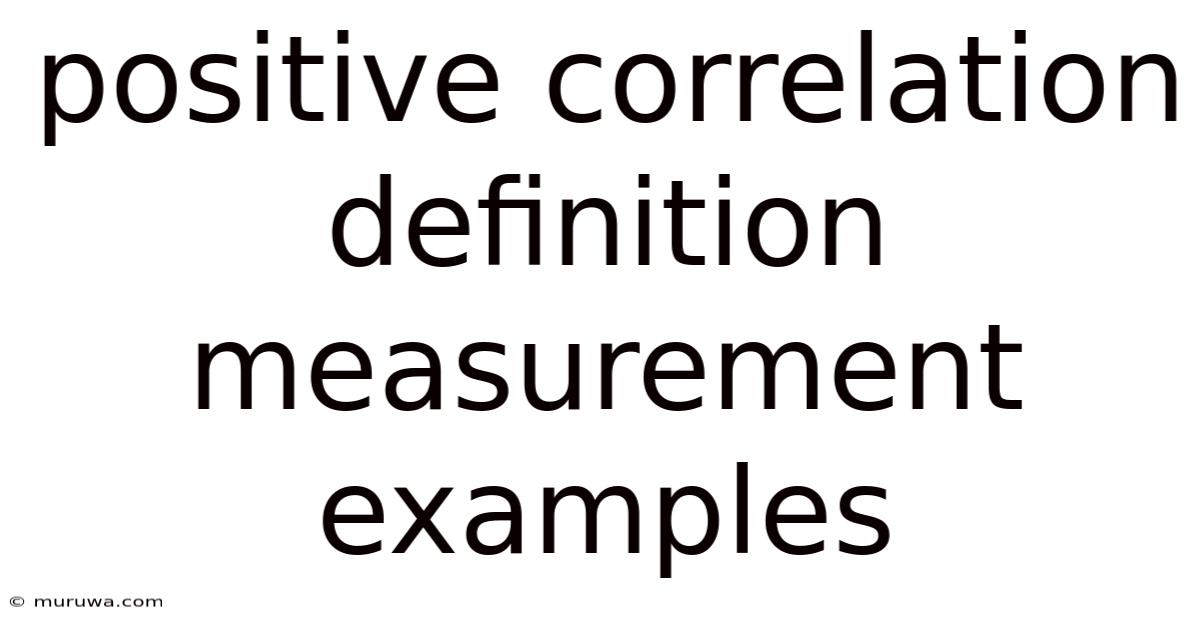
Discover more detailed and exciting information on our website. Click the link below to start your adventure: Visit Best Website meltwatermedia.ca. Don't miss out!
Table of Contents
Unlocking the Secrets of Positive Correlation: Definition, Measurement, and Real-World Examples
What if understanding positive correlation unlocks the key to predicting future trends and optimizing outcomes? This fundamental statistical concept is a powerful tool for understanding relationships within data and making informed decisions across various fields.
Editor’s Note: This article on positive correlation provides a comprehensive exploration of its definition, measurement, and practical applications across diverse fields. Updated with the latest insights, this resource offers readers a clear and actionable understanding of this crucial statistical relationship.
Why Positive Correlation Matters:
Positive correlation describes a statistical relationship where two or more variables tend to move in the same direction. As one variable increases, the other also increases, and vice-versa. Understanding this relationship is critical for numerous reasons:
- Predictive Power: Identifying a positive correlation allows for predictions about one variable based on the value of another. This is invaluable in forecasting, planning, and risk management.
- Decision-Making: Knowing that variables are positively correlated helps in making informed decisions. For example, a positive correlation between advertising spend and sales revenue may inform marketing strategies.
- Understanding Relationships: Positive correlations reveal underlying causal relationships (though correlation does not equal causation) or shared influences affecting multiple variables. This insight aids in developing better theories and models.
- Improvement and Optimization: In various fields, identifying positive correlations enables optimizing systems and processes. For instance, a positive correlation between employee training and productivity suggests focusing on employee development.
Overview: What This Article Covers
This article will provide a detailed explanation of positive correlation, covering its definition, various methods of measurement (including Pearson's r, Spearman's rho, and Kendall's tau), and a range of real-world examples across diverse fields. We will also explore the limitations and potential pitfalls of interpreting positive correlations, emphasizing the crucial distinction between correlation and causation.
The Research and Effort Behind the Insights
The information presented in this article is based on extensive research from reputable statistical sources, academic journals, and industry reports. We've meticulously reviewed numerous studies and real-world applications to ensure accuracy and provide a comprehensive overview of positive correlation. Our approach uses clear, concise language, avoiding overly technical jargon, ensuring accessibility to a broad audience.
Key Takeaways:
- Definition of Positive Correlation: A clear understanding of what constitutes a positive correlation and its implications.
- Measurement Techniques: An exploration of various statistical methods used to quantify the strength and direction of positive correlations.
- Real-World Applications: Diverse examples showcasing the practical uses of positive correlation across different domains.
- Limitations and Cautions: A discussion of the pitfalls of interpreting correlations, particularly the crucial difference between correlation and causation.
Smooth Transition to the Core Discussion:
Having established the significance of positive correlation, let's delve into its core aspects, starting with a precise definition and moving into its measurement and varied applications.
Exploring the Key Aspects of Positive Correlation
1. Definition and Core Concepts:
A positive correlation exists when an increase in one variable is associated with an increase in another variable. The strength of this relationship can range from weak to strong. A perfect positive correlation (+1) indicates that a change in one variable perfectly predicts a proportional change in the other. However, in reality, perfect correlations are rare. Most positive correlations fall between 0 and +1, with values closer to +1 indicating stronger relationships.
2. Applications Across Industries:
Positive correlation analysis finds applications across numerous sectors:
- Economics and Finance: Analyzing the correlation between stock prices, interest rates, and economic indicators helps in investment decisions and risk assessment. A positive correlation between inflation and interest rates, for example, might lead investors to adjust their portfolios accordingly.
- Healthcare: Researchers use positive correlation analysis to identify risk factors for diseases. A positive correlation between smoking and lung cancer, for example, informs public health campaigns and policy decisions.
- Education: Studies might reveal a positive correlation between class attendance and academic performance, informing teaching strategies and student support programs.
- Marketing: Analyzing the correlation between advertising expenditure and sales revenue guides marketing budgets and campaign effectiveness.
- Environmental Science: Positive correlations may exist between pollution levels and respiratory illnesses, helping in environmental policy formulation.
3. Challenges and Solutions:
While positive correlation analysis is valuable, it presents some challenges:
- Causation vs. Correlation: The most significant challenge is the distinction between correlation and causation. A positive correlation only indicates an association; it doesn't prove that one variable causes the other. A confounding variable might be influencing both.
- Data Quality: Accurate and reliable data is crucial for meaningful correlation analysis. Inaccurate or incomplete data can lead to misleading results.
- Non-linear Relationships: Correlation analysis primarily focuses on linear relationships. Non-linear relationships might be missed using standard correlation measures.
4. Impact on Innovation:
Understanding positive correlations facilitates innovation by:
- Targeted Interventions: Identifying positive correlations between variables allows for the development of targeted interventions to improve outcomes. For instance, if there's a positive correlation between exercise and mental well-being, targeted exercise programs can enhance mental health.
- Improved Predictions: Accurate prediction of future trends based on positively correlated variables supports proactive decision-making and resource allocation.
- Enhanced Efficiency: Optimizing systems and processes based on positive correlations leads to increased efficiency and reduced waste.
Closing Insights: Summarizing the Core Discussion
Positive correlation is a powerful statistical tool that helps us understand the relationships between variables. However, it's crucial to remember that correlation does not imply causation. Careful consideration of data quality, potential confounding variables, and the limitations of correlation analysis are essential for drawing valid conclusions and making informed decisions.
Exploring the Connection Between Scatter Plots and Positive Correlation
Scatter plots are invaluable visual tools for representing and understanding positive correlations. A scatter plot displays data points representing pairs of values for two variables. In a positive correlation, the points tend to cluster along a line sloping upwards from left to right. The steeper the slope, generally, the stronger the positive correlation.
Key Factors to Consider:
- Roles and Real-World Examples: Scatter plots allow for a quick visual assessment of the strength and direction of a correlation. For example, a scatter plot showing the relationship between hours studied and exam scores would likely exhibit a positive correlation, with points clustering along an upward-sloping line.
- Risks and Mitigations: Over-interpreting a scatter plot can be misleading. Outliers, clusters of points, and non-linear relationships can obscure the true nature of the correlation. Careful examination and potentially using other statistical measures are needed.
- Impact and Implications: Scatter plots provide an intuitive understanding of the data, making complex statistical concepts easier to grasp. They are frequently used in presentations and reports to communicate findings clearly.
Conclusion: Reinforcing the Connection
Scatter plots serve as essential visual aids in interpreting positive correlations. While they offer a readily understandable representation, it's crucial to complement them with appropriate statistical calculations to quantify the strength of the relationship and to avoid misinterpretations.
Further Analysis: Examining Pearson's r in Greater Detail
Pearson's r is the most commonly used measure of linear correlation. It quantifies the strength and direction of the linear relationship between two variables. It ranges from -1 (perfect negative correlation) to +1 (perfect positive correlation), with 0 indicating no linear correlation. Pearson's r is sensitive to outliers and assumes a linear relationship between the variables.
Methods for Measuring Positive Correlation:
Beyond Pearson's r, other correlation coefficients offer different strengths and are suitable for specific data types:
-
Spearman's rho: This non-parametric correlation coefficient measures the monotonic relationship between two variables. It is less sensitive to outliers than Pearson's r and can be used with ordinal data. A positive Spearman's rho indicates a positive monotonic relationship, where an increase in one variable is associated with an increase in the other, although not necessarily linearly.
-
Kendall's tau: Another non-parametric correlation coefficient, Kendall's tau measures the ordinal association between two variables. It's less sensitive to outliers than Pearson's r and is suitable for data with many ties (repeated values). A positive Kendall's tau indicates a positive monotonic relationship.
The choice of correlation coefficient depends on the data type, the nature of the relationship (linear or monotonic), and the presence of outliers.
FAQ Section: Answering Common Questions About Positive Correlation
Q: What is the difference between correlation and causation?
A: Correlation indicates an association between two variables, but it does not imply that one variable causes the change in the other. Causation requires demonstrating a cause-and-effect relationship. A confounding variable could be influencing both variables, creating a spurious correlation.
Q: How can I calculate Pearson's r?
A: Pearson's r is calculated using a specific formula involving the covariance of the two variables and their standard deviations. Statistical software packages readily calculate Pearson's r.
Q: What are some limitations of correlation analysis?
A: Correlation analysis primarily focuses on linear relationships. It might miss non-linear relationships between variables. It’s also sensitive to outliers, which can distort the results. Finally, correlation does not equal causation.
Practical Tips: Maximizing the Benefits of Positive Correlation Analysis
- Clearly Define Variables: Ensure clear definitions of the variables under investigation to avoid ambiguity.
- Assess Data Quality: Check for errors, missing values, and outliers in the data before conducting the analysis.
- Choose Appropriate Method: Select the appropriate correlation coefficient based on the data type and the nature of the relationship between variables.
- Consider Confounding Variables: Identify and account for potential confounding variables that might influence the relationship between the variables of interest.
- Interpret Results Cautiously: Remember that correlation does not imply causation. Avoid overinterpreting the results and focus on establishing plausible causal relationships through further investigation.
Final Conclusion: Wrapping Up with Lasting Insights
Understanding positive correlation is crucial for making informed decisions in various fields. While correlation analysis provides valuable insights into the relationships between variables, it's essential to interpret the results carefully, considering the limitations and potential pitfalls. By combining correlation analysis with other analytical methods and careful consideration of context, researchers and decision-makers can harness the power of positive correlations to unlock valuable knowledge and optimize outcomes. The ability to identify and interpret positive correlations remains a cornerstone of data-driven decision-making and contributes significantly to advancements across disciplines.
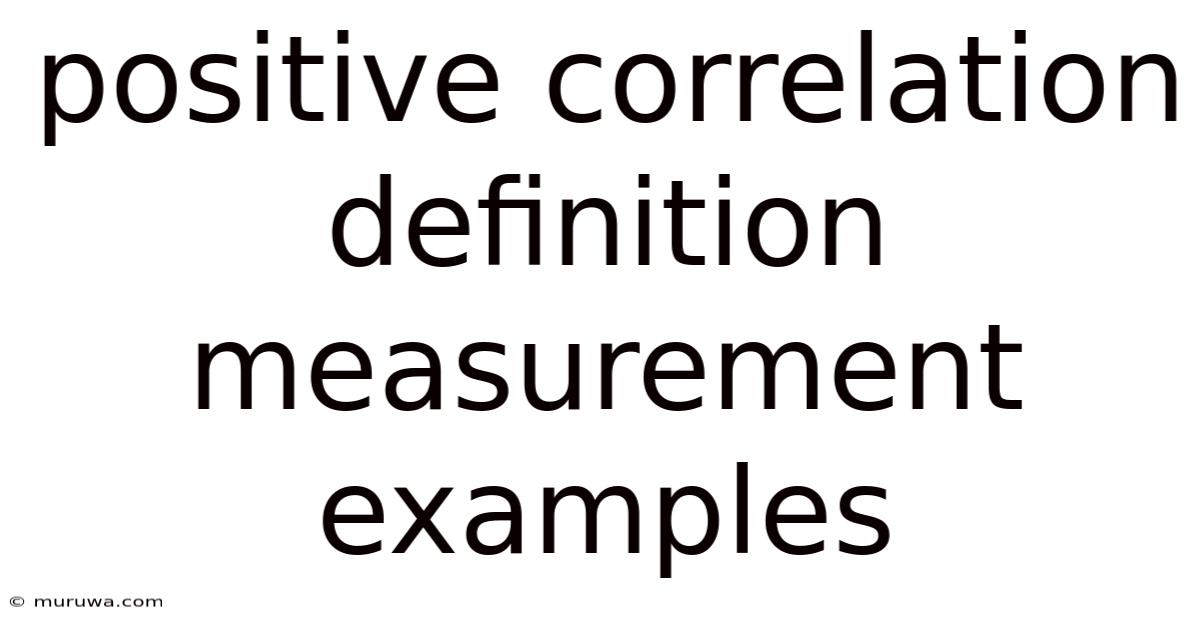
Thank you for visiting our website wich cover about Positive Correlation Definition Measurement Examples. We hope the information provided has been useful to you. Feel free to contact us if you have any questions or need further assistance. See you next time and dont miss to bookmark.
Also read the following articles
Article Title | Date |
---|---|
What Is Path Dependency Definition Effects And Example | Mar 10, 2025 |
How To Calculate Child Tax Credit In 2015 | Mar 10, 2025 |
Post Trade Processing Definition How It Works And Examples | Mar 10, 2025 |
Point And Figure Pf Chart Definition And How To Use In Trade | Mar 10, 2025 |
What Credit Bureau Does Honda Finance Use | Mar 10, 2025 |