Polynomial Trending Definition
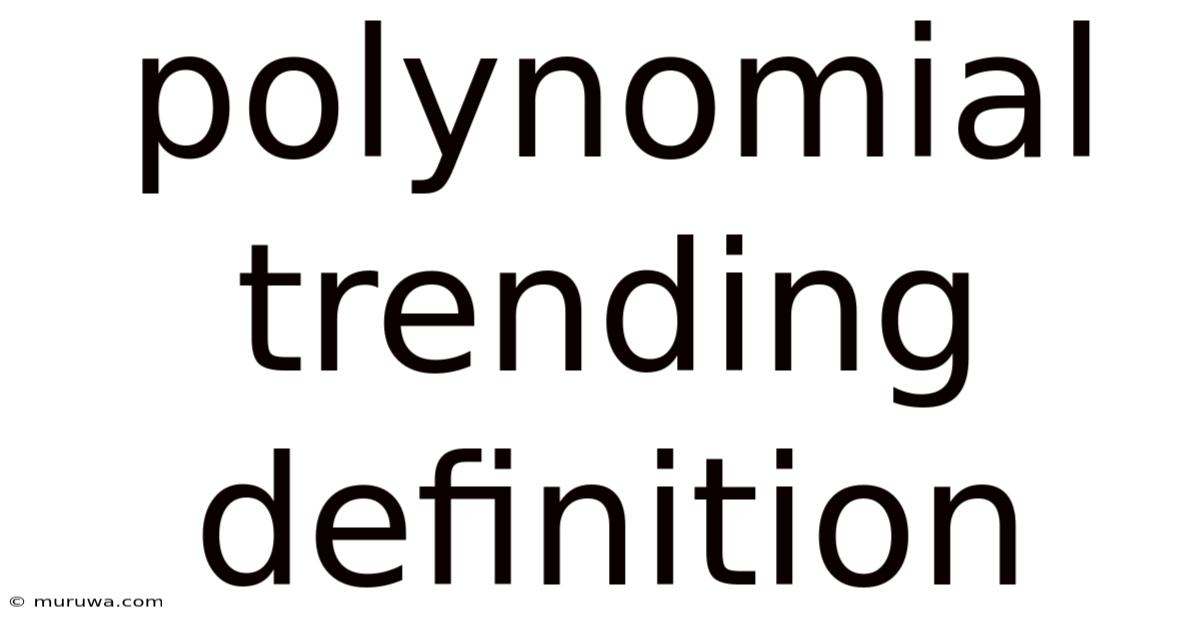
Discover more detailed and exciting information on our website. Click the link below to start your adventure: Visit Best Website meltwatermedia.ca. Don't miss out!
Table of Contents
Decoding Polynomial Trending: A Deep Dive into Definition, Applications, and Significance
What if understanding polynomial trending unlocks the secrets to more accurate forecasting and deeper insights into complex data sets? This powerful analytical technique is rapidly gaining traction across diverse fields, offering a robust approach to modeling dynamic systems.
Editor’s Note: This article on polynomial trending provides a comprehensive overview of this crucial statistical concept, exploring its definition, applications, and implications for various industries. Updated insights and real-world examples are included to ensure readers gain a complete understanding.
Why Polynomial Trending Matters:
Polynomial trending refers to the application of polynomial functions to model trends in data over time. Unlike simpler linear models that assume a constant rate of change, polynomial trends capture more complex patterns, accommodating accelerating or decelerating growth. This adaptability makes it invaluable for analyzing datasets displaying non-linear relationships, a common occurrence in many real-world scenarios. Its relevance spans from financial forecasting and climate modeling to biological growth analysis and engineering design. Understanding polynomial trending empowers businesses and researchers to make more informed decisions, predict future outcomes with greater accuracy, and gain deeper insights into underlying processes.
Overview: What This Article Covers:
This article will provide a thorough exploration of polynomial trending, starting with its fundamental definition and progressing through its practical applications, inherent limitations, and considerations for effective implementation. We will examine different polynomial degrees, their suitability for various data patterns, and the challenges involved in model selection and interpretation. Real-world examples across diverse fields will illustrate the power and versatility of this analytical technique.
The Research and Effort Behind the Insights:
This article draws upon extensive research encompassing statistical literature, academic papers, and industry reports. It incorporates examples from various fields, highlighting the practical applications and implications of polynomial trending. The analysis focuses on providing a clear, concise, and accurate understanding of the subject, emphasizing practical implications and actionable insights.
Key Takeaways:
- Definition and Core Concepts: A detailed explanation of polynomial functions and their application to trend analysis.
- Practical Applications: Examples of polynomial trending across various industries, demonstrating its versatility.
- Challenges and Limitations: Addressing the complexities and potential pitfalls associated with polynomial trend modeling.
- Model Selection and Interpretation: Guidance on choosing the appropriate polynomial degree and interpreting the results accurately.
- Advanced Techniques: Exploring extensions and refinements of basic polynomial trending, such as weighted least squares and spline interpolation.
Smooth Transition to the Core Discussion:
Having established the importance of polynomial trending, let’s delve into the core aspects of this analytical technique, beginning with a precise definition and exploration of its mathematical underpinnings.
Exploring the Key Aspects of Polynomial Trending:
1. Definition and Core Concepts:
A polynomial trend is a mathematical representation of a time series using a polynomial function. A polynomial function of degree n takes the general form:
y = a₀ + a₁x + a₂x² + a₃x³ + ... + aₙxⁿ
where:
- y represents the dependent variable (the value being modeled).
- x represents the independent variable (usually time).
- a₀, a₁, a₂, ..., aₙ are the coefficients that determine the shape of the polynomial curve. These coefficients are estimated using statistical methods, often least squares regression.
The degree of the polynomial (n) determines the complexity of the curve. A polynomial of degree 1 is a straight line (linear trend), degree 2 is a parabola (quadratic trend), degree 3 is a cubic curve, and so on. Higher-degree polynomials can model increasingly complex trends but also risk overfitting the data, leading to inaccurate predictions.
2. Applications Across Industries:
Polynomial trending finds applications in a surprisingly wide range of fields:
- Finance: Modeling stock prices, predicting economic growth, analyzing investment returns. Higher-degree polynomials can capture the volatility and non-linear behavior often seen in financial markets.
- Climate Science: Analyzing temperature trends, projecting sea-level rise, modeling greenhouse gas emissions. Polynomial models can accommodate accelerating or decelerating changes in environmental variables.
- Engineering: Designing curves for roads, railways, and other infrastructure projects. Polynomial curves offer flexibility in creating smooth, continuous paths that meet specific design criteria.
- Biology: Modeling population growth, analyzing the growth of organisms, studying the spread of diseases. Polynomial functions can capture the sigmoidal growth patterns common in biological systems.
- Data Science: Smoothing noisy time series data, identifying trends in large datasets, and forecasting future values. Polynomial regression is a fundamental tool in data analysis and machine learning.
3. Challenges and Solutions:
While powerful, polynomial trending presents several challenges:
- Overfitting: Higher-degree polynomials can overfit the data, capturing noise rather than the underlying trend. This leads to poor predictive accuracy. Techniques like cross-validation and regularization can help mitigate overfitting.
- Extrapolation: Extrapolating beyond the range of the observed data can be unreliable, especially with higher-degree polynomials. The extrapolated values may deviate significantly from the true trend. Caution is necessary when making predictions outside the observed data range.
- Interpretation: Interpreting the coefficients of higher-degree polynomials can be difficult. The coefficients do not have a direct, intuitive meaning as they do in linear regression. Focus should be on the overall shape of the curve and its predictive power.
- Data Preprocessing: Outliers and data errors can significantly impact the accuracy of polynomial trend fitting. Proper data cleaning and preprocessing are crucial steps.
4. Impact on Innovation:
Polynomial trending contributes to innovation by enabling more accurate predictions and better understanding of complex systems. In finance, it improves forecasting models; in climate science, it refines projections of future climate change; in engineering, it facilitates the design of safer and more efficient infrastructure. The ability to model non-linear trends unlocks possibilities for innovation across multiple sectors.
Exploring the Connection Between Data Transformation and Polynomial Trending:
Data transformation plays a crucial role in enhancing the effectiveness of polynomial trending. Often, raw data doesn't exhibit a clear polynomial relationship. Transformations such as logarithmic, square root, or Box-Cox transformations can linearize the relationship, making polynomial fitting more accurate and meaningful. For example, if the data exhibits exponential growth, a logarithmic transformation can convert it into a linear relationship, making linear regression (a first-degree polynomial) sufficient. This reduces complexity and prevents overfitting associated with higher-degree polynomials.
Key Factors to Consider:
- Roles and Real-World Examples: Transformations like logarithmic transformations are particularly useful when dealing with data exhibiting exponential growth. For instance, in modeling population growth, a logarithmic transformation can linearize the data, allowing for a simpler linear regression model.
- Risks and Mitigations: Inappropriate transformations can distort the data and lead to misleading conclusions. Careful consideration of the data's distribution and underlying process is critical before applying any transformation.
- Impact and Implications: Effective data transformation enhances the accuracy, interpretability, and efficiency of polynomial trending. It facilitates more reliable predictions and provides deeper insights into the underlying processes.
Conclusion: Reinforcing the Connection:
The interplay between data transformation and polynomial trending highlights the importance of a thorough understanding of data characteristics. By carefully selecting and applying appropriate transformations, analysts can improve the accuracy and reliability of polynomial trend models, leading to more effective forecasting and decision-making.
Further Analysis: Examining Data Preprocessing in Greater Detail:
Data preprocessing is a critical step before applying polynomial trending. It involves several procedures:
- Outlier Detection and Removal: Outliers can unduly influence the fitted polynomial curve. Robust statistical methods like the median absolute deviation (MAD) can identify and remove or adjust outliers.
- Data Smoothing: Noisy data can obscure the underlying trend. Smoothing techniques like moving averages or Savitzky-Golay filters can reduce noise and reveal the underlying pattern.
- Missing Data Imputation: Missing data points can disrupt the trend analysis. Imputation methods, such as linear interpolation or k-nearest neighbors, can fill in missing values.
FAQ Section: Answering Common Questions About Polynomial Trending:
Q: What is the best degree of polynomial to use?
A: There's no single "best" degree. The optimal degree depends on the specific data and the underlying trend. Start with a low-degree polynomial and gradually increase the degree until a satisfactory fit is achieved, while carefully watching for signs of overfitting. Techniques like cross-validation can help select the optimal degree.
Q: How do I interpret the coefficients of a polynomial trend?
A: The interpretation of coefficients is less straightforward than in linear regression. Focus on the overall shape of the curve and its predictive power rather than the individual coefficient values.
Q: What are the limitations of polynomial trending?
A: Polynomial trending assumes a smooth, continuous trend. It may not be suitable for data with abrupt changes or discontinuities. Extrapolation beyond the observed data range can be unreliable. Overfitting is a potential issue with higher-degree polynomials.
Practical Tips: Maximizing the Benefits of Polynomial Trending:
- Visualize your data: Plot your data to assess the overall trend and identify potential outliers or non-linear patterns.
- Experiment with different polynomial degrees: Start with a low degree and gradually increase it, monitoring the fit and looking for signs of overfitting.
- Use cross-validation: This technique helps to assess the predictive accuracy of the model and avoid overfitting.
- Consider data transformations: Transform your data if necessary to linearize the relationship and improve the fit.
- Interpret the results carefully: Focus on the overall trend and predictive ability of the model, rather than individual coefficients.
Final Conclusion: Wrapping Up with Lasting Insights:
Polynomial trending is a powerful technique for modeling non-linear trends in data. By understanding its capabilities and limitations, and by employing appropriate data preprocessing and model selection techniques, researchers and analysts can leverage this method to gain valuable insights and make more accurate predictions across a wide range of applications. Its adaptability and versatility make it an essential tool in the modern data analyst's arsenal. The continued development and refinement of polynomial trending methods will undoubtedly lead to further advancements in forecasting and understanding complex systems.
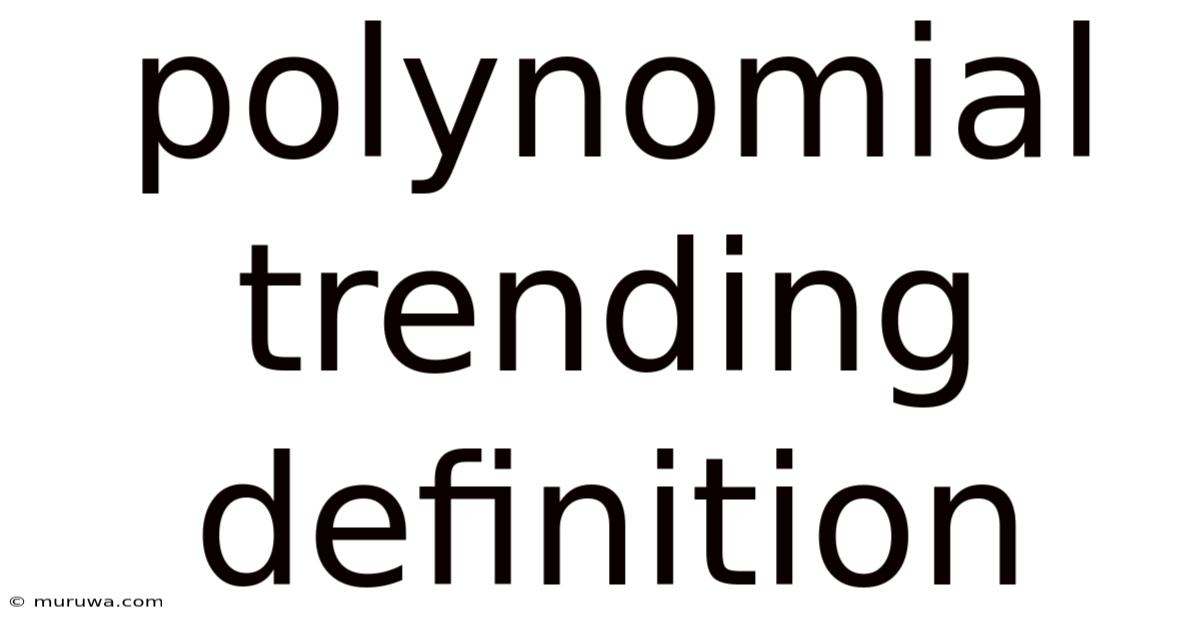
Thank you for visiting our website wich cover about Polynomial Trending Definition. We hope the information provided has been useful to you. Feel free to contact us if you have any questions or need further assistance. See you next time and dont miss to bookmark.
Also read the following articles
Article Title | Date |
---|---|
Pledging Requirement Definition | Mar 10, 2025 |
Personal Lines Insurance Definition How It Works And Coverage | Mar 10, 2025 |
What Is A Preferred Line Of Credit | Mar 10, 2025 |
Poverty Gap Definition Measurement Index | Mar 10, 2025 |
Pin Cashing Definition How It Works Examples | Mar 10, 2025 |