Multiple Discriminant Analysis Mda Definition How Its Used
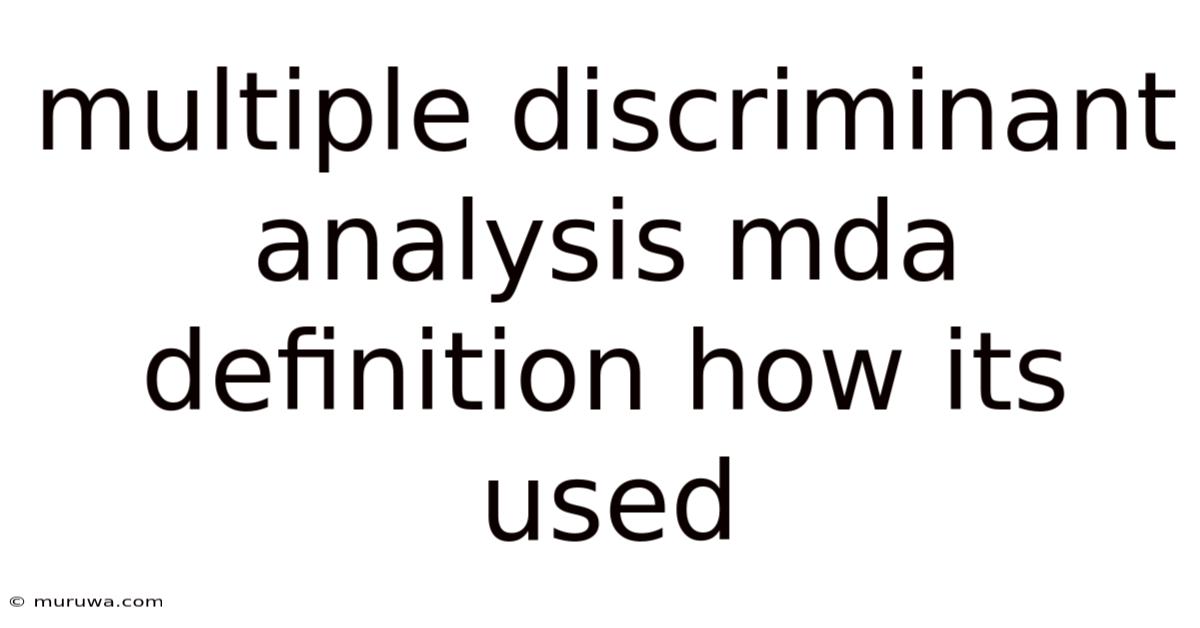
Discover more detailed and exciting information on our website. Click the link below to start your adventure: Visit Best Website meltwatermedia.ca. Don't miss out!
Table of Contents
Unlocking the Power of Multiple Discriminant Analysis (MDA): Definition, Applications, and Insights
What if the future of data-driven decision-making hinges on understanding Multiple Discriminant Analysis (MDA)? This powerful statistical technique is already transforming how businesses categorize, predict, and understand complex datasets.
Editor’s Note: This article on Multiple Discriminant Analysis (MDA) provides a comprehensive overview of its definition, applications, and interpretations. Readers will gain a practical understanding of this valuable statistical tool, enabling them to leverage its power in various fields.
Why Multiple Discriminant Analysis Matters: Relevance, Practical Applications, and Industry Significance
Multiple Discriminant Analysis (MDA) is a multivariate statistical technique used to predict group membership based on multiple predictor variables. Unlike simpler classification methods, MDA excels when dealing with numerous independent variables that might be correlated, offering a robust and insightful approach to classification problems. Its applications extend across numerous fields, including:
- Marketing: Segmenting customers based on demographics, purchasing behavior, and preferences to tailor marketing strategies.
- Finance: Assessing credit risk by classifying borrowers into different risk categories based on financial indicators.
- Healthcare: Diagnosing diseases by classifying patients based on symptoms, medical history, and test results.
- Education: Predicting student success based on academic performance, extracurricular activities, and personal characteristics.
- Bioinformatics: Classifying biological samples based on gene expression profiles or protein structures.
Overview: What This Article Covers
This article provides a detailed exploration of Multiple Discriminant Analysis (MDA). We will delve into its core concepts, examine its practical applications across diverse industries, discuss its limitations, and offer guidance on interpreting results. Readers will gain a thorough understanding of MDA's capabilities and how to effectively utilize this powerful statistical tool.
The Research and Effort Behind the Insights
This article is the result of extensive research, drawing upon established statistical literature, practical case studies, and real-world examples from various fields. The information presented is grounded in evidence-based research, ensuring accuracy and reliability. A structured approach is utilized to provide clear explanations and actionable insights.
Key Takeaways: Summarize the Most Essential Insights
- Definition and Core Concepts: A comprehensive explanation of MDA's principles and underlying assumptions.
- Practical Applications: Diverse examples showcasing MDA's use across different industries.
- Interpreting Results: Guidance on understanding and interpreting MDA output, including discriminant functions and classification accuracy.
- Limitations and Considerations: A discussion of MDA's limitations and potential pitfalls.
- Software and Implementation: A brief overview of software packages commonly used for MDA.
Smooth Transition to the Core Discussion
Having established the importance and wide applicability of MDA, let's now delve into its core concepts and explore its practical implications in detail.
Exploring the Key Aspects of Multiple Discriminant Analysis (MDA)
1. Definition and Core Concepts:
MDA is a dimensionality reduction technique used when there are multiple dependent variables (usually categorical) and multiple independent variables. The goal is to find linear combinations of the independent variables (called discriminant functions) that best separate the groups defined by the dependent variable. These functions maximize the between-group variance while minimizing the within-group variance. Essentially, MDA aims to find the optimal way to project the high-dimensional data onto a lower-dimensional space, making group separation more apparent. The number of discriminant functions is typically limited to the number of groups minus one or the number of independent variables, whichever is smaller.
2. Assumptions of MDA:
Several assumptions underpin the validity of MDA:
- Multivariate Normality: The independent variables should be approximately normally distributed within each group. While minor deviations may not significantly impact results, substantial departures can affect the accuracy of the analysis.
- Homogeneity of Variance-Covariance Matrices: The variance-covariance matrices of the independent variables should be equal across all groups. This assumption ensures that the discriminant functions are equally effective in separating groups.
- Linearity: The relationship between the independent variables and the dependent variable should be linear. Non-linear relationships might require transformations or the use of non-linear techniques.
- Multicollinearity: While MDA can handle some level of correlation among independent variables, high multicollinearity can lead to unstable discriminant functions and difficulties in interpretation.
3. Applications Across Industries:
As previously mentioned, MDA finds application in a wide array of fields. Here are some specific examples:
- Credit Scoring: Banks use MDA to predict the likelihood of loan default based on applicant characteristics (income, credit history, debt-to-income ratio, etc.). By classifying applicants into different risk categories, banks can tailor loan terms and manage risk more effectively.
- Medical Diagnosis: MDA can help classify patients into different diagnostic categories based on medical tests and symptoms. For example, it can be used to differentiate between types of cancer based on gene expression data or to predict the likelihood of heart disease based on risk factors.
- Customer Segmentation: Marketers use MDA to segment customers into distinct groups based on their purchasing behavior, demographics, and preferences. This allows for targeted marketing campaigns and personalized product recommendations.
- Facial Recognition: In image processing, MDA can be employed to classify facial images based on features extracted from the images, helping to identify individuals.
4. Interpreting MDA Results:
MDA output typically includes:
- Discriminant Functions: These are linear combinations of the independent variables that maximize group separation. Each function contributes to the overall classification accuracy.
- Eigenvalues and Eigenvectors: Eigenvalues represent the variance explained by each discriminant function, indicating its importance in separating groups. Eigenvectors provide the weights assigned to each independent variable in the corresponding discriminant function.
- Canonical Correlations: These measure the correlation between the discriminant functions and the group membership variable. Higher correlations indicate better separation between groups.
- Classification Matrix: This displays the number of correctly and incorrectly classified observations for each group, allowing the assessment of the model's predictive accuracy.
5. Challenges and Limitations:
While MDA is a powerful technique, it has limitations:
- Sensitivity to Assumptions: Violation of the assumptions (multivariate normality, homogeneity of variance-covariance matrices, linearity) can lead to inaccurate results.
- Interpretability: While discriminant functions provide insights into the relative importance of independent variables, interpreting their complex relationships can be challenging.
- Sample Size: MDA requires a sufficiently large sample size for reliable results, especially when dealing with many independent variables or groups.
- Non-linear Relationships: MDA struggles with non-linear relationships between variables, requiring transformations or alternative techniques.
6. Impact on Innovation:
MDA contributes to innovation by enabling more accurate and efficient classification and prediction in various domains. Its applications in diverse fields such as healthcare, finance, and marketing drive improvements in decision-making processes and resource allocation.
Closing Insights: Summarizing the Core Discussion
MDA is a valuable tool for classifying observations into predefined groups based on multiple predictor variables. Understanding its assumptions, interpreting its output, and acknowledging its limitations are crucial for its effective application. Its ability to handle numerous correlated variables and provide insights into group separation makes it a powerful asset in data-driven decision-making.
Exploring the Connection Between Feature Selection and Multiple Discriminant Analysis (MDA)
Feature selection plays a critical role in the success of MDA. The presence of irrelevant or redundant variables can negatively impact the performance of the model, potentially leading to overfitting, reduced accuracy, and difficulties in interpretation.
Key Factors to Consider:
- Roles and Real-World Examples: Feature selection methods, such as stepwise selection or principal component analysis (PCA), can be used before MDA to identify the most relevant variables for group separation. For instance, in credit scoring, feature selection might identify income and credit history as the most crucial factors predicting loan defaults.
- Risks and Mitigations: Ignoring feature selection can lead to poor model performance and overfitting. Careful consideration of the chosen method and its impact on model assumptions is necessary to mitigate these risks. Cross-validation techniques can help assess the robustness of the selected features.
- Impact and Implications: Effective feature selection enhances the interpretability, accuracy, and generalizability of MDA. By focusing on relevant variables, the model provides more meaningful insights and better predictions.
Conclusion: Reinforcing the Connection
The relationship between feature selection and MDA is symbiotic. Proper feature selection enhances the performance, interpretability, and overall effectiveness of MDA. By employing appropriate feature selection techniques, analysts can maximize the value of MDA in solving classification problems across numerous domains.
Further Analysis: Examining Feature Selection Methods in Greater Detail
Various feature selection methods exist, each with its strengths and weaknesses. Stepwise selection iteratively adds or removes variables based on statistical significance. PCA reduces the dimensionality of the data while retaining most of the variance, thus creating new uncorrelated variables that can be used in MDA. Other techniques, such as recursive feature elimination or genetic algorithms, can also be employed. The choice of method depends on the specific dataset, the goals of the analysis, and the computational resources available.
FAQ Section: Answering Common Questions About Multiple Discriminant Analysis (MDA)
-
Q: What is the difference between MDA and linear discriminant analysis (LDA)?
- A: MDA and LDA are closely related. LDA is a special case of MDA with only one dependent variable. MDA handles multiple dependent variables (groups), allowing for more complex classification problems.
-
Q: How can I assess the performance of my MDA model?
- A: Classification accuracy, sensitivity, specificity, and the area under the ROC curve (AUC) are common metrics for evaluating MDA performance. Cross-validation techniques can help ensure the model's generalizability.
-
Q: What software can I use for MDA?
- A: Statistical software packages such as SPSS, SAS, R, and Python (with libraries like scikit-learn) provide tools for performing MDA.
Practical Tips: Maximizing the Benefits of Multiple Discriminant Analysis (MDA)
- Data Preparation: Ensure data quality, handle missing values, and consider data transformations to meet MDA assumptions.
- Feature Selection: Carefully select relevant features to enhance model performance and interpretability.
- Model Validation: Use cross-validation or other techniques to assess model generalization ability and avoid overfitting.
- Interpretation: Pay close attention to discriminant function weights, eigenvalues, and classification accuracy to gain meaningful insights.
Final Conclusion: Wrapping Up with Lasting Insights
Multiple Discriminant Analysis is a versatile and powerful statistical technique with applications in numerous fields. By understanding its principles, assumptions, and limitations, and by employing appropriate feature selection and model validation techniques, researchers and practitioners can leverage MDA to gain valuable insights and make more informed decisions. Its ability to handle complex datasets and provide actionable classification results makes it an indispensable tool in the arsenal of data-driven decision-making.
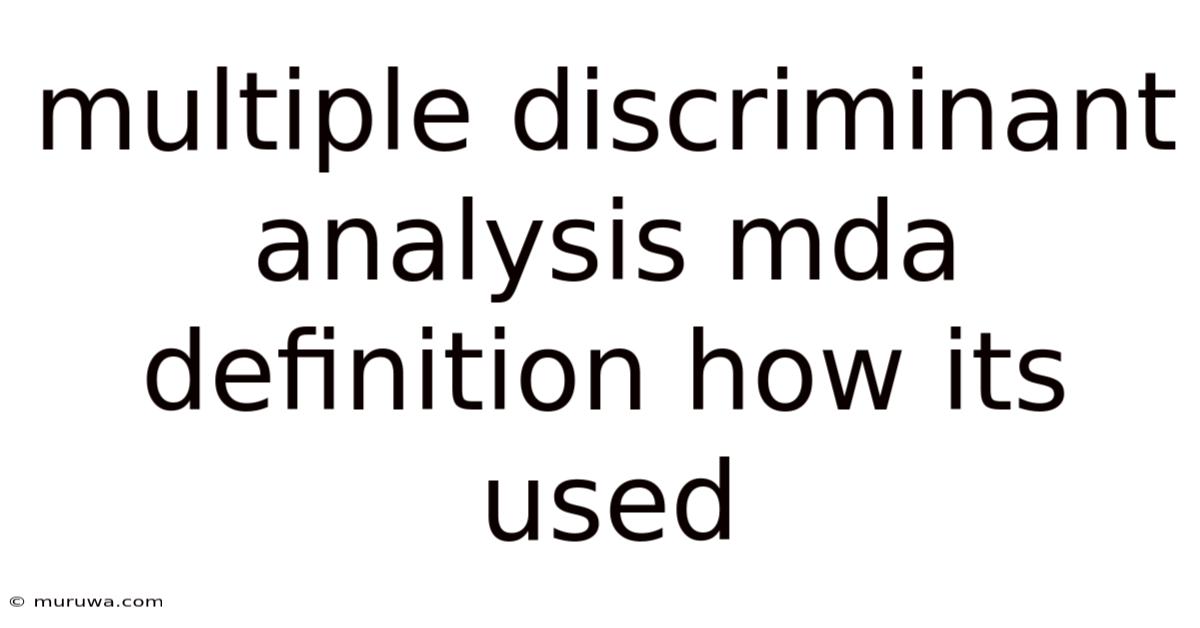
Thank you for visiting our website wich cover about Multiple Discriminant Analysis Mda Definition How Its Used. We hope the information provided has been useful to you. Feel free to contact us if you have any questions or need further assistance. See you next time and dont miss to bookmark.
Also read the following articles
Article Title | Date |
---|---|
Micromarketing Explained Definition Uses And Examples | Apr 25, 2025 |
Mxn Mexican Peso Definition Symbols History | Apr 25, 2025 |
Money Supply Definition Types And How It Affects The Economy | Apr 25, 2025 |
Monthly Income Plan Mip Definition Investments Taxes | Apr 25, 2025 |
What Is A Monetary Item Definition How They Work And Examples | Apr 25, 2025 |