Linear Relationship Definition
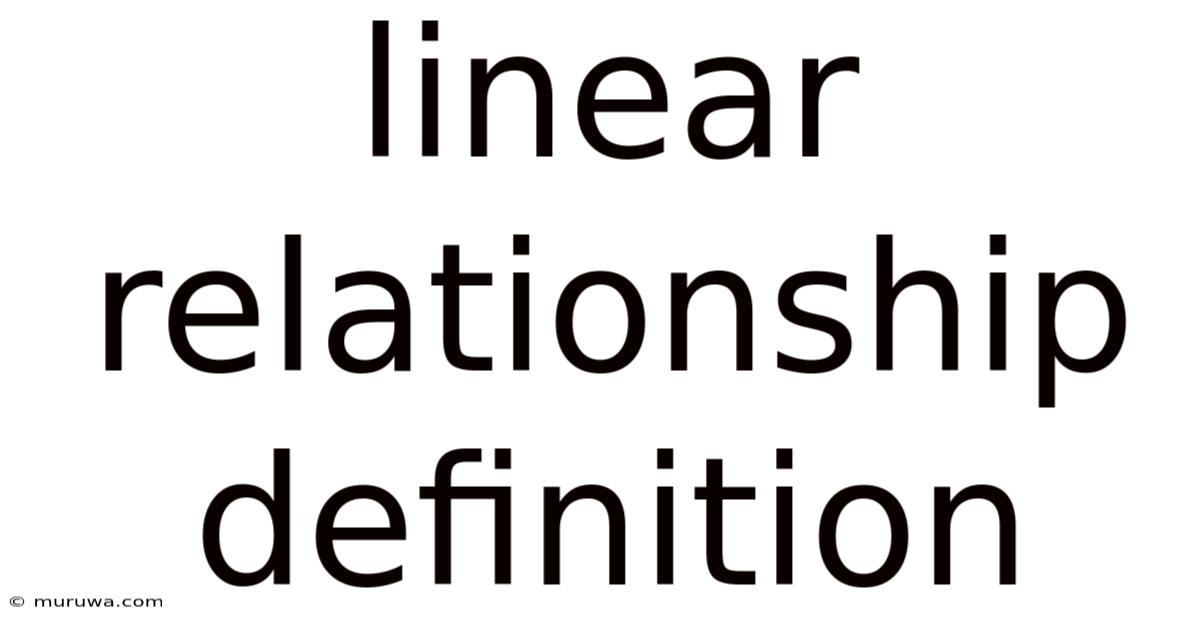
Discover more detailed and exciting information on our website. Click the link below to start your adventure: Visit Best Website meltwatermedia.ca. Don't miss out!
Table of Contents
Unraveling the Linear Relationship: Definition, Applications, and Interpretations
What if the very foundation of predictive modeling rests on understanding linear relationships? This fundamental concept underpins numerous fields, offering powerful tools for analysis and forecasting.
Editor's Note: This comprehensive article on linear relationships provides a detailed exploration of this critical concept, covering its definition, applications, and interpretations. Updated with the latest insights, this resource serves as a valuable guide for students, researchers, and professionals across diverse disciplines.
Why Linear Relationships Matter: Relevance, Practical Applications, and Industry Significance
Linear relationships are the cornerstone of many analytical techniques. Understanding them is crucial for making informed decisions across various fields, from finance and economics to engineering and the sciences. A linear relationship describes a direct proportional association between two variables: as one increases, the other increases proportionally, or as one decreases, the other decreases proportionally. This simplicity belies its profound impact. Linear relationships are essential for:
- Predictive Modeling: Forecasting future outcomes based on past data relies heavily on identifying and utilizing linear relationships. This is fundamental to time-series analysis, demand forecasting, and risk assessment.
- Statistical Analysis: Regression analysis, a core statistical tool, hinges on the identification of linear relationships between variables. This allows researchers to quantify the strength and direction of these relationships.
- Scientific Research: Many scientific phenomena can be modeled using linear relationships, providing a simplified yet effective framework for understanding complex systems. Examples include Ohm's Law in physics and certain aspects of chemical kinetics.
- Engineering and Design: Linear relationships are fundamental to engineering design, used in structural analysis, circuit design, and control systems. Understanding these relationships ensures stability and efficiency.
- Business and Finance: Linear models are employed extensively in financial modeling, predicting stock prices, evaluating investment portfolios, and managing risk.
Overview: What This Article Covers
This article provides a comprehensive overview of linear relationships. It begins by defining the core concept and explores its mathematical representation. We will then examine different types of linear relationships, discuss their graphical representation, and delve into the significance of the slope and intercept. Finally, we will explore the applications of linear relationships in various fields and address common misconceptions.
The Research and Effort Behind the Insights
This article draws upon extensive research from various academic sources, including textbooks on statistics, mathematics, and engineering. It incorporates examples from multiple disciplines to illustrate the wide-ranging applicability of linear relationships. The information presented is rigorously vetted to ensure accuracy and clarity.
Key Takeaways:
- Definition and Core Concepts: A precise definition of a linear relationship, including its mathematical formulation.
- Graphical Representation: Understanding how linear relationships are visually represented using graphs and their interpretation.
- Slope and Intercept: The meaning and significance of the slope and y-intercept in the context of a linear relationship.
- Types of Linear Relationships: Exploring positive, negative, and zero relationships.
- Applications Across Disciplines: Illustrative examples from various fields showcasing the practical use of linear relationships.
- Limitations and Challenges: Acknowledging the limitations of linear models and circumstances where they are unsuitable.
Smooth Transition to the Core Discussion:
With a foundational understanding of the relevance of linear relationships, let's delve into a more detailed examination of the concept itself.
Exploring the Key Aspects of Linear Relationships
1. Definition and Core Concepts:
A linear relationship between two variables, typically denoted as x and y, exists when a change in one variable results in a proportional change in the other. This relationship can be mathematically expressed as a linear equation:
y = mx + c
Where:
y
is the dependent variable.x
is the independent variable.m
is the slope, representing the rate of change of y with respect to x.c
is the y-intercept, representing the value of y when x is zero.
This equation implies that for every unit increase in x, y changes by m units. If m is positive, the relationship is positive (as x increases, y increases); if m is negative, the relationship is negative (as x increases, y decreases). If m is zero, there is no linear relationship between x and y.
2. Graphical Representation:
A linear relationship is graphically represented as a straight line. The slope (m) determines the steepness of the line, and the y-intercept (c) indicates where the line crosses the y-axis. A positive slope indicates an upward-sloping line, a negative slope indicates a downward-sloping line, and a slope of zero indicates a horizontal line.
3. Slope and Intercept:
The slope and y-intercept are crucial parameters in understanding a linear relationship. The slope quantifies the strength and direction of the relationship, while the y-intercept provides a baseline value. In practical applications, the slope and intercept often carry specific meanings relevant to the context of the problem. For example, in a linear model predicting sales based on advertising expenditure, the slope might represent the return on investment for each dollar spent on advertising, and the y-intercept might represent the baseline sales level even without advertising.
4. Types of Linear Relationships:
- Positive Linear Relationship: As the independent variable (x) increases, the dependent variable (y) also increases. The slope (m) is positive. Example: The relationship between study time and exam scores.
- Negative Linear Relationship: As the independent variable (x) increases, the dependent variable (y) decreases. The slope (m) is negative. Example: The relationship between price and demand for a product.
- Zero Linear Relationship: There is no relationship between the independent and dependent variables. The slope (m) is zero. Example: The relationship between shoe size and IQ.
5. Applications Across Disciplines:
Linear relationships find applications in a myriad of fields. Some examples include:
- Physics: Ohm's Law (V = IR), describing the relationship between voltage, current, and resistance.
- Economics: Supply and demand curves, depicting the relationship between price and quantity.
- Finance: Capital Asset Pricing Model (CAPM), used to determine the expected rate of return for an asset.
- Engineering: Stress-strain relationships in materials science, relating the applied stress to the resulting strain.
- Biology: Growth curves in populations under ideal conditions, often exhibiting a linear relationship initially.
Closing Insights: Summarizing the Core Discussion
Linear relationships are fundamental mathematical concepts with vast practical implications across diverse disciplines. Understanding the definition, graphical representation, and interpretation of slope and intercept is crucial for analyzing data, building predictive models, and making informed decisions. The simplicity of the linear model makes it a powerful tool, although its limitations should be acknowledged when dealing with complex, non-linear relationships.
Exploring the Connection Between Correlation and Linear Relationships
Correlation and linear relationships are closely related but distinct concepts. Correlation measures the strength and direction of a linear association between two variables, while a linear relationship describes the specific mathematical form of that association (represented by the linear equation y = mx + c). A high correlation coefficient suggests a strong linear relationship, but it doesn't guarantee a perfectly linear relationship or specify the exact equation. There could still be some degree of scatter or deviation from a perfectly straight line. Conversely, a low or zero correlation coefficient suggests either a weak or non-existent linear relationship, although a non-linear relationship might still exist between the variables.
Key Factors to Consider:
-
Roles and Real-World Examples: Correlation helps quantify the strength of a linear relationship, assisting in predictive modeling and identifying significant variables. For example, a high positive correlation between advertising expenditure and sales suggests a strong linear relationship that can be exploited for forecasting.
-
Risks and Mitigations: Misinterpreting correlation as causation is a common pitfall. A strong correlation doesn't imply causality; a third, unobserved variable might be driving both. Careful consideration of potential confounding variables is necessary.
-
Impact and Implications: Understanding correlation strengthens the ability to build robust predictive models, enhancing decision-making in various fields. However, overreliance on correlation without considering causality can lead to inaccurate conclusions and ineffective strategies.
Conclusion: Reinforcing the Connection
The relationship between correlation and linear relationships is crucial. Correlation provides a measure of the strength of a linear association, while a linear model provides the mathematical framework for understanding and predicting that association. However, a clear understanding of both concepts, including their limitations, is vital for responsible data analysis and informed decision-making.
Further Analysis: Examining Correlation Coefficients in Greater Detail
Correlation coefficients, typically denoted by r, range from -1 to +1. r = +1 indicates a perfect positive linear correlation, r = -1 indicates a perfect negative linear correlation, and r = 0 indicates no linear correlation. The magnitude of r reflects the strength of the correlation, while its sign indicates the direction. Interpreting correlation coefficients requires careful consideration of the sample size and potential outliers, which can influence the value of r. Different correlation coefficients exist for different data types (e.g., Pearson's correlation for continuous data, Spearman's rank correlation for ordinal data).
FAQ Section: Answering Common Questions About Linear Relationships
-
What is a linear relationship? A linear relationship exists between two variables when a change in one variable produces a proportional change in the other, representable by a straight line on a graph.
-
How do I determine if a relationship is linear? Visual inspection of a scatter plot can provide a preliminary indication. Statistical methods like calculating correlation coefficients or performing linear regression analysis provide more rigorous assessments.
-
What are the limitations of linear models? Linear models assume a constant rate of change, which may not always hold true in real-world scenarios. They are not suitable for modeling non-linear relationships. Outliers can significantly influence the results of linear regression.
-
What if my data doesn't perfectly fit a straight line? Real-world data rarely fits perfectly. Linear regression seeks to find the "best-fitting" line, minimizing the overall deviation between the data points and the line. Transforming the data (e.g., using logarithmic or exponential transformations) might improve the linearity.
Practical Tips: Maximizing the Benefits of Linear Relationship Analysis
-
Visualize Your Data: Create scatter plots to visually inspect for potential linear relationships before performing any statistical analysis.
-
Calculate Correlation Coefficients: Quantify the strength and direction of linear relationships using appropriate correlation coefficients.
-
Perform Linear Regression: Fit a linear model to your data to obtain the equation of the line and evaluate its goodness of fit.
-
Interpret Results Cautiously: Avoid drawing causal conclusions solely based on correlation. Consider potential confounding variables and limitations of linear models.
-
Explore Data Transformations: If your data shows a non-linear relationship, consider transforming the variables to improve linearity.
Final Conclusion: Wrapping Up with Lasting Insights
Understanding linear relationships is fundamental to numerous fields. By mastering the concepts of correlation, linear equations, and regression analysis, individuals can unlock powerful tools for data analysis, prediction, and decision-making. However, it's crucial to remember the limitations of linear models and apply them responsibly. Continual learning and critical evaluation are essential for effective utilization of this fundamental concept.
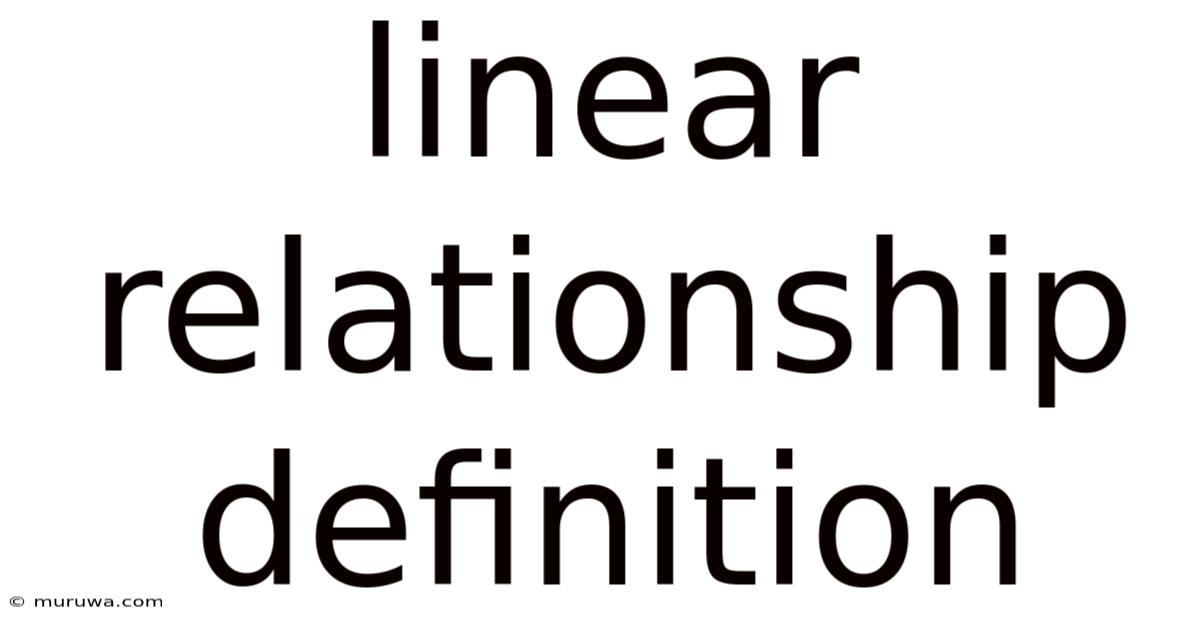
Thank you for visiting our website wich cover about Linear Relationship Definition. We hope the information provided has been useful to you. Feel free to contact us if you have any questions or need further assistance. See you next time and dont miss to bookmark.
Also read the following articles
Article Title | Date |
---|---|
Buy Stops Above Definition And Example | Apr 23, 2025 |
Lock Up Agreement Definition Purpose And Example | Apr 23, 2025 |
Buy The Dips Definition | Apr 23, 2025 |
Limit Down Definition And How It Works For Stocks And Futures | Apr 23, 2025 |
Land Contract Definition | Apr 23, 2025 |