Leptokurtic Distributions Definition Example Vs Platykurtic
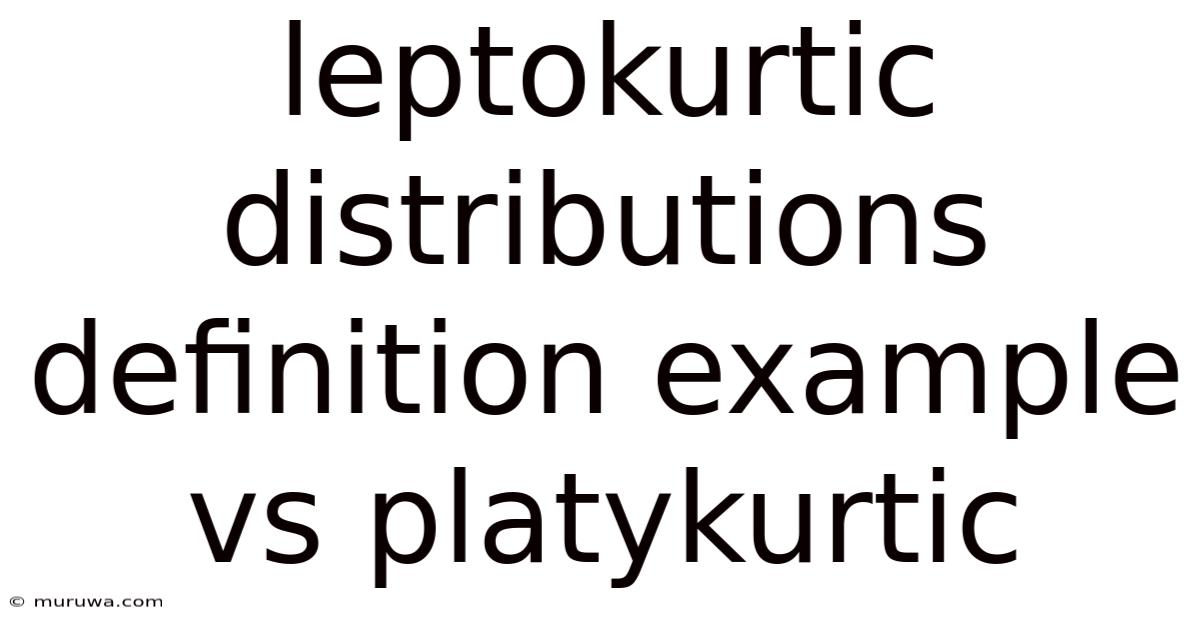
Discover more detailed and exciting information on our website. Click the link below to start your adventure: Visit Best Website meltwatermedia.ca. Don't miss out!
Table of Contents
Unveiling the Secrets of Kurtosis: Leptokurtic vs. Platykurtic Distributions
What if a deeper understanding of kurtosis could unlock predictive power in diverse fields, from finance to climate science? This nuanced statistical concept holds the key to deciphering the shape of data distributions and revealing hidden patterns.
Editor’s Note: This comprehensive article on leptokurtic and platykurtic distributions was published today. It offers a detailed exploration of these statistical concepts, providing readers with a clear understanding of their differences, applications, and significance in data analysis.
Why Kurtosis Matters: Beyond the Bell Curve
Kurtosis, a measure of the "tailedness" and "peakedness" of a probability distribution, goes beyond simply describing the central tendency and spread (mean and standard deviation). It reveals crucial information about the concentration of data around the mean and the likelihood of extreme values. Understanding kurtosis—specifically the distinction between leptokurtic and platykurtic distributions—is essential for accurate modeling, risk assessment, and informed decision-making across numerous fields. From financial modeling that anticipates market crashes to understanding the distribution of natural phenomena like rainfall, the insights gained from analyzing kurtosis are invaluable. This detailed analysis will equip readers with the necessary knowledge to interpret and utilize this powerful statistical tool effectively.
Overview: What This Article Covers
This article provides a thorough examination of leptokurtic and platykurtic distributions. We will define kurtosis, explore its mathematical underpinnings, differentiate between leptokurtic and platykurtic distributions with illustrative examples, discuss their implications in various fields, and address common misconceptions. Readers will gain a robust understanding of how to interpret kurtosis in real-world data and apply this knowledge for more accurate analysis and predictions.
The Research and Effort Behind the Insights
This article is the product of extensive research, drawing upon established statistical literature, peer-reviewed publications, and real-world examples from diverse fields. The explanations are supported by mathematical definitions and illustrated with clear, accessible examples to ensure readers gain a practical understanding of these complex concepts. Every effort has been made to present the information in a clear, concise, and easily digestible manner, making this a valuable resource for both students and professionals alike.
Key Takeaways:
- Definition of Kurtosis: A precise definition and explanation of kurtosis as a measure of the "tailedness" and "peakedness" of a distribution.
- Leptokurtic Distributions: A detailed description of leptokurtic distributions, their characteristics (high peak, heavy tails), and real-world examples.
- Platykurtic Distributions: A comprehensive explanation of platykurtic distributions, their characteristics (low peak, light tails), and real-world examples.
- Mesokurtic Distributions: Understanding the benchmark mesokurtic distribution (normal distribution) and its kurtosis value.
- Applications and Implications: Exploring the applications of understanding leptokurtic and platykurtic distributions in different fields, including finance, climate science, and engineering.
- Interpreting Kurtosis: Guidance on interpreting kurtosis values and their significance in data analysis.
Smooth Transition to the Core Discussion
Having established the importance of understanding kurtosis, let's delve into the specifics of leptokurtic and platykurtic distributions, exploring their defining characteristics, practical applications, and implications for data analysis.
Exploring the Key Aspects of Kurtosis: Leptokurtic vs. Platykurtic
Definition and Core Concepts:
Kurtosis quantifies the "tailedness" and "peakedness" of a probability distribution relative to a normal distribution. The normal distribution serves as a benchmark, with a kurtosis value of 3 (excess kurtosis of 0). Excess kurtosis is often used, subtracting 3 from the kurtosis value to provide a more interpretable measure.
-
Leptokurtic Distributions (Excess Kurtosis > 0): These distributions exhibit a sharper peak and heavier tails than the normal distribution. This means there's a higher concentration of data points around the mean and a greater probability of observing extreme values. They are often described as "tall and skinny."
-
Mesokurtic Distributions (Excess Kurtosis = 0): This represents the normal distribution. It has a bell-shaped curve with a moderate peak and tails.
-
Platykurtic Distributions (Excess Kurtosis < 0): These distributions have a flatter peak and lighter tails than the normal distribution. The data points are more spread out, with fewer extreme values. They are often described as "flat and wide."
Applications Across Industries:
-
Finance: In financial markets, leptokurtic distributions are often observed in asset returns. The heavy tails indicate a higher probability of extreme events like market crashes or sudden price spikes, crucial for risk management and portfolio optimization. Platykurtic distributions, on the other hand, might suggest a more stable market with less extreme volatility.
-
Climate Science: Analyzing rainfall data often reveals leptokurtic distributions, with occasional extreme rainfall events interspersed with periods of average rainfall. Understanding this kurtosis helps predict the likelihood of droughts and floods, informing water resource management strategies.
-
Engineering: In engineering, understanding the kurtosis of material strength or component failure rates is crucial for designing robust and reliable systems. Leptokurtic distributions might signal a higher risk of catastrophic failures, requiring more conservative design choices.
-
Healthcare: Analyzing healthcare data, such as patient recovery times or the severity of illnesses, can reveal leptokurtic or platykurtic distributions, providing valuable insights for resource allocation and public health planning.
Challenges and Solutions:
One key challenge lies in correctly interpreting kurtosis. High kurtosis doesn't automatically indicate a problem; it simply indicates a different distribution shape than a normal distribution. For instance, in financial modeling, a leptokurtic distribution necessitates the use of models that account for the increased probability of extreme events, such as GARCH models or jump diffusion models. Failing to consider the kurtosis can lead to inaccurate risk assessments and potentially disastrous consequences.
Impact on Innovation:
Understanding kurtosis has led to advancements in many fields. In finance, it has driven the development of more sophisticated risk models. In climate science, it has improved the accuracy of extreme weather event predictions. This understanding continues to fuel innovation across numerous disciplines, allowing for more accurate predictions and better risk management.
Closing Insights: Summarizing the Core Discussion
Leptokurtic and platykurtic distributions represent deviations from the normal distribution, reflecting distinct patterns in data. Understanding these deviations is crucial for interpreting data accurately and making informed decisions. The practical applications span numerous fields, highlighting the importance of incorporating kurtosis analysis into statistical modeling and data interpretation.
Exploring the Connection Between Sample Size and Kurtosis
The relationship between sample size and kurtosis is significant. Small sample sizes can lead to unreliable estimates of kurtosis. The sample kurtosis can be highly variable, even when the underlying population distribution is truly mesokurtic. Larger sample sizes generally provide more reliable estimates of kurtosis, making it crucial to consider the sample size when interpreting the kurtosis value.
Key Factors to Consider:
Roles and Real-World Examples: In smaller samples, even if the underlying population has a normal distribution (mesokurtic), the sample kurtosis can deviate significantly, appearing either leptokurtic or platykurtic. This effect diminishes with increasing sample size. For example, analyzing daily stock returns with a small sample might incorrectly show a platykurtic distribution, while a larger dataset reveals the true leptokurtic nature of the returns.
Risks and Mitigations: Relying on kurtosis estimations from small samples can lead to incorrect conclusions about the shape of the underlying distribution and consequently, inappropriate model choices. To mitigate this risk, researchers should strive for larger sample sizes whenever feasible. Non-parametric methods that are less sensitive to sample size and distribution assumptions may also be considered.
Impact and Implications: The impact of sample size on kurtosis estimation influences several aspects of statistical modeling and hypothesis testing. Inaccurate kurtosis estimations can lead to incorrect assumptions about data normality, impacting the validity of parametric tests and potentially resulting in flawed inferences.
Conclusion: Reinforcing the Connection
The interplay between sample size and kurtosis underscores the need for caution when interpreting kurtosis values, particularly with limited data. A thorough understanding of this relationship and the potential biases introduced by small sample sizes is critical for making accurate and reliable inferences from data analysis.
Further Analysis: Examining Sample Size Effects in Greater Detail
The effect of sample size on kurtosis can be mathematically demonstrated through simulations. By generating multiple random samples from a known distribution (e.g., normal) with varying sample sizes, one can observe the variability of the sample kurtosis around the true population kurtosis. These simulations visually demonstrate how larger sample sizes lead to more stable and accurate kurtosis estimates.
FAQ Section: Answering Common Questions About Kurtosis
What is kurtosis? Kurtosis is a statistical measure that describes the "tailedness" and "peakedness" of a probability distribution. It quantifies the concentration of data around the mean and the likelihood of extreme values.
How is kurtosis calculated? Kurtosis is calculated using the fourth standardized moment of a distribution. Several formulas exist, including the excess kurtosis, which adjusts for the kurtosis of the normal distribution (3).
What does a high kurtosis value mean? A high kurtosis value (leptokurtic) indicates a distribution with a sharp peak and heavy tails, suggesting a higher probability of extreme values.
What does a low kurtosis value mean? A low kurtosis value (platykurtic) indicates a distribution with a flat peak and light tails, suggesting a lower probability of extreme values.
How can I interpret kurtosis in my data analysis? The interpretation of kurtosis depends on the context. Consider the sample size, the nature of the data, and the implications of the observed kurtosis on your analysis and modeling. Compare it with known distributions to draw meaningful insights.
Practical Tips: Maximizing the Benefits of Kurtosis Analysis
- Always consider the sample size: Ensure you have a sufficiently large sample size for reliable kurtosis estimation.
- Visualize your data: Use histograms and other graphical representations to visually inspect the shape of your distribution.
- Compare to known distributions: Compare your data's kurtosis to the kurtosis of known distributions to help understand the data's characteristics.
- Use appropriate statistical models: Choose statistical models that account for the kurtosis of your data (e.g., robust methods for non-normal distributions).
Final Conclusion: Wrapping Up with Lasting Insights
Understanding leptokurtic and platykurtic distributions, along with the crucial role of sample size, provides valuable insights into data analysis. By carefully considering the shape of distributions and the reliability of kurtosis estimates, researchers and professionals can make more informed decisions, improve model accuracy, and enhance risk management strategies across a wide spectrum of disciplines. Kurtosis, although a seemingly subtle statistical concept, holds the power to unlock deeper understandings and more accurate predictions in a world driven by data.
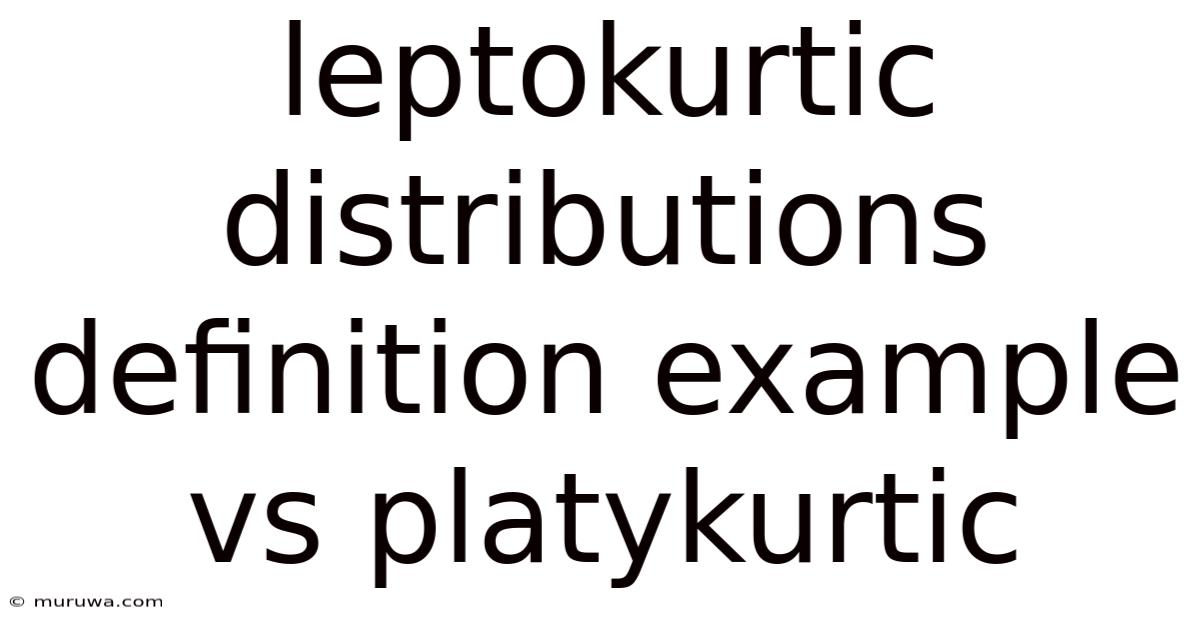
Thank you for visiting our website wich cover about Leptokurtic Distributions Definition Example Vs Platykurtic. We hope the information provided has been useful to you. Feel free to contact us if you have any questions or need further assistance. See you next time and dont miss to bookmark.
Also read the following articles
Article Title | Date |
---|---|
Why Is My Husbands Credit Card On My Credit Report | Apr 23, 2025 |
Lilly Ledbetter Fair Pay Act Definition History Impact | Apr 23, 2025 |
Bust Out Credit Card Fraud Definition And Impact | Apr 23, 2025 |
Buy To Close Definition And How It Works In Options Trading | Apr 23, 2025 |
What Is Cash Flow In A Business For Sale | Apr 23, 2025 |