Kurtosis Definition Types And Importance
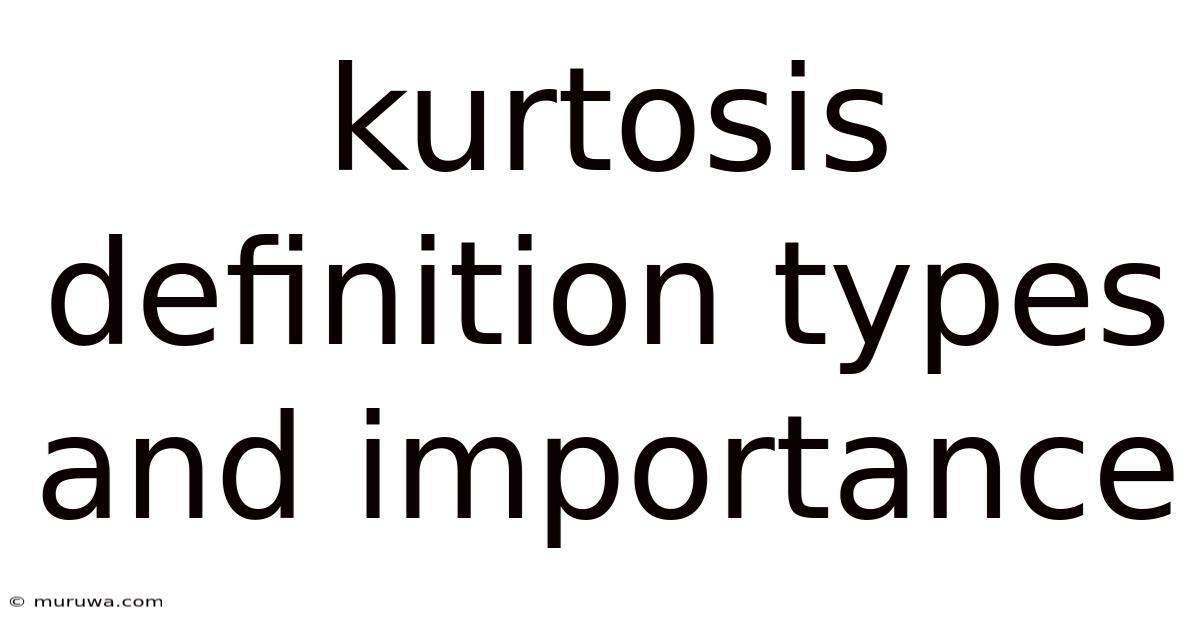
Discover more detailed and exciting information on our website. Click the link below to start your adventure: Visit Best Website meltwatermedia.ca. Don't miss out!
Table of Contents
Unveiling Kurtosis: Definition, Types, and Importance
What if the seemingly simple shape of a data distribution holds the key to unlocking deeper insights about its underlying processes? Kurtosis, a measure of the "tailedness" and "peakedness" of a distribution, is precisely that key, offering invaluable insights across diverse fields.
Editor’s Note: This article on kurtosis provides a comprehensive exploration of its definition, types, and importance across various disciplines. The information presented is current and supported by statistical principles.
Why Kurtosis Matters: Relevance, Practical Applications, and Industry Significance
Kurtosis, a crucial statistical concept, goes beyond simply describing the shape of a data distribution. It unveils crucial information about the likelihood of extreme values and the concentration of data around the mean. Understanding kurtosis is vital in various fields, including finance (assessing risk), engineering (analyzing material strength), healthcare (studying disease prevalence), and social sciences (investigating income inequality). Its practical applications are extensive, enabling more accurate predictions, informed decision-making, and a deeper comprehension of complex phenomena.
Overview: What This Article Covers
This article provides a thorough exploration of kurtosis, starting with its precise definition and delving into the different types – mesokurtic, leptokurtic, and platykurtic. We'll examine its calculation, explore its significance in various contexts, and address common misconceptions. The article concludes by highlighting practical applications and offering actionable insights for utilizing kurtosis in data analysis.
The Research and Effort Behind the Insights
This article is the result of extensive research, drawing upon established statistical literature, academic papers, and practical applications across multiple disciplines. The explanations are supported by clear examples and illustrative diagrams, ensuring readers receive accurate and readily understandable information.
Key Takeaways:
- Definition and Core Concepts: A precise understanding of kurtosis and its relationship to the shape of a distribution.
- Types of Kurtosis: Differentiation between mesokurtic, leptokurtic, and platykurtic distributions and their implications.
- Calculating Kurtosis: Methods for calculating kurtosis, including excess kurtosis.
- Interpreting Kurtosis: Understanding the significance of kurtosis values and their practical interpretations.
- Applications Across Industries: Real-world examples of kurtosis's use in diverse fields.
- Limitations of Kurtosis: Awareness of the potential pitfalls and limitations of relying solely on kurtosis.
Smooth Transition to the Core Discussion
Having established the importance and scope of our topic, let's delve into the core aspects of kurtosis, starting with its precise definition and then exploring its different types and practical implications.
Exploring the Key Aspects of Kurtosis
Definition and Core Concepts:
Kurtosis is a statistical measure that describes the "tailedness" and "peakedness" of a probability distribution. It quantifies the concentration of data around the mean and the presence of outliers. A higher kurtosis value suggests a sharper peak and heavier tails (more extreme values), while a lower kurtosis value indicates a flatter peak and lighter tails. It's crucial to remember that kurtosis measures the relative peakedness and tailedness compared to a normal distribution.
Types of Kurtosis:
Kurtosis is often categorized into three types based on its relationship to the kurtosis of a normal distribution:
-
Mesokurtic: A mesokurtic distribution has a kurtosis similar to that of a normal distribution (approximately 3 for the sample kurtosis and 0 for excess kurtosis). The data is relatively evenly distributed around the mean, with a moderate peak and tails.
-
Leptokurtic: A leptokurtic distribution exhibits higher kurtosis than a normal distribution (kurtosis > 3, excess kurtosis > 0). It is characterized by a sharper peak and heavier tails, indicating a greater concentration of data around the mean and a higher probability of extreme values. Leptokurtic distributions are often associated with higher risk in financial modeling or increased potential for unexpected events in other fields.
-
Platykurtic: A platykurtic distribution has lower kurtosis than a normal distribution (kurtosis < 3, excess kurtosis < 0). It features a flatter peak and lighter tails compared to a normal distribution, suggesting a more uniform spread of data and fewer extreme values.
Calculating Kurtosis:
Kurtosis is typically calculated using two main approaches:
-
Sample Kurtosis: This is calculated directly from the data using the following formula:
k = (n(n+1))/((n-1)(n-2)(n-3)) * Σ((xᵢ - x̄)⁴ / s⁴)
where:
- n is the sample size
- xᵢ is the i-th data point
- x̄ is the sample mean
- s is the sample standard deviation
-
Excess Kurtosis: This adjusts the sample kurtosis by subtracting 3, making the kurtosis of a normal distribution equal to 0. Excess kurtosis provides a more direct comparison between the peakedness and tailedness of different distributions relative to a normal distribution. Excess Kurtosis = Sample Kurtosis - 3
Interpreting Kurtosis:
The interpretation of kurtosis values depends on the context and the specific research question. A high kurtosis value might suggest the presence of outliers or a process generating extreme values. Conversely, low kurtosis might indicate a more homogeneous data set with less variability. It's essential to consider kurtosis alongside other descriptive statistics, such as mean, median, standard deviation, and skewness, for a comprehensive understanding of the data distribution.
Applications Across Industries:
Kurtosis finds application in numerous fields:
-
Finance: Assessing portfolio risk, identifying potential market crashes, and understanding the distribution of returns. Leptokurtic distributions of asset returns indicate a higher probability of extreme events (e.g., large gains or losses).
-
Engineering: Analyzing the strength and durability of materials, predicting equipment failures, and understanding stress distributions.
-
Healthcare: Studying the prevalence of diseases, analyzing patient health outcomes, and identifying risk factors for specific conditions.
-
Social Sciences: Investigating income inequality, analyzing voting patterns, and understanding social stratification.
-
Image Processing: Evaluating image sharpness and texture. Higher kurtosis often indicates sharper images.
Closing Insights: Summarizing the Core Discussion
Kurtosis offers a powerful tool for understanding the shape and characteristics of data distributions beyond the typical measures of central tendency and dispersion. By carefully interpreting kurtosis values and considering them alongside other descriptive statistics, researchers and analysts can gain deeper insights into the underlying processes generating the data.
Exploring the Connection Between Skewness and Kurtosis
Skewness and kurtosis are both measures of the shape of a distribution, but they capture different aspects. Skewness describes the asymmetry of the distribution, while kurtosis focuses on the peakedness and tailedness. While they are distinct, they are often considered together to provide a more comprehensive understanding of a data set’s characteristics. A highly skewed distribution may also exhibit high kurtosis, but this is not always the case. The relationship between skewness and kurtosis can be complex and requires careful consideration.
Key Factors to Consider:
-
Roles and Real-World Examples: In financial markets, a highly skewed and leptokurtic distribution of stock returns might indicate the presence of fat tails, making accurate risk assessments more challenging.
-
Risks and Mitigations: Over-reliance on kurtosis alone without considering other statistical measures can lead to misinterpretations and flawed conclusions. Careful consideration of the entire data distribution, including the context, is essential.
-
Impact and Implications: Understanding the interplay between skewness and kurtosis can enhance the accuracy of predictive models, risk management strategies, and other decision-making processes.
Conclusion: Reinforcing the Connection
The relationship between skewness and kurtosis is crucial for a complete picture of a data set's shape. By considering both measures, analysts can obtain a more nuanced understanding of the distribution's characteristics and avoid potential biases or misinterpretations that might result from focusing solely on one aspect.
Further Analysis: Examining Skewness in Greater Detail
Skewness quantifies the asymmetry of a distribution. A positive skew indicates a longer tail on the right (more high values), while a negative skew suggests a longer tail on the left (more low values). Calculating skewness involves a similar approach to kurtosis, employing the third central moment of the data. Understanding skewness, in conjunction with kurtosis, significantly enhances the ability to interpret a dataset’s characteristics.
FAQ Section: Answering Common Questions About Kurtosis
-
What is kurtosis? Kurtosis is a statistical measure that describes the "tailedness" and "peakedness" of a probability distribution. It indicates the concentration of data around the mean and the presence of outliers.
-
How is kurtosis calculated? Kurtosis is calculated using either the sample kurtosis formula or excess kurtosis (sample kurtosis - 3).
-
What does a high kurtosis value mean? A high kurtosis value suggests a sharper peak, heavier tails, and a greater probability of extreme values compared to a normal distribution.
-
What does a low kurtosis value mean? A low kurtosis value indicates a flatter peak, lighter tails, and a more uniform distribution compared to a normal distribution.
-
What are the limitations of kurtosis? Kurtosis should not be interpreted in isolation. It’s crucial to consider it with other descriptive statistics and the context of the data. Extreme values can disproportionately affect kurtosis calculations.
-
How is kurtosis used in practice? Kurtosis is used across various fields, including finance, engineering, healthcare, and social sciences, to assess risk, analyze data distributions, and make informed decisions.
Practical Tips: Maximizing the Benefits of Kurtosis
-
Visualize Your Data: Always start by creating histograms or other visualizations to get a visual sense of the distribution's shape. This aids in interpreting kurtosis values.
-
Consider the Context: Interpret kurtosis within the specific context of your data and the research question.
-
Use Multiple Measures: Don't rely solely on kurtosis. Consider skewness, mean, median, standard deviation, and other descriptive statistics for a more comprehensive analysis.
-
Be Aware of Outliers: Outliers can strongly influence kurtosis calculations. Investigate potential outliers and consider their impact on your results.
-
Explore Alternative Measures: If kurtosis seems to be misleading, explore other measures of shape or tail behavior, like quantiles or robust estimators.
Final Conclusion: Wrapping Up with Lasting Insights
Kurtosis, although sometimes overlooked, offers a crucial window into understanding the intricacies of data distributions. By carefully analyzing its value in conjunction with other descriptive statistics and understanding its limitations, researchers and analysts can extract more meaningful insights and make more informed decisions in various fields. The careful interpretation of kurtosis, combined with a broader understanding of data characteristics, is a cornerstone of robust statistical analysis.
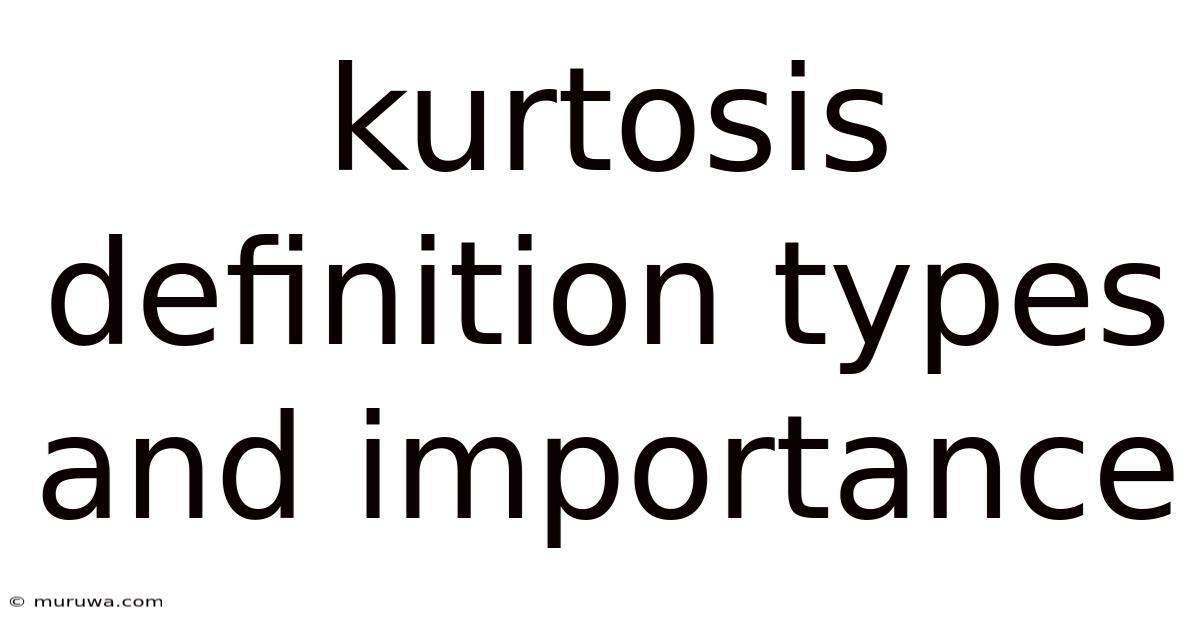
Thank you for visiting our website wich cover about Kurtosis Definition Types And Importance. We hope the information provided has been useful to you. Feel free to contact us if you have any questions or need further assistance. See you next time and dont miss to bookmark.
Also read the following articles
Article Title | Date |
---|---|
How To Check My Childs Credit Report | Apr 23, 2025 |
Leading Indicators Definition And How Theyre Used By Investors | Apr 23, 2025 |
K Definition | Apr 23, 2025 |
Low Volume Pullback Definition As Indicator And How It Works | Apr 23, 2025 |
Busted Convertible Security Definition | Apr 23, 2025 |