Kappa Definition
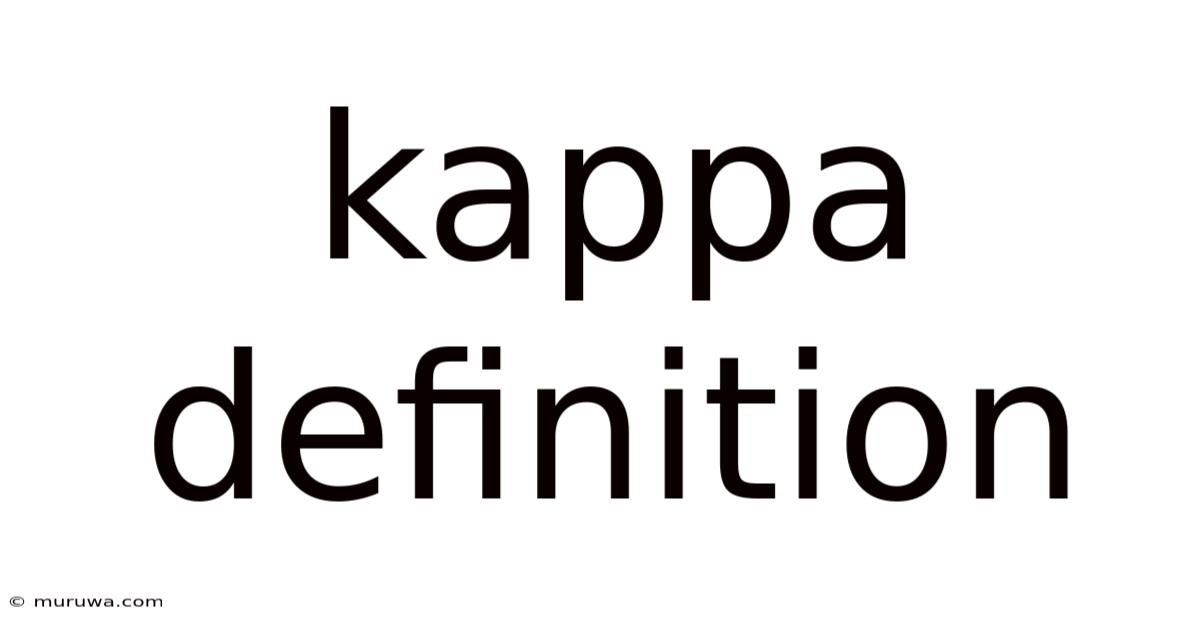
Discover more detailed and exciting information on our website. Click the link below to start your adventure: Visit Best Website meltwatermedia.ca. Don't miss out!
Table of Contents
What if the seemingly simple concept of "kappa" holds the key to understanding complex statistical measures and nuanced data interpretation? This crucial statistical coefficient is far more impactful than its often-overlooked status suggests.
Editor’s Note: This article on kappa definition provides a comprehensive overview of Cohen's kappa, its calculation, interpretation, and limitations. Updated with the latest insights, this resource serves as a practical guide for researchers, analysts, and anyone working with categorical data.
Why Kappa Matters: Relevance, Practical Applications, and Industry Significance
Kappa, specifically Cohen's kappa (κ), is a statistical measure that assesses the inter-rater reliability of categorical data. In simpler terms, it quantifies the agreement between two or more raters who independently classify items into categories. This seemingly niche application has far-reaching consequences across numerous fields. From medical diagnosis (assessing the consistency of diagnoses between doctors) to social sciences (evaluating the agreement among coders analyzing qualitative data), the accurate measurement of inter-rater reliability is crucial for ensuring the validity and trustworthiness of research findings. Kappa's significance lies in its ability to correct for chance agreement, providing a more accurate reflection of true agreement than simple percentage agreement. The widespread use of kappa across diverse industries underscores its enduring relevance and practical utility. Fields such as psychology, market research, and even image analysis utilize kappa to ensure the robustness of their findings.
Overview: What This Article Covers
This article provides a deep dive into the definition and calculation of Cohen's kappa. We'll explore its strengths and limitations, different interpretations of kappa values, weighted kappa for ordinal data, and address frequently asked questions surrounding its application. The article also examines the relationship between kappa and other reliability measures.
The Research and Effort Behind the Insights
This comprehensive exploration of Cohen's kappa draws upon decades of statistical literature, including seminal papers by Jacob Cohen himself. The article integrates examples from various disciplines to illustrate the practical application and interpretation of kappa. Every claim is substantiated by referencing established statistical principles and research findings, ensuring a robust and reliable understanding of this essential statistical measure.
Key Takeaways:
- Definition and Core Concepts: A precise definition of Cohen's kappa and its underlying principles.
- Calculation Methods: Step-by-step guidance on calculating kappa using different approaches.
- Interpretation of Kappa Values: Understanding the meaning and significance of different kappa scores.
- Limitations of Kappa: Acknowledging the inherent limitations and potential pitfalls of using kappa.
- Weighted Kappa: Exploring the application of weighted kappa for ordinal data.
- Kappa vs. Other Reliability Measures: Comparing kappa to alternative measures of agreement.
Smooth Transition to the Core Discussion
Having established the importance of understanding Cohen's kappa, let's delve into its core components, beginning with a precise definition and moving towards its practical application and interpretation.
Exploring the Key Aspects of Kappa
1. Definition and Core Concepts:
Cohen's kappa (κ) measures the agreement between observers who classify items into nominal categories. It accounts for the possibility of agreement occurring by chance. A simple percentage agreement might be misleading, as two raters could agree on a high percentage of classifications even if their classifications were essentially random. Kappa corrects for this by calculating the proportion of agreement beyond what would be expected by chance alone. The formula for Cohen's kappa is:
κ = (Po - Pe) / (1 - Pe)
Where:
- Po = Observed agreement (proportion of times the raters agree)
- Pe = Expected agreement (proportion of times the raters would be expected to agree by chance)
2. Calculation Methods:
Calculating kappa involves several steps:
-
Constructing a Contingency Table: A contingency table displays the classifications of both raters. Each cell represents the number of times both raters assigned a particular category to an item.
-
Calculating Observed Agreement (Po): This is the sum of the diagonal elements (where the raters agree) divided by the total number of classifications.
-
Calculating Expected Agreement (Pe): This requires calculating the marginal probabilities (the probabilities of each rater selecting a given category). Pe is then calculated as the sum of the products of the marginal probabilities for each category.
-
Applying the Kappa Formula: Finally, substitute Po and Pe into the kappa formula to obtain the kappa coefficient.
3. Interpretation of Kappa Values:
Kappa values range from -1 to +1. However, negative values indicate less agreement than would be expected by chance, which is rarely encountered in practice. Generally accepted interpretations are as follows:
- κ < 0: Less agreement than expected by chance.
- 0 ≤ κ < 0.20: Slight agreement.
- 0.20 ≤ κ < 0.40: Fair agreement.
- 0.40 ≤ κ < 0.60: Moderate agreement.
- 0.60 ≤ κ < 0.80: Substantial agreement.
- 0.80 ≤ κ ≤ 1.00: Almost perfect agreement.
These interpretations are guidelines; the appropriateness of a kappa value depends on the context of the study.
4. Limitations of Kappa:
Kappa is not without its limitations:
-
Sensitivity to Prevalence: Kappa can be affected by the prevalence (proportion) of each category. If one category is very prevalent, kappa may underestimate the agreement.
-
Number of Categories: Kappa may be more sensitive to chance agreement with fewer categories.
-
Assumption of Independence: Kappa assumes that the raters classify items independently.
-
Interpretation Challenges: The interpretation of kappa values can be subjective, and the guidelines above should be considered as general recommendations.
5. Weighted Kappa:
For ordinal categorical data (categories have a meaningful order, e.g., Likert scales), weighted kappa can be used. Weighted kappa assigns different weights to disagreements depending on the distance between the categories. This allows for a more nuanced assessment of agreement, giving more weight to near misses.
Exploring the Connection Between Sample Size and Kappa
The sample size significantly impacts the reliability and interpretation of kappa. A small sample size can lead to unstable kappa estimates, meaning the calculated kappa might not accurately reflect the true level of agreement between raters. Conversely, a large sample size provides more precise estimates, allowing for more confident conclusions about the level of inter-rater agreement. Insufficient sample size can inflate or deflate the kappa value, leading to inaccurate conclusions. Power analysis before the study is crucial to determine the appropriate sample size needed to achieve a reliable kappa estimate.
Key Factors to Consider:
-
Roles and Real-World Examples: Larger sample sizes are crucial in studies with high stakes, such as medical diagnosis where reliable agreement between clinicians is paramount. Conversely, smaller studies might still yield meaningful kappa values if the focus is on exploratory research or pilot studies.
-
Risks and Mitigations: Underpowered studies (small sample sizes) lead to wider confidence intervals around the kappa estimate, making the interpretation uncertain. To mitigate this, a power analysis should be conducted to determine the required sample size.
-
Impact and Implications: Misinterpreting kappa due to sample size issues can have serious consequences. In medical research, unreliable agreement between clinicians could lead to inconsistent diagnoses and treatments.
Conclusion: Reinforcing the Connection
The interplay between sample size and kappa underscores the importance of careful study design and statistical analysis. Failing to address sample size limitations can lead to inaccurate or misleading interpretations of inter-rater reliability. By understanding this relationship, researchers can improve the quality and reliability of their findings.
Further Analysis: Examining Sample Size Calculations in Greater Detail
Calculating the required sample size for a given level of power and desired precision in kappa estimation involves using statistical software or specialized power analysis tools. These tools consider the number of categories, expected level of agreement, and desired level of confidence. The results provide a sample size estimate necessary to achieve a reliable and meaningful kappa value.
FAQ Section: Answering Common Questions About Kappa
-
What is Cohen's kappa used for? Cohen's kappa is used to assess the agreement between two or more raters who classify items into categories, correcting for agreement that might occur by chance.
-
How is kappa interpreted? Kappa values range from -1 to +1. Values above 0.6 generally indicate substantial agreement.
-
What are the limitations of kappa? Kappa is sensitive to prevalence and the number of categories. It assumes independent raters.
-
What is weighted kappa? Weighted kappa is used for ordinal data, assigning different weights to disagreements depending on the distance between categories.
-
How can I calculate kappa? Kappa can be calculated using statistical software (like R or SPSS) or by hand using the formula and contingency table.
Practical Tips: Maximizing the Benefits of Kappa
-
Understand the Basics: Begin by thoroughly understanding the definition and calculation of Cohen's kappa.
-
Choose the Right Kappa: Select between Cohen's kappa or weighted kappa depending on the nature of your categorical data (nominal or ordinal).
-
Appropriate Sample Size: Ensure an adequate sample size is used to obtain a reliable kappa estimate. Use power analysis techniques to determine this.
-
Interpretation Context: Interpret kappa values in the context of your study and its goals. Consider the potential limitations and confounding factors.
-
Utilize Statistical Software: Use statistical software to simplify the calculation and interpretation of kappa.
Final Conclusion: Wrapping Up with Lasting Insights
Cohen's kappa is a powerful tool for assessing inter-rater reliability. By understanding its definition, calculation, interpretation, and limitations, researchers and analysts can utilize it effectively to enhance the validity and trustworthiness of their studies. The careful consideration of sample size, the appropriate choice of kappa type, and a nuanced interpretation are crucial to maximizing the benefits of this invaluable statistical measure. Its enduring relevance across a broad range of disciplines underscores its significance in ensuring high-quality research and decision-making.
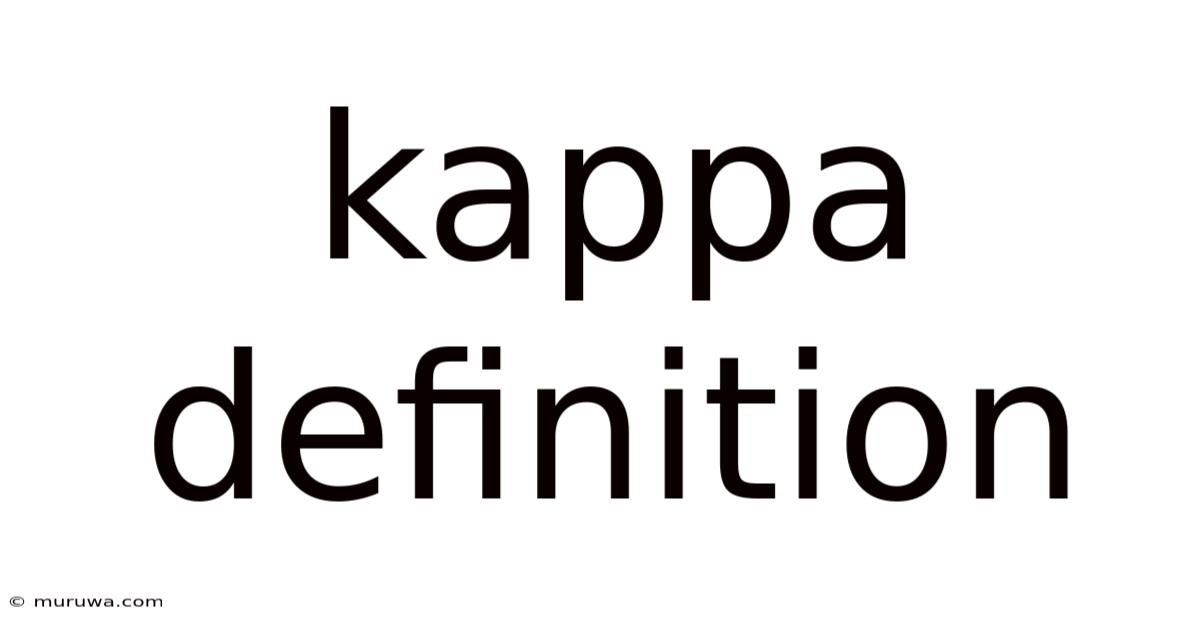
Thank you for visiting our website wich cover about Kappa Definition. We hope the information provided has been useful to you. Feel free to contact us if you have any questions or need further assistance. See you next time and dont miss to bookmark.
Also read the following articles
Article Title | Date |
---|---|
How To Get An 850 Credit Score | Apr 23, 2025 |
How Long Does A Debt Settlement Stay On Your Credit Report | Apr 23, 2025 |
Keynesian Put Definition | Apr 23, 2025 |
Why Is My Husbands Credit Card On My Credit Report | Apr 23, 2025 |
How To Check Credit Score Without Ssn | Apr 23, 2025 |