Joint Probability Definition Formula And Example
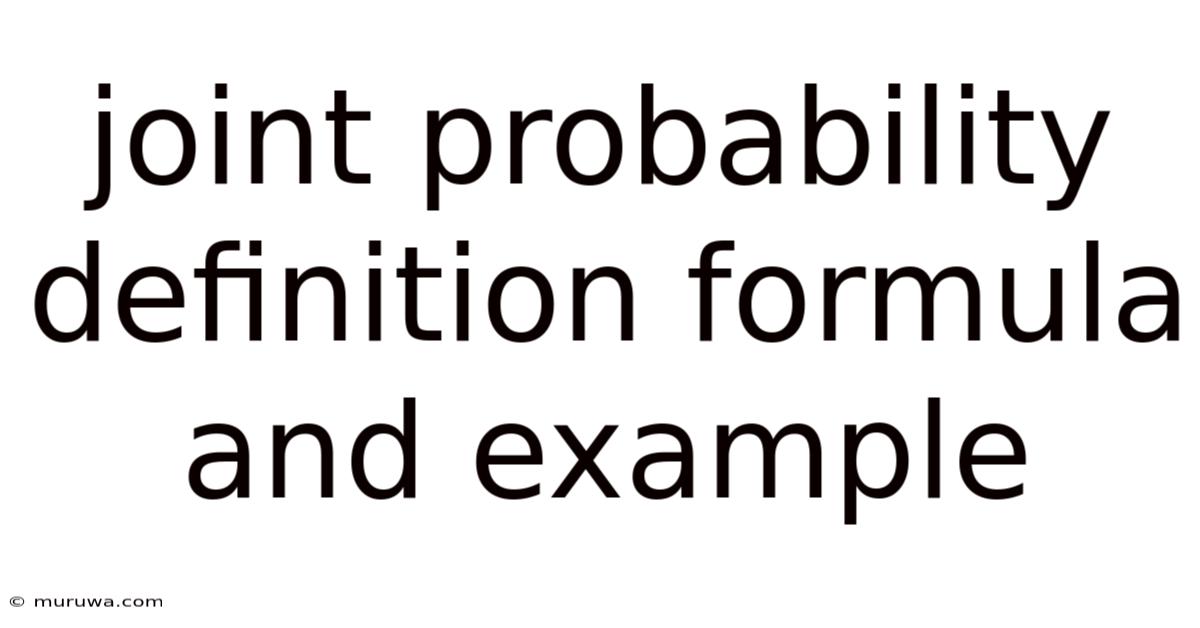
Discover more detailed and exciting information on our website. Click the link below to start your adventure: Visit Best Website meltwatermedia.ca. Don't miss out!
Table of Contents
Understanding Joint Probability: Definition, Formula, and Examples
What if the seemingly complex world of probabilities could be simplified by understanding joint probability? This fundamental concept unlocks the ability to analyze interconnected events and make more informed decisions in various fields, from finance to healthcare.
Editor’s Note: This article on joint probability, its definition, formula, and examples, was published today, providing readers with up-to-date information and insights into this crucial statistical concept.
Why Joint Probability Matters:
Joint probability is a cornerstone of probability theory, offering a powerful tool to analyze the likelihood of two or more events occurring simultaneously. Its relevance spans numerous fields:
- Finance: Assessing the risk of multiple financial instruments failing concurrently.
- Healthcare: Determining the probability of a patient having multiple conditions based on symptoms.
- Engineering: Evaluating the reliability of systems with multiple interdependent components.
- Machine Learning: Understanding the relationships between different variables in datasets.
The ability to quantify the likelihood of multiple events occurring together empowers better prediction, risk management, and decision-making.
Overview: What This Article Covers:
This article will comprehensively explore joint probability, covering its definition, the formula for calculating it, various scenarios (independent vs. dependent events), and numerous illustrative examples. We will also delve into conditional probability and its relationship with joint probability, addressing common misconceptions and providing a solid foundation for understanding this vital concept.
The Research and Effort Behind the Insights:
This article draws upon established probability theory principles and incorporates numerous real-world examples to enhance understanding. Each claim is supported by mathematical definitions and illustrative scenarios, ensuring accuracy and clarity for the reader. The structured approach aims to provide a clear and accessible understanding of joint probability.
Key Takeaways:
- Definition and Core Concepts: A precise definition of joint probability and its fundamental principles.
- Formula and Calculation: Understanding the formula and step-by-step methods for calculating joint probability.
- Independent vs. Dependent Events: Distinguishing between these scenarios and their impact on calculations.
- Conditional Probability: Exploring the relationship between joint and conditional probability.
- Real-World Applications: Illustrative examples from various fields showcasing practical applications.
Smooth Transition to the Core Discussion:
Having established the importance and scope of joint probability, let's now delve into its core aspects.
Exploring the Key Aspects of Joint Probability:
1. Definition and Core Concepts:
Joint probability refers to the probability of two or more events occurring together. It's a measure of the likelihood of a specific combination of outcomes from multiple events. For instance, what's the probability of rolling a 6 on a die and flipping heads on a coin? This is a joint probability question. We denote the joint probability of events A and B as P(A and B) or P(A ∩ B).
2. Formula and Calculation:
The formula for calculating joint probability depends on whether the events are independent or dependent.
-
Independent Events: If events A and B are independent (the occurrence of one doesn't affect the probability of the other), the joint probability is simply the product of their individual probabilities:
P(A ∩ B) = P(A) * P(B)
-
Dependent Events: If events A and B are dependent, the joint probability is calculated using conditional probability:
P(A ∩ B) = P(A|B) * P(B) or P(A ∩ B) = P(B|A) * P(A)
Where P(A|B) represents the conditional probability of A given B has already occurred (and vice-versa for P(B|A)).
3. Independent vs. Dependent Events:
Understanding the distinction is crucial.
- Independent Events: The outcome of one event doesn't influence the outcome of the other. Example: Rolling a die and flipping a coin are independent events.
- Dependent Events: The outcome of one event affects the probability of the other. Example: Drawing two cards from a deck without replacement. The probability of the second card depends on the first card drawn.
4. Conditional Probability and its Relationship with Joint Probability:
Conditional probability, P(A|B), is the probability of event A occurring given that event B has already occurred. The relationship between joint and conditional probability is fundamental:
P(A|B) = P(A ∩ B) / P(B) and P(B|A) = P(A ∩ B) / P(A)
5. Real-World Applications and Examples:
Let's illustrate with several examples:
Example 1: Independent Events (Dice and Coin)
What is the probability of rolling a 6 on a fair six-sided die and flipping heads on a fair coin?
- P(rolling a 6) = 1/6
- P(flipping heads) = 1/2
- Since the events are independent: P(6 and heads) = (1/6) * (1/2) = 1/12
Example 2: Dependent Events (Cards)
What is the probability of drawing two aces from a standard deck of 52 cards without replacement?
- P(first ace) = 4/52
- P(second ace | first ace) = 3/51 (since one ace has already been drawn)
- P(two aces) = (4/52) * (3/51) = 1/221
Example 3: Dependent Events (Medical Diagnosis)
Suppose a test for a particular disease has a 90% accuracy rate (true positive) and a 5% false positive rate. If 1% of the population has the disease, what is the probability that a randomly selected person who tests positive actually has the disease?
Let:
- D = event that a person has the disease
- T = event that a person tests positive
We are given:
- P(D) = 0.01
- P(T|D) = 0.90 (true positive rate)
- P(T|¬D) = 0.05 (false positive rate)
We want to find P(D|T). Using Bayes' theorem (derived from conditional probability and joint probability):
P(D|T) = [P(T|D) * P(D)] / [P(T|D) * P(D) + P(T|¬D) * P(¬D)]
P(¬D) = 1 - P(D) = 0.99
P(D|T) = [0.90 * 0.01] / [0.90 * 0.01 + 0.05 * 0.99] ≈ 0.1538
This means that only about 15.38% of people who test positive actually have the disease. This highlights the importance of considering both the true positive and false positive rates in medical diagnosis.
Exploring the Connection Between Sample Space and Joint Probability:
The sample space, the set of all possible outcomes, is crucial in understanding joint probability. For independent events, the joint probability is simply calculated by multiplying individual probabilities. However, for dependent events, the sample space changes after each event, leading to conditional probabilities that must be considered.
Key Factors to Consider:
- Roles and Real-World Examples: As demonstrated above, the application of joint probability varies vastly depending on the context. In finance, it might involve portfolio risk assessment; in healthcare, it’s crucial for disease diagnosis; and in engineering, it's essential for system reliability analysis.
- Risks and Mitigations: Misinterpreting the independence or dependence of events can lead to inaccurate calculations and poor decision-making. Careful consideration of the events and their relationship is crucial.
- Impact and Implications: Accurate joint probability calculations significantly improve predictive models and risk management strategies across numerous fields. Failing to account for joint probabilities can lead to substantial errors.
Conclusion: Reinforcing the Connection:
The sample space and the nature of the events (independent or dependent) are fundamental to accurately computing joint probabilities. The examples demonstrate the versatility and power of joint probability in analyzing and understanding interconnected events.
Further Analysis: Examining Conditional Probability in Greater Detail:
Conditional probability is inextricably linked to joint probability. Understanding conditional probability helps clarify situations where events are dependent. Bayes' theorem, built upon conditional probability, provides a powerful tool to update probabilities based on new evidence. This is crucial in many applications, such as medical diagnosis, spam filtering, and risk assessment.
FAQ Section: Answering Common Questions About Joint Probability:
-
What is joint probability? Joint probability is the probability of two or more events occurring together.
-
How does joint probability differ for independent and dependent events? For independent events, it's the product of individual probabilities. For dependent events, it involves conditional probabilities.
-
What is conditional probability and how does it relate to joint probability? Conditional probability is the probability of an event given another event has already occurred. It's used to calculate joint probability for dependent events.
-
How is joint probability used in real-world applications? It's used extensively in finance, healthcare, engineering, and machine learning for risk assessment, prediction, and decision-making.
Practical Tips: Maximizing the Benefits of Understanding Joint Probability:
- Start with the basics: Clearly define the events and whether they are independent or dependent.
- Understand conditional probability: Master conditional probability to handle dependent events effectively.
- Use appropriate formulas: Employ the correct formula for independent or dependent events.
- Visualize the problem: Using diagrams (like Venn diagrams or tree diagrams) can improve understanding and accuracy.
Final Conclusion: Wrapping Up with Lasting Insights:
Joint probability is a powerful tool for understanding and quantifying the likelihood of multiple events occurring together. By mastering its concepts and applications, individuals and organizations can make more informed decisions in diverse fields, enhancing prediction accuracy and risk management capabilities. A thorough understanding of joint probability and its close connection to conditional probability is crucial for anyone working with data analysis, probability, or statistics.
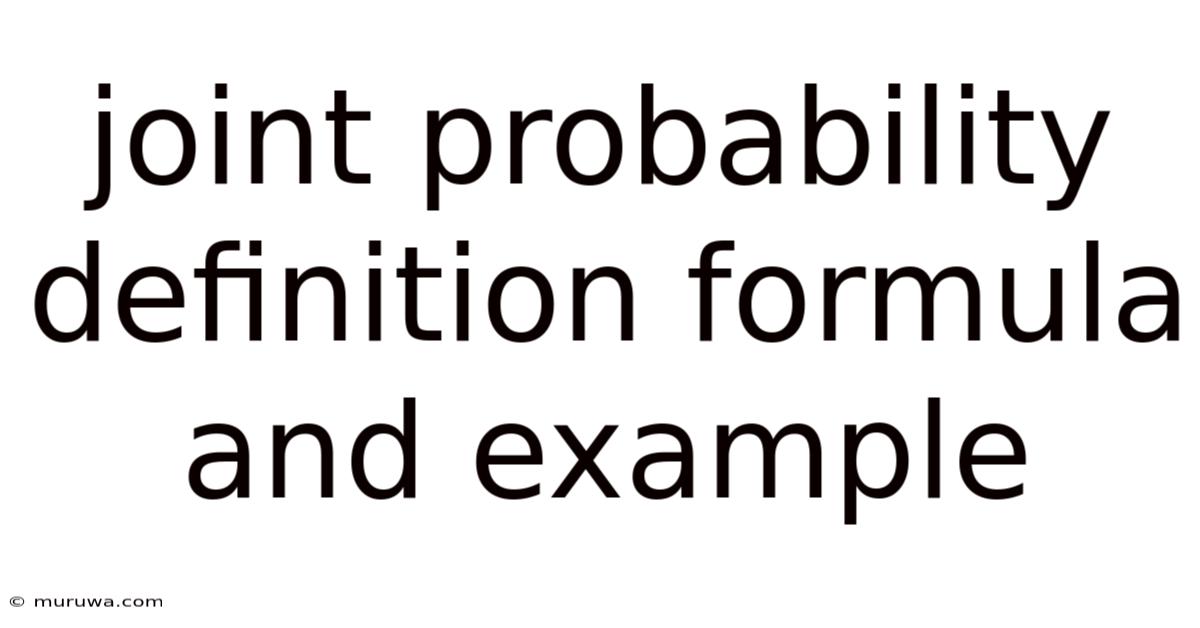
Thank you for visiting our website wich cover about Joint Probability Definition Formula And Example. We hope the information provided has been useful to you. Feel free to contact us if you have any questions or need further assistance. See you next time and dont miss to bookmark.
Also read the following articles
Article Title | Date |
---|---|
How Does A 20 Year Term Life Insurance Policy Work | Apr 28, 2025 |
How To Get Cash From Merrick Bank Credit Card Without Pin | Apr 28, 2025 |
How To Find Apple Credit Card Number | Apr 28, 2025 |
How To Respond To Irs Notice Cp11 | Apr 28, 2025 |
When Can I Use My Credit Card Again After Paying It Off | Apr 28, 2025 |