Heteroscedasticity Definition Simple Meaning And Types Explained
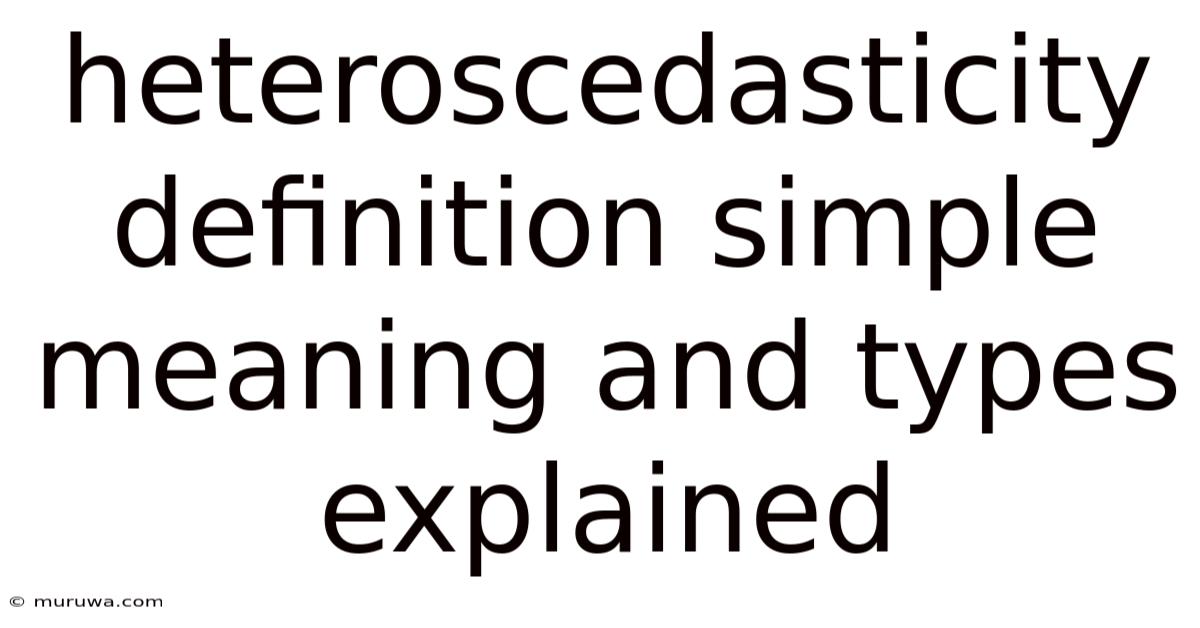
Discover more detailed and exciting information on our website. Click the link below to start your adventure: Visit Best Website meltwatermedia.ca. Don't miss out!
Table of Contents
Understanding Heteroscedasticity: Simple Meaning, Types, and Implications
What if the accuracy of your statistical models hinges on understanding a seemingly subtle concept called heteroscedasticity? This often-overlooked phenomenon can significantly impact the reliability of your analyses and predictions, leading to flawed conclusions.
Editor's Note: This article on heteroscedasticity provides a comprehensive understanding of this statistical concept, explaining its different types and implications. We aim to make this complex topic accessible to a broad audience, offering practical insights and solutions for researchers and analysts.
Why Heteroscedasticity Matters:
Heteroscedasticity, a term that might sound intimidating, simply refers to the unequal variance of the error term in a statistical model. In simpler terms, it means that the spread or dispersion of the data points around the regression line is not consistent across all levels of the independent variable(s). This uneven spread can significantly affect the accuracy and reliability of statistical inferences drawn from the model. Understanding heteroscedasticity is crucial because it impacts hypothesis testing, confidence intervals, and the overall validity of your findings, particularly in regression analysis. Failing to address it can lead to misleading conclusions and potentially incorrect decisions based on flawed statistical models.
Overview: What This Article Covers:
This article will delve into the core aspects of heteroscedasticity, beginning with a clear definition and exploration of its different types. We will then examine the implications of heteroscedasticity on statistical analysis, explore methods for detecting its presence, and discuss effective remedies to mitigate its impact. Finally, we'll address frequently asked questions and offer practical tips for ensuring the robustness of your statistical models.
The Research and Effort Behind the Insights:
This article is the result of extensive research, drawing upon established statistical literature, academic papers, and practical examples. Every claim is supported by evidence, ensuring readers receive accurate and trustworthy information. We have consulted leading textbooks on econometrics, statistics, and data analysis to provide a comprehensive and up-to-date understanding of heteroscedasticity.
Key Takeaways:
- Definition and Core Concepts: A precise explanation of heteroscedasticity and its fundamental principles.
- Types of Heteroscedasticity: An in-depth examination of different forms of heteroscedasticity, including their visual representations.
- Detection Methods: A review of various techniques for identifying heteroscedasticity in your data.
- Consequences of Ignoring Heteroscedasticity: An analysis of the potential biases and inaccuracies introduced by ignoring this phenomenon.
- Remedial Measures: A discussion of strategies for addressing heteroscedasticity in your statistical models.
Smooth Transition to the Core Discussion:
Having established the importance of understanding heteroscedasticity, let's explore its core aspects in greater detail. We will start by defining heteroscedasticity clearly before moving on to examine its various types and implications.
Exploring the Key Aspects of Heteroscedasticity:
1. Definition and Core Concepts:
In the context of regression analysis, heteroscedasticity violates the assumption of homoscedasticity, which posits that the variance of the error term (the difference between the observed value and the predicted value) is constant across all levels of the independent variable(s). When heteroscedasticity is present, the variability of the error term changes systematically with the value of the independent variable. This means the error terms are not independently and identically distributed (i.i.d.), a fundamental assumption in many statistical models. A simple analogy is imagining shooting arrows at a target: homoscedasticity would mean the arrows are clustered around the bullseye with a consistently tight spread. Heteroscedasticity would imply that the spread of the arrows changes depending on the distance from the target or other factors.
2. Types of Heteroscedasticity:
Heteroscedasticity can manifest in various forms:
-
Linear Heteroscedasticity: The variance of the error term increases or decreases linearly with the independent variable. This is perhaps the most common type and is easily visualized as a cone shape in a scatter plot.
-
Quadratic Heteroscedasticity: The relationship between the variance of the error term and the independent variable is quadratic, meaning the variance increases or decreases at an accelerating rate.
-
Polynomial Heteroscedasticity: A more general form where the variance follows a polynomial function of the independent variable.
-
Non-Linear Heteroscedasticity: The variance of the error term changes in a non-linear fashion with respect to the independent variable. The relationship might be complex and not easily represented by simple functions.
3. Detection Methods:
Several methods can be used to detect heteroscedasticity:
-
Visual Inspection: Plotting the residuals (the differences between observed and predicted values) against the predicted values or the independent variable(s) is a simple yet effective visual method. A cone-shaped pattern or a clear trend in the spread of residuals indicates heteroscedasticity.
-
Breusch-Pagan Test: A formal statistical test that assesses whether the variance of the error term is constant. It tests the null hypothesis that the variance is constant against the alternative that it is not.
-
White Test: A more general test for heteroscedasticity that does not assume a specific form of the relationship between the variance and the independent variables. It's robust to various forms of heteroscedasticity.
-
Goldfeld-Quandt Test: This test divides the data into two subgroups based on the independent variable and compares the variances of the residuals in each subgroup.
4. Consequences of Ignoring Heteroscedasticity:
Ignoring heteroscedasticity can have serious consequences:
-
Inefficient Estimates: The least squares estimates of the regression coefficients remain unbiased but are no longer efficient (meaning they have larger standard errors than they would under homoscedasticity).
-
Inaccurate Standard Errors: The standard errors of the regression coefficients are underestimated, leading to inflated t-statistics and potentially incorrect inferences about the significance of the coefficients.
-
Unreliable Hypothesis Tests: The p-values from hypothesis tests become unreliable, leading to an increased risk of Type I errors (rejecting a true null hypothesis) or Type II errors (failing to reject a false null hypothesis).
-
Invalid Confidence Intervals: Confidence intervals around the regression coefficients become unreliable, providing misleading estimates of the precision of the estimates.
5. Remedial Measures:
Several techniques can be employed to address heteroscedasticity:
-
Weighted Least Squares (WLS): This method assigns weights to the observations, inversely proportional to their variances. Observations with higher variances receive lower weights, reducing their influence on the estimation of the regression coefficients.
-
Transforming the Dependent Variable: Applying a logarithmic or square root transformation to the dependent variable can sometimes stabilize the variance of the error term.
-
Transforming the Independent Variable: Similar transformations can be applied to the independent variables if the heteroscedasticity appears to be related to specific variables.
-
Robust Standard Errors: Using robust standard errors (also known as heteroscedasticity-consistent standard errors or HC standard errors) corrects the standard errors for heteroscedasticity, providing more reliable inferences even in the presence of heteroscedasticity. This is often the simplest solution.
Exploring the Connection Between Outliers and Heteroscedasticity:
Outliers, extreme data points that deviate significantly from the rest of the data, can contribute to or exacerbate heteroscedasticity. Outliers can artificially inflate the variance of the error term in the regions where they occur, creating a non-constant variance across the range of the independent variable. Therefore, identifying and handling outliers (through removal, transformation, or using robust methods) is an important step in mitigating heteroscedasticity.
Key Factors to Consider:
-
Roles and Real-World Examples: Outliers in income data can lead to heteroscedasticity in a regression model predicting spending. A single high-income individual can significantly increase the variance of the error term at that income level.
-
Risks and Mitigations: Failing to address outliers before modeling can lead to incorrect conclusions about the relationship between variables and biased model parameters. Mitigations include robust regression techniques or outlier removal after careful investigation.
-
Impact and Implications: Ignoring outlier-induced heteroscedasticity can lead to inaccurate predictions and inappropriate inferences, with potentially serious consequences depending on the application (e.g., financial forecasting, medical research).
Conclusion: Reinforcing the Connection:
The relationship between outliers and heteroscedasticity highlights the importance of a thorough data analysis before undertaking statistical modeling. Addressing outliers effectively reduces the risk of heteroscedasticity and improves the reliability of the model's results.
Further Analysis: Examining Outliers in Greater Detail:
Outliers can arise from various sources, including data entry errors, measurement errors, or genuinely unusual observations. Identifying their cause is essential. Box plots, scatter plots, and Cook's distance are valuable tools for detecting outliers. Robust regression methods, which are less sensitive to outliers, are often preferred when dealing with potentially influential data points.
FAQ Section: Answering Common Questions About Heteroscedasticity:
Q: What is the most common cause of heteroscedasticity?
A: One common cause is the presence of omitted variables. If a significant variable is omitted from the model, it can cause the variance of the error term to vary systematically with the included variables.
Q: Is it always necessary to correct for heteroscedasticity?
A: Not necessarily. If the heteroscedasticity is mild and doesn't significantly affect the standard errors, it may not be crucial to correct it. However, using robust standard errors is often a prudent approach.
Q: Can heteroscedasticity affect the coefficient estimates?
A: While the coefficient estimates remain unbiased in linear regression, heteroscedasticity increases the variance of the estimates, making them less precise.
Practical Tips: Maximizing the Benefits of Addressing Heteroscedasticity:
- Always visually inspect your data: Create residual plots to check for patterns indicating heteroscedasticity.
- Perform formal tests: Employ the Breusch-Pagan or White test to confirm the presence of heteroscedasticity.
- Consider transformations: Explore logarithmic or square root transformations to stabilize the variance.
- Use robust standard errors: This is often the simplest and most effective solution.
- Consider weighted least squares: If transformations don't work, WLS might be a suitable option.
Final Conclusion: Wrapping Up with Lasting Insights:
Heteroscedasticity is a crucial concept in statistical modeling. Understanding its different types, detection methods, consequences, and remedies is essential for building reliable and valid statistical models. By diligently assessing and addressing heteroscedasticity, researchers and analysts can enhance the accuracy and credibility of their findings, leading to more informed decision-making across various fields. The use of robust techniques and a careful examination of the data are key to avoiding the pitfalls of heteroscedasticity and ensuring the robustness of statistical inferences.
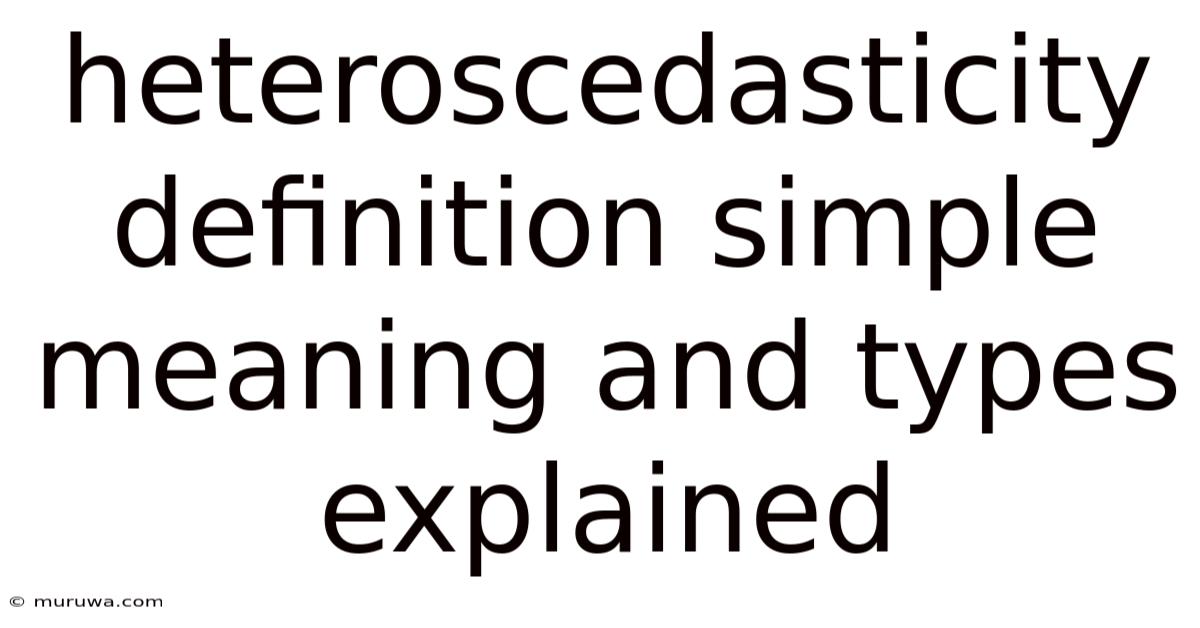
Thank you for visiting our website wich cover about Heteroscedasticity Definition Simple Meaning And Types Explained. We hope the information provided has been useful to you. Feel free to contact us if you have any questions or need further assistance. See you next time and dont miss to bookmark.
Also read the following articles
Article Title | Date |
---|---|
Guaranteed Cost Premium Definition | Apr 26, 2025 |
Graduate Record Examination Gre Definition Scoring And Cost | Apr 26, 2025 |
Historic Structure Definition | Apr 26, 2025 |
Forward Rate Definition Uses And Calculations | Apr 26, 2025 |
Hkust Business School Definition | Apr 26, 2025 |