Garch Model Definition And Uses In Statistics
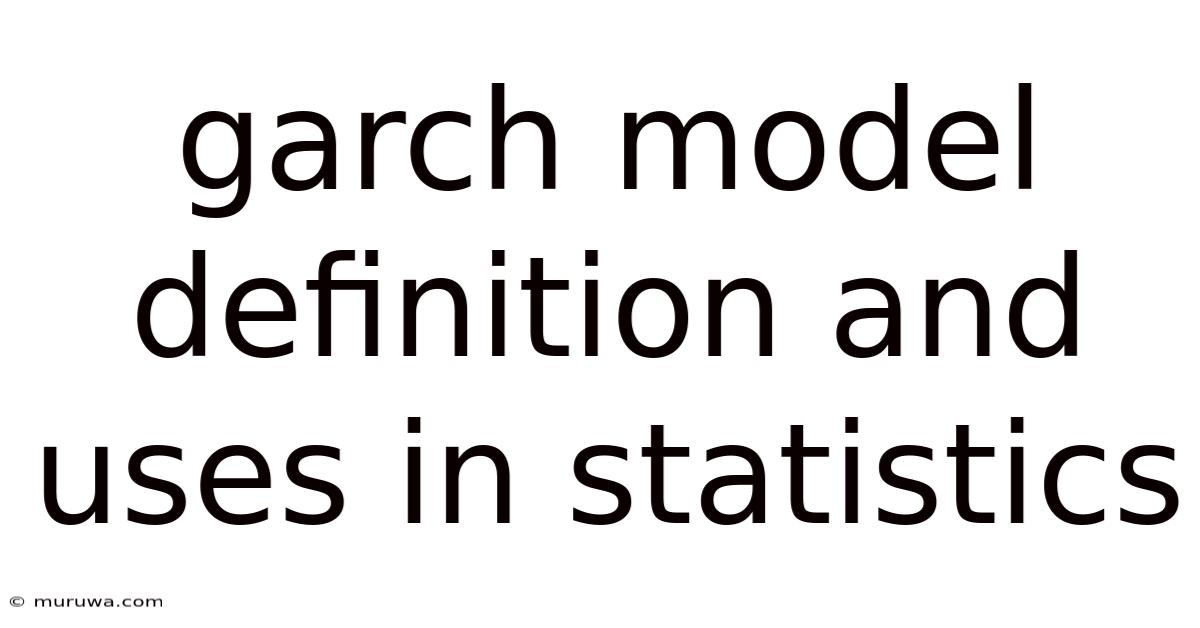
Discover more detailed and exciting information on our website. Click the link below to start your adventure: Visit Best Website meltwatermedia.ca. Don't miss out!
Table of Contents
Unveiling the Power of GARCH Models: Definition and Applications in Statistics
What if accurate prediction of financial market volatility were readily available? Generalized Autoregressive Conditional Heteroskedasticity (GARCH) models offer a powerful statistical framework for achieving precisely that, revolutionizing risk management and forecasting in various fields.
Editor’s Note: This in-depth exploration of GARCH models is designed to provide readers with a comprehensive understanding of their definition, applications, and implications. This article has been compiled using the latest research and data, offering readers current, actionable insights.
Why GARCH Models Matter: Relevance, Practical Applications, and Industry Significance
GARCH models hold immense significance in fields grappling with time-series data exhibiting volatility clustering – periods of high volatility followed by periods of relative calm, a characteristic prevalent in financial markets, weather patterns, and even social media activity. Their ability to capture and forecast this dynamic volatility makes them invaluable tools for:
- Risk Management: Accurate volatility forecasting is crucial for effective risk management in finance, enabling better portfolio optimization, hedging strategies, and options pricing.
- Trading Strategies: GARCH models inform trading algorithms, providing insights into optimal entry and exit points based on predicted volatility levels.
- Economic Forecasting: Understanding volatility in macroeconomic indicators like inflation or GDP growth is vital for policymakers and economists.
- Weather Forecasting: GARCH models can improve the prediction of extreme weather events by analyzing the changing volatility of weather patterns.
- Social Science Research: Analyzing the fluctuating intensity of social phenomena, like social media trends or crime rates, benefits from GARCH modeling.
Overview: What This Article Covers
This article provides a detailed exploration of GARCH models, starting with fundamental definitions and progressing to advanced applications. Readers will gain a practical understanding of:
- The core concepts of ARCH and GARCH models.
- The mathematical foundation of GARCH models.
- Different variants of GARCH models and their respective applications.
- Model diagnostics and evaluation techniques.
- Real-world applications and case studies across various industries.
- The limitations and challenges associated with GARCH models.
The Research and Effort Behind the Insights
This article is the culmination of extensive research, drawing upon academic papers, industry reports, and practical applications of GARCH models. The information presented is supported by empirical evidence and aims to provide readers with accurate, reliable, and actionable insights. The structured approach ensures a clear understanding of complex concepts, making this resource suitable for both beginners and experienced analysts.
Key Takeaways:
- Definition and Core Concepts: A clear explanation of ARCH and GARCH models, their underlying assumptions, and their mathematical representation.
- Model Estimation: Detailed steps involved in estimating GARCH model parameters.
- Model Diagnostics: Methods for assessing the goodness-of-fit and validity of estimated GARCH models.
- Applications and Case Studies: Real-world examples demonstrating the practical use of GARCH models across different sectors.
- Advanced GARCH Models: An exploration of extensions and variations of the basic GARCH model.
- Limitations and Future Directions: An honest appraisal of the limitations and areas of ongoing research in GARCH modeling.
Smooth Transition to the Core Discussion:
Having established the importance of GARCH models, let’s delve into their mathematical foundations and practical applications. We will begin by examining the simpler ARCH model before progressing to the more widely used GARCH model.
Exploring the Key Aspects of GARCH Models
1. ARCH (Autoregressive Conditional Heteroskedasticity) Models:
The ARCH model, proposed by Robert Engle in 1982, is a foundational model for understanding conditional heteroskedasticity. It posits that the variance of the error term in a time-series model is not constant but depends on the past squared error terms. The basic ARCH(p) model is defined as:
σₜ² = α₀ + α₁εₜ₋₁² + α₂εₜ₋₂² + ... + αₚεₜ₋ₚ²
Where:
σₜ²
is the conditional variance of the error term at time t.εₜ
is the error term at time t.α₀
,α₁
, ...,αₚ
are parameters to be estimated.
ARCH models capture the volatility clustering effect, but they often require a high order (p) to adequately capture the persistence of volatility. This can lead to computational difficulties and potential issues with model stability.
2. GARCH (Generalized Autoregressive Conditional Heteroskedasticity) Models:
The GARCH model, a generalization of the ARCH model, addresses the limitations of the ARCH model by including past conditional variances in the equation for the conditional variance. The basic GARCH(p,q) model is defined as:
σₜ² = α₀ + α₁εₜ₋₁² + ... + αₚεₜ₋ₚ² + β₁σₜ₋₁² + ... + β꜀σₜ₋꜀²
Where:
β₁
, ...,β꜀
are parameters representing the autoregressive components of the conditional variance.
The GARCH(1,1) model is the most commonly used variant, offering a good balance between model complexity and explanatory power:
σₜ² = α₀ + α₁εₜ₋₁² + β₁σₜ₋₁²
The parameters in the GARCH(1,1) model must satisfy certain conditions for the model to be stationary and ensure positive conditional variance: α₀ > 0
, α₁ ≥ 0
, β₁ ≥ 0
, and α₁ + β₁ < 1
.
3. Model Estimation:
GARCH model parameters are typically estimated using maximum likelihood estimation (MLE). This involves finding the parameter values that maximize the likelihood of observing the actual data given the model specification. Specialized software packages like R, Python (with libraries like statsmodels), and EViews are commonly used for GARCH model estimation.
4. Model Diagnostics:
After estimating a GARCH model, it's crucial to assess its goodness-of-fit and validity. This involves:
- Residual Analysis: Examining the standardized residuals for autocorrelation and normality.
- ARCH-LM Test: Testing for the presence of remaining ARCH effects in the residuals.
- Information Criteria: Using criteria like AIC (Akaike Information Criterion) and BIC (Bayesian Information Criterion) to compare different GARCH models.
5. Applications Across Industries:
GARCH models find widespread applications in various fields:
- Finance: Forecasting volatility of stock prices, exchange rates, and other financial assets. Value at Risk (VaR) calculations rely heavily on GARCH models.
- Economics: Modeling the volatility of macroeconomic variables like inflation and GDP growth.
- Energy: Predicting the volatility of energy prices, crucial for risk management in the energy sector.
- Insurance: Assessing the risk associated with insurance claims.
6. Impact on Innovation:
GARCH models continue to evolve with advancements like:
- EGARCH (Exponential GARCH): Allows for asymmetric effects, where positive and negative shocks have different impacts on volatility.
- GJR-GARCH (Glosten, Jagannathan, and Runkle GARCH): Similar to EGARCH but with a simpler specification.
- TGARCH (Threshold GARCH): Captures asymmetric effects based on thresholds.
Closing Insights: Summarizing the Core Discussion
GARCH models are essential tools for analyzing and forecasting volatility in time-series data. Their versatility and ability to capture the dynamic nature of volatility make them indispensable in diverse fields. Understanding the underlying principles, estimation techniques, and diagnostic procedures is crucial for effective application.
Exploring the Connection Between Volatility Clustering and GARCH Models
Volatility clustering, the phenomenon where periods of high volatility are followed by periods of high volatility and similarly for low volatility, is a key characteristic driving the need for GARCH models. The inherent autoregressive nature of GARCH models directly addresses this clustering by explicitly modeling the dependence of current volatility on past volatility and past squared innovations.
Key Factors to Consider:
- Roles and Real-World Examples: Volatility clustering is evident in financial markets, where periods of market crashes or booms are followed by extended periods of similar activity. This is precisely what GARCH models are designed to capture and predict.
- Risks and Mitigations: Failure to account for volatility clustering can lead to inaccurate risk assessments and flawed investment strategies. Proper model selection and thorough diagnostics are crucial to mitigate these risks.
- Impact and Implications: Accurate forecasting of volatility through GARCH models significantly impacts portfolio optimization, risk management, and option pricing, contributing to more efficient and stable financial markets.
Conclusion: Reinforcing the Connection
The intimate relationship between volatility clustering and GARCH models is undeniable. GARCH models provide a robust framework for analyzing and forecasting this crucial aspect of many time series, leading to improved decision-making across various sectors.
Further Analysis: Examining Volatility Clustering in Greater Detail
Volatility clustering is not merely a statistical anomaly; it reflects the inherent dynamics of many systems. In financial markets, it can be attributed to factors such as market sentiment, news events, and herding behavior. Understanding the underlying drivers of volatility clustering is crucial for developing more accurate and reliable GARCH models.
FAQ Section: Answering Common Questions About GARCH Models
- What is a GARCH model? A GARCH model is a statistical model used to model the volatility of time series data, particularly those exhibiting volatility clustering.
- How is a GARCH model different from an ARCH model? GARCH models extend ARCH models by including past conditional variances in addition to past squared errors in the volatility equation, leading to more robust and flexible modeling of volatility.
- What are the assumptions of a GARCH model? Key assumptions include conditional normality of errors and the absence of autocorrelation in the standardized residuals.
- What are some limitations of GARCH models? GARCH models can be computationally intensive, particularly for higher-order models. They may also struggle with highly non-linear relationships or structural breaks in the data.
- What software can I use to estimate a GARCH model? Popular choices include R, Python (statsmodels), EViews, and MATLAB.
Practical Tips: Maximizing the Benefits of GARCH Models
- Understand the Data: Before applying a GARCH model, thoroughly analyze the data for stationarity, potential outliers, and the presence of volatility clustering.
- Model Selection: Carefully choose the appropriate GARCH model (e.g., GARCH(1,1), EGARCH, GJR-GARCH) based on the data characteristics and the research question.
- Diagnostics: Conduct thorough diagnostic checks to ensure the model is a good fit for the data.
- Interpretation: Interpret the estimated parameters cautiously, focusing on their economic implications rather than just statistical significance.
- Out-of-Sample Testing: Always test the model's predictive accuracy on out-of-sample data to assess its robustness and generalizability.
Final Conclusion: Wrapping Up with Lasting Insights
GARCH models have revolutionized the way we understand and forecast volatility. Their applications extend far beyond finance, offering a powerful tool for analyzing dynamic systems across various domains. By understanding their strengths and limitations, researchers and practitioners can leverage the power of GARCH models to make better-informed decisions and navigate uncertainty more effectively. Continued research and development will undoubtedly lead to further refinements and advancements in this vital area of statistical modeling.
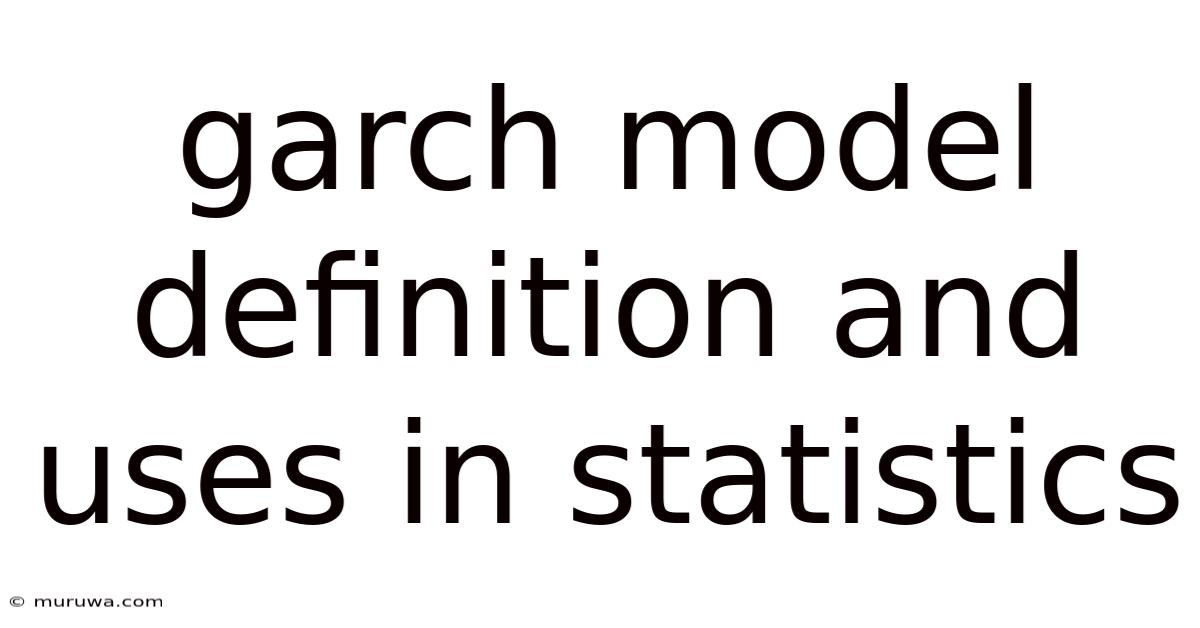
Thank you for visiting our website wich cover about Garch Model Definition And Uses In Statistics. We hope the information provided has been useful to you. Feel free to contact us if you have any questions or need further assistance. See you next time and dont miss to bookmark.
Also read the following articles
Article Title | Date |
---|---|
General Examination Definition | Apr 26, 2025 |
Functional Decomposition Definition Diagrams And Applications | Apr 26, 2025 |
What A Forfeited Share Means With Definition And Example | Apr 26, 2025 |
Grandfathered Bond Definition | Apr 26, 2025 |
Forwardation Definition | Apr 26, 2025 |