Frequency Severity Method Definition And How Insurers Use It
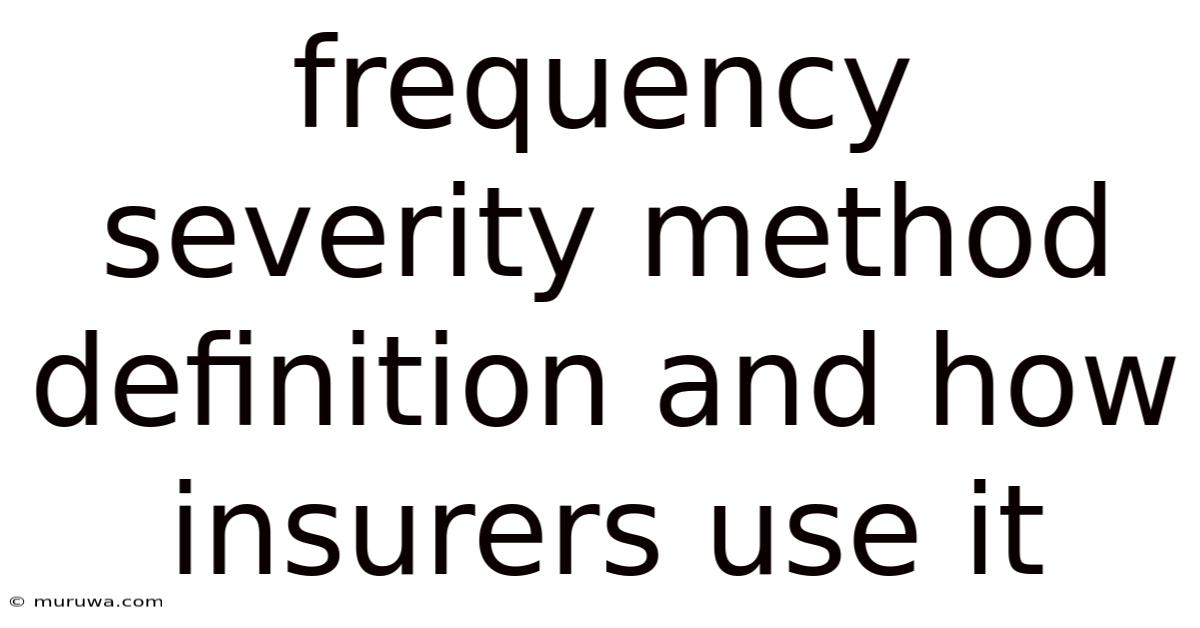
Discover more detailed and exciting information on our website. Click the link below to start your adventure: Visit Best Website meltwatermedia.ca. Don't miss out!
Table of Contents
Decoding the Frequency-Severity Method: How Insurers Assess and Manage Risk
What if accurate risk assessment were the key to unlocking sustainable profitability in the insurance industry? The frequency-severity method is a powerful tool that allows insurers to do just that, providing a robust framework for understanding and managing risk.
Editor’s Note: This article on the frequency-severity method in insurance has been published today, offering current insights into this critical risk assessment technique. This explanation will benefit insurance professionals, students, and anyone interested in understanding the inner workings of the insurance industry.
Why the Frequency-Severity Method Matters:
The frequency-severity method is a cornerstone of actuarial science and risk management within the insurance sector. It provides a structured approach to analyzing the likelihood and potential impact of insured events. Understanding this method is crucial for insurers to accurately price policies, set reserves, and ultimately, remain financially solvent. The method's applications extend beyond simple pricing; it informs underwriting decisions, reinsurance strategies, and overall risk mitigation planning. This understanding directly impacts the cost and availability of insurance for consumers and businesses.
Overview: What This Article Covers:
This article will delve into the core concepts of the frequency-severity method. We will define its key components, explore how insurers apply it in practice, and discuss its limitations and adaptations. Readers will gain a comprehensive understanding of this crucial risk assessment tool, including its practical applications and implications for the insurance industry.
The Research and Effort Behind the Insights:
This article draws upon decades of actuarial science literature, industry reports, and real-world examples. The information presented reflects established methodologies and best practices within the insurance industry. Data-driven analysis and expert opinions have been carefully considered to ensure the accuracy and reliability of the presented insights.
Key Takeaways:
- Definition and Core Concepts: A detailed explanation of frequency and severity in the context of insurance risk.
- Data Collection and Analysis: The methods used to gather and analyze relevant data for frequency-severity modeling.
- Practical Applications: How insurers leverage this method for pricing, reserving, and underwriting.
- Limitations and Refinements: Acknowledging the method's limitations and exploring refinements like severity-modifying factors.
- Future Trends: Discussing the evolving role of technology and data analytics in enhancing frequency-severity analysis.
Smooth Transition to the Core Discussion:
Having established the importance of the frequency-severity method, let's now explore its core components and application within the insurance industry in more detail.
Exploring the Key Aspects of the Frequency-Severity Method:
1. Definition and Core Concepts:
The frequency-severity method analyzes risk by separating it into two key components:
-
Frequency: This refers to the number of insured events (claims) expected within a specific timeframe, such as a year. For example, the frequency of auto accidents for a particular group of drivers. This is often expressed as a rate, like the number of claims per 100 insured vehicles.
-
Severity: This represents the average cost or size of each claim. Returning to the auto accident example, severity would be the average cost of repairing a vehicle after an accident. This is typically expressed as a dollar amount or a range.
The power of this method lies in its simplicity and clarity. By separating frequency and severity, insurers can gain a more nuanced understanding of their exposure to risk. A high-frequency, low-severity risk profile (e.g., many minor claims) differs significantly from a low-frequency, high-severity risk profile (e.g., few but very costly claims). Each requires a distinct approach to risk management and pricing.
2. Data Collection and Analysis:
Accurate data is the lifeblood of the frequency-severity method. Insurers rely on extensive historical claim data, including:
- Claim count: The total number of claims filed within a specific period.
- Claim size distribution: The distribution of claim costs, providing insights into average severity and the potential for extreme values.
- Exposures: The amount of risk the insurer is exposed to (e.g., number of insured vehicles, total insured value).
Statistical techniques, including frequency distributions (e.g., Poisson, negative binomial) and severity distributions (e.g., log-normal, Pareto), are employed to model and analyze this data. These models allow insurers to project future frequency and severity, which are essential for pricing and reserving. Sophisticated statistical software and actuarial modeling techniques are typically used for this purpose.
3. Practical Applications:
Insurers employ the frequency-severity method across various aspects of their operations:
-
Pricing: By combining projections of frequency and severity, insurers can calculate the expected cost of claims for a given risk profile. This expected cost, along with operational expenses and desired profit margins, determines the premium charged to policyholders.
-
Reserving: Insurers set aside funds (reserves) to cover future claims. The frequency-severity method helps estimate the amount of reserves needed to meet expected liabilities, ensuring financial stability. This is crucial for long-tail lines of insurance, where claims can take years to materialize (e.g., liability insurance).
-
Underwriting: The method guides underwriting decisions. By analyzing the frequency and severity of claims associated with specific risk characteristics (e.g., age, driving history, location), insurers can assess the riskiness of potential policyholders and adjust premiums accordingly. It also helps identify risk factors that may require further investigation or mitigation.
-
Reinsurance: Reinsurers utilize frequency-severity analysis to assess the risk they assume when covering a portion of an insurer's liabilities. Understanding the underlying frequency and severity allows them to price their reinsurance contracts effectively.
4. Limitations and Refinements:
The frequency-severity method, while powerful, has limitations:
-
Data Dependency: Accurate results depend heavily on the quality and quantity of historical data. Limited or biased data can lead to inaccurate projections.
-
Model Assumptions: The chosen statistical distributions and model assumptions can influence results. Care must be taken in selecting appropriate models and validating their assumptions.
-
Ignoring Correlation: The basic method typically assumes independence between frequency and severity. In reality, these factors can be correlated (e.g., higher severity claims might be associated with higher claim frequencies).
-
Inflation: Inflation can significantly affect severity estimates. Adjustments for inflation are crucial for accurate forecasting.
To address these limitations, refinements have been developed:
-
Severity-Modifying Factors: These factors account for characteristics that influence claim severity without affecting frequency (e.g., inflation, changes in medical costs).
-
More Complex Models: More sophisticated statistical models can account for correlations between frequency and severity and incorporate additional explanatory variables.
5. Future Trends:
Technological advancements are significantly impacting the frequency-severity method:
-
Big Data and Analytics: The availability of vast datasets and advanced analytical techniques allows for more accurate and granular risk assessment.
-
Machine Learning: Machine learning algorithms can identify complex patterns and relationships in claim data, improving the accuracy of frequency and severity predictions.
-
Telematics and IoT: Data collected from connected devices (e.g., telematics in vehicles) provides real-time insights into driving behavior and risk, improving the accuracy of frequency estimations.
Exploring the Connection Between Data Quality and the Frequency-Severity Method:
The relationship between data quality and the effectiveness of the frequency-severity method is paramount. High-quality data, characterized by completeness, accuracy, and consistency, is crucial for reliable model building and accurate risk assessments. Conversely, poor data quality can lead to inaccurate predictions, potentially impacting pricing, reserving, and overall risk management.
Key Factors to Consider:
-
Roles and Real-World Examples: Inaccurate data, such as missing claim information or misclassified claim types, can directly skew frequency and severity estimates. For example, underreporting of minor accidents can lead to an underestimation of frequency, while inaccurate cost estimations can distort severity measures.
-
Risks and Mitigations: The risks associated with poor data quality include inaccurate premiums, inadequate reserves, and increased financial vulnerability. Mitigating these risks requires robust data validation processes, quality control checks, and the implementation of data governance frameworks.
-
Impact and Implications: The consequences of using flawed data in frequency-severity models can be significant, ranging from pricing errors that lead to losses to insufficient reserves that expose the insurer to solvency risks. Ultimately, it erodes the insurer's ability to accurately assess and manage risk.
Conclusion: Reinforcing the Connection:
The quality of data directly determines the reliability of the frequency-severity method. Investing in data quality and implementing effective data management processes are essential for insurers to leverage this powerful tool for accurate risk assessment and sound business decisions.
Further Analysis: Examining Data Collection Methods in Greater Detail:
Effective data collection is the foundation of accurate frequency-severity analysis. Insurers employ a variety of methods, including:
-
Claim Reporting Systems: These systems capture detailed information on individual claims, including dates, types of losses, and settlement amounts. Regular data audits and validation are critical to ensure data integrity.
-
Policy Information Systems: These systems provide details on insured items, policy terms, and risk characteristics. This information is used to calculate exposures and segment the insured population for analysis.
-
Third-Party Data Providers: Insurers may supplement their internal data with external data sources, such as government statistics, industry benchmarks, and location-based risk information.
-
Data Mining and Predictive Analytics: Advanced techniques are used to identify trends, patterns, and anomalies in claim data, enriching the insights derived from frequency-severity models.
FAQ Section: Answering Common Questions About the Frequency-Severity Method:
Q: What is the difference between the frequency-severity method and other risk assessment methods?
A: While other methods exist (e.g., loss ratio analysis), the frequency-severity method uniquely separates frequency and severity, providing a more detailed and nuanced understanding of risk. This allows for a more granular approach to pricing and reserving.
Q: How often should insurers update their frequency-severity models?
A: The frequency of model updates depends on the volatility of the insured risk and the availability of new data. Annual updates are common, but more frequent updates might be needed in rapidly changing environments.
Q: Can the frequency-severity method be used for all types of insurance?
A: Yes, though the specific applications and challenges might vary depending on the line of insurance. The method is widely applicable across property, casualty, and health insurance.
Practical Tips: Maximizing the Benefits of the Frequency-Severity Method:
- Invest in data quality: Ensure data accuracy, completeness, and consistency.
- Choose appropriate statistical models: Select models that accurately reflect the distribution of frequency and severity.
- Regularly update models: Incorporate new data to maintain model accuracy and relevance.
- Consider severity-modifying factors: Account for inflation and other factors impacting claim costs.
- Validate model assumptions: Regularly assess the validity of underlying assumptions.
Final Conclusion: Wrapping Up with Lasting Insights:
The frequency-severity method is a fundamental tool for insurers to accurately assess and manage risk. By understanding its core components, applications, and limitations, insurers can optimize pricing strategies, allocate reserves effectively, and build a more sustainable and profitable business. The continued evolution of data analytics and technology will further enhance this method's power and precision, paving the way for even more sophisticated risk management practices within the insurance industry.
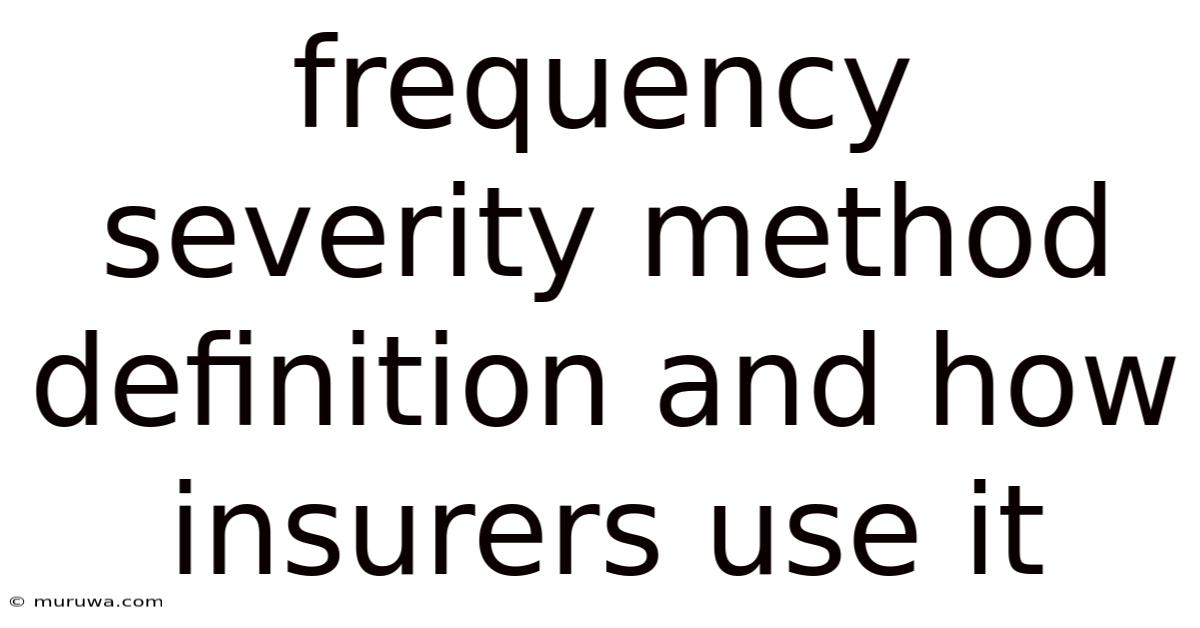
Thank you for visiting our website wich cover about Frequency Severity Method Definition And How Insurers Use It. We hope the information provided has been useful to you. Feel free to contact us if you have any questions or need further assistance. See you next time and dont miss to bookmark.
Also read the following articles
Article Title | Date |
---|---|
Franchise Cover Definition | Apr 26, 2025 |
Fragmentation Definition Examples Pros And Cons In Business | Apr 26, 2025 |
Front End Load Definition Types Average Percentage And Example | Apr 26, 2025 |
Fugit Definition | Apr 26, 2025 |
Front Office Definition Duties Front Office Vs Back Office | Apr 26, 2025 |