Fine Tuning Definition
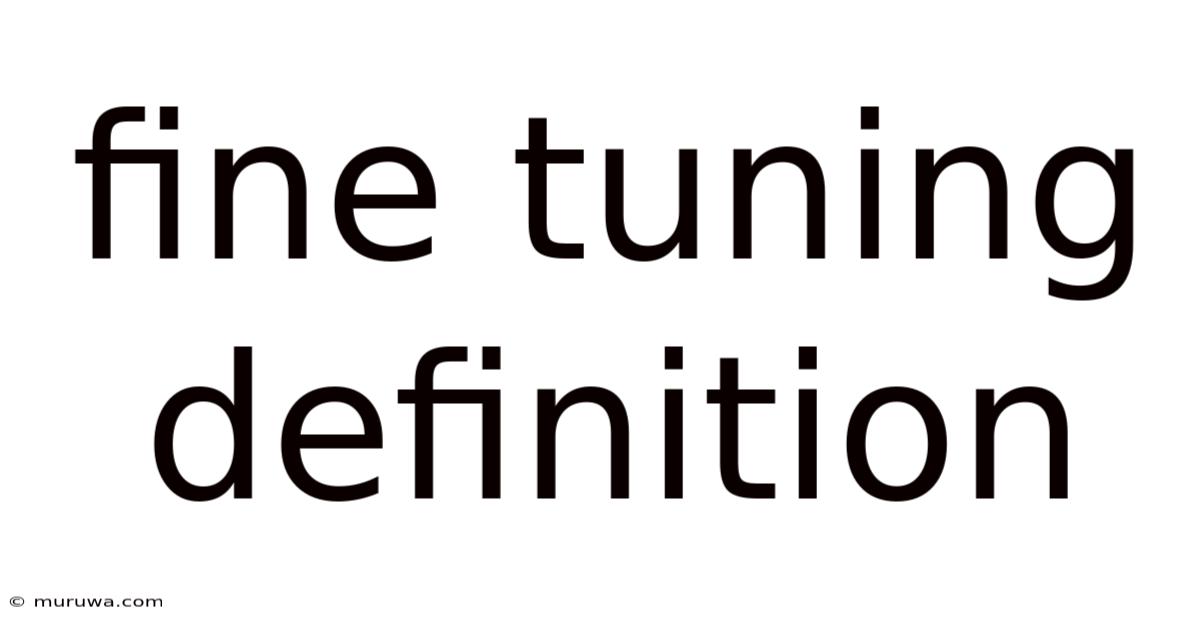
Discover more detailed and exciting information on our website. Click the link below to start your adventure: Visit Best Website meltwatermedia.ca. Don't miss out!
Table of Contents
Fine-Tuning: Unleashing the Potential of Pre-trained Models
What if the future of artificial intelligence hinges on our ability to effectively fine-tune pre-trained models? This crucial process is rapidly transforming AI, allowing for the creation of highly specialized and efficient applications across diverse fields.
Editor’s Note: This article on fine-tuning provides a comprehensive overview of this critical aspect of machine learning, exploring its techniques, benefits, and challenges. The information presented here is current as of today and incorporates insights from recent research and industry practices.
Why Fine-Tuning Matters: Relevance, Practical Applications, and Industry Significance
Fine-tuning, in the context of machine learning, refers to the process of adapting a pre-trained model to a specific task or dataset. Instead of training a model from scratch, which requires vast amounts of data and computational resources, fine-tuning leverages the knowledge already encoded within a pre-trained model. This significantly reduces training time, data requirements, and computational costs, making advanced AI more accessible. Its relevance spans numerous industries, including healthcare (disease diagnosis), finance (fraud detection), natural language processing (chatbots, translation), computer vision (object recognition, image classification), and many more. The ability to rapidly adapt pre-trained models to new domains is a key driver of innovation and efficiency in the AI landscape.
Overview: What This Article Covers
This article delves into the core aspects of fine-tuning, providing a detailed explanation of its mechanics, various techniques, advantages, limitations, and real-world applications. Readers will gain a thorough understanding of how fine-tuning works, its impact on model performance, and best practices for its successful implementation. We will also explore the relationship between fine-tuning and transfer learning, addressing common challenges and outlining strategies for optimization.
The Research and Effort Behind the Insights
This article is the result of extensive research, drawing upon numerous academic publications, industry reports, and practical experience in the field of machine learning. The information presented is supported by evidence from credible sources, ensuring accuracy and providing readers with trustworthy insights. The structured approach employed aims to provide a clear and comprehensive understanding of fine-tuning, its nuances, and its implications for the future of AI.
Key Takeaways:
- Definition and Core Concepts: A clear definition of fine-tuning and its foundational principles within the context of transfer learning.
- Practical Applications: Real-world examples of fine-tuning across diverse industries and applications.
- Techniques and Strategies: An exploration of various fine-tuning techniques, including parameter-efficient fine-tuning methods.
- Challenges and Solutions: Identification of common challenges and strategies to overcome them.
- Future Implications: Discussion of the long-term impact of fine-tuning on AI development and deployment.
Smooth Transition to the Core Discussion:
Having established the significance of fine-tuning, let’s delve into the intricacies of this powerful technique, exploring its underlying mechanisms, practical applications, and potential limitations.
Exploring the Key Aspects of Fine-Tuning
1. Definition and Core Concepts:
Fine-tuning builds upon the concept of transfer learning. Transfer learning involves leveraging knowledge gained from solving one problem to improve performance on a related problem. A pre-trained model, usually trained on a massive dataset (like ImageNet for computer vision or a massive text corpus for NLP), has already learned general features and representations. Fine-tuning adapts these pre-trained weights to a new, more specific task by further training the model on a smaller, task-specific dataset. This targeted training refines the model's parameters, aligning its learned features with the nuances of the new task. The key is to utilize the pre-trained knowledge as a strong starting point, requiring less data and computational power than training from scratch.
2. Applications Across Industries:
Fine-tuning finds extensive applications across various domains:
-
Natural Language Processing (NLP): Fine-tuning pre-trained language models like BERT, RoBERTa, and GPT-3 allows for highly accurate sentiment analysis, text classification, question answering, and machine translation tailored to specific languages or domains. For instance, a model pre-trained on a massive English corpus can be fine-tuned on a smaller dataset of medical texts to perform accurate medical diagnosis prediction.
-
Computer Vision: Pre-trained models like ResNet, Inception, and EfficientNet, initially trained on ImageNet, can be fine-tuned for specific image classification tasks (e.g., identifying different types of cancer cells in medical images) or object detection tasks (e.g., autonomous driving). The pre-trained model provides a robust foundation, reducing the need for extensive training data for the new task.
-
Speech Recognition: Pre-trained acoustic models can be fine-tuned to improve accuracy for specific accents, noise levels, or speakers. This is crucial for building robust speech-to-text systems that work well in diverse real-world environments.
-
Time Series Analysis: Fine-tuning pre-trained models on time series data can be used for forecasting tasks in finance (stock prices), energy (demand prediction), or weather (forecasting).
3. Techniques and Strategies:
Several fine-tuning techniques exist, each with its strengths and weaknesses:
-
Full Fine-Tuning: All layers of the pre-trained model are updated during training. This offers maximum flexibility but requires more computational resources and is prone to overfitting on small datasets.
-
Partial Fine-Tuning: Only certain layers of the model (typically the later layers) are fine-tuned, while the earlier layers (which capture general features) remain frozen. This reduces computational cost and the risk of overfitting.
-
Feature Extraction: The pre-trained model is used as a fixed feature extractor. Only a new classifier is trained on top of the fixed feature representation. This is the simplest approach, requiring minimal computational resources, but may limit the model's ability to adapt to the new task.
-
Parameter-Efficient Fine-Tuning (PEFT): These techniques aim to minimize the number of trainable parameters during fine-tuning. Examples include:
- Adapter Modules: Small networks are inserted into the pre-trained model, learning task-specific adaptations without changing the original weights.
- Prefix Tuning: A small set of parameters is added to the input, guiding the model's behavior without modifying the core model.
- Prompt Tuning: Learned prompts are added to the input, influencing the model's output without updating the model's weights. This is particularly useful for large language models.
4. Challenges and Solutions:
Fine-tuning, while powerful, presents certain challenges:
-
Overfitting: Fine-tuning on small datasets can lead to overfitting, where the model performs well on the training data but poorly on unseen data. Regularization techniques (e.g., dropout, weight decay), data augmentation, and early stopping can mitigate this.
-
Catastrophic Forgetting: The model may "forget" what it learned during pre-training while adapting to the new task. Techniques like regularization and careful selection of the learning rate can help alleviate this.
-
Computational Cost: While less than training from scratch, fine-tuning still requires computational resources. Parameter-efficient fine-tuning methods can help address this.
-
Data Scarcity: The success of fine-tuning relies on having sufficient data for the new task. Data augmentation and transfer learning from related tasks can help compensate for limited data.
5. Impact on Innovation:
Fine-tuning has profoundly impacted AI innovation by:
-
Democratizing AI: Reducing computational costs and data requirements makes advanced AI accessible to researchers and developers with limited resources.
-
Accelerating Development: Fine-tuning significantly shortens development time compared to training from scratch, enabling faster iteration and innovation.
-
Enabling Specialized Applications: Fine-tuning allows for the creation of highly specialized AI models tailored to niche tasks and domains.
Exploring the Connection Between Transfer Learning and Fine-Tuning
Transfer learning is the broader concept, while fine-tuning is a specific technique within transfer learning. Transfer learning encompasses various methods for leveraging knowledge from one task to improve performance on another. Fine-tuning is a prominent method where the pre-trained model's parameters are directly adjusted to adapt to the new task.
Key Factors to Consider:
-
Roles and Real-World Examples: Transfer learning provides the framework, while fine-tuning provides the mechanism for adapting the pre-trained model. For example, a pre-trained model for image classification on ImageNet can be fine-tuned for medical image analysis, leveraging the general image features learned during pre-training to improve the accuracy and efficiency of medical image diagnosis.
-
Risks and Mitigations: Overfitting and catastrophic forgetting are key risks. Mitigation strategies include regularization, data augmentation, and careful hyperparameter tuning.
-
Impact and Implications: Transfer learning and fine-tuning have dramatically increased the efficiency and effectiveness of AI model development, leading to faster progress in various AI applications.
Conclusion: Reinforcing the Connection:
The relationship between transfer learning and fine-tuning is synergistic. Transfer learning provides the conceptual foundation, while fine-tuning offers a powerful practical implementation. By understanding this connection, developers can effectively leverage pre-trained models to create highly specialized and efficient AI systems.
Further Analysis: Examining Transfer Learning in Greater Detail
Transfer learning is a vast field encompassing various techniques beyond fine-tuning, such as feature extraction, domain adaptation, and multi-task learning. These methods differ in how they leverage pre-trained knowledge, offering varying degrees of adaptation and flexibility depending on the specific application and available resources.
FAQ Section: Answering Common Questions About Fine-Tuning
Q: What is fine-tuning?
A: Fine-tuning is the process of adapting a pre-trained model to a specific task or dataset by further training the model on a smaller, task-specific dataset.
Q: What are the benefits of fine-tuning?
A: Fine-tuning reduces training time, data requirements, and computational costs compared to training from scratch.
Q: What are the challenges of fine-tuning?
A: Challenges include overfitting, catastrophic forgetting, and computational cost.
Q: What are some examples of parameter-efficient fine-tuning methods?
A: Examples include adapter modules, prefix tuning, and prompt tuning.
Practical Tips: Maximizing the Benefits of Fine-Tuning
-
Choose an appropriate pre-trained model: Select a model whose architecture and pre-training data are relevant to the new task.
-
Carefully select the hyperparameters: Experiment with different learning rates, batch sizes, and regularization techniques to optimize performance.
-
Use data augmentation: Increase the size and diversity of the training data to improve generalization and reduce overfitting.
-
Monitor performance closely: Track the model's performance on validation data to detect overfitting and adjust the training process accordingly.
-
Consider parameter-efficient fine-tuning: For resource-constrained environments or very large pre-trained models, explore parameter-efficient methods.
Final Conclusion: Wrapping Up with Lasting Insights
Fine-tuning represents a pivotal advancement in machine learning, enabling the creation of highly specialized and efficient AI models across numerous domains. By understanding its techniques, challenges, and best practices, researchers and developers can harness its power to accelerate innovation and democratize access to advanced AI technologies. Its continued development and refinement will undoubtedly play a crucial role in shaping the future of artificial intelligence.
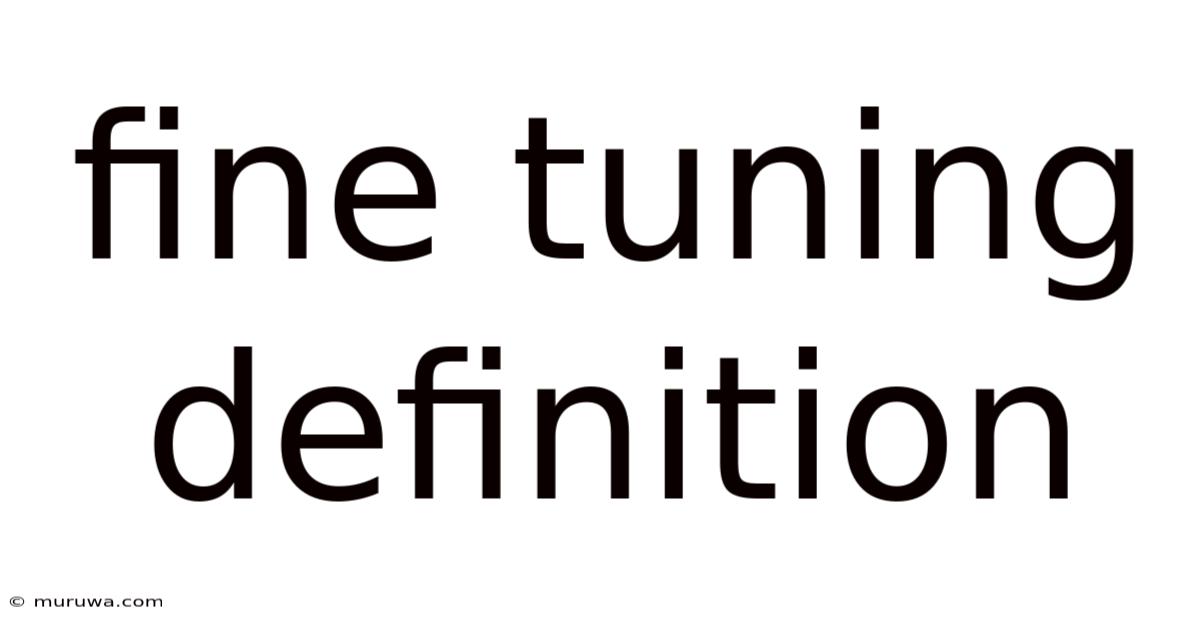
Thank you for visiting our website wich cover about Fine Tuning Definition. We hope the information provided has been useful to you. Feel free to contact us if you have any questions or need further assistance. See you next time and dont miss to bookmark.
Also read the following articles
Article Title | Date |
---|---|
How Much Does Dexcom Cost Without Insurance | Apr 12, 2025 |
Which Most Effectively Describes What Weaken The Stock Market In The Late 1920s | Apr 12, 2025 |
What Is Sr 50 Insurance | Apr 12, 2025 |
How To Appeal Car Insurance Claim Denial | Apr 12, 2025 |
Fire Insurance Definition Elements How It Works And Example | Apr 12, 2025 |