Error Term Definition Example And How To Calculate With Formula
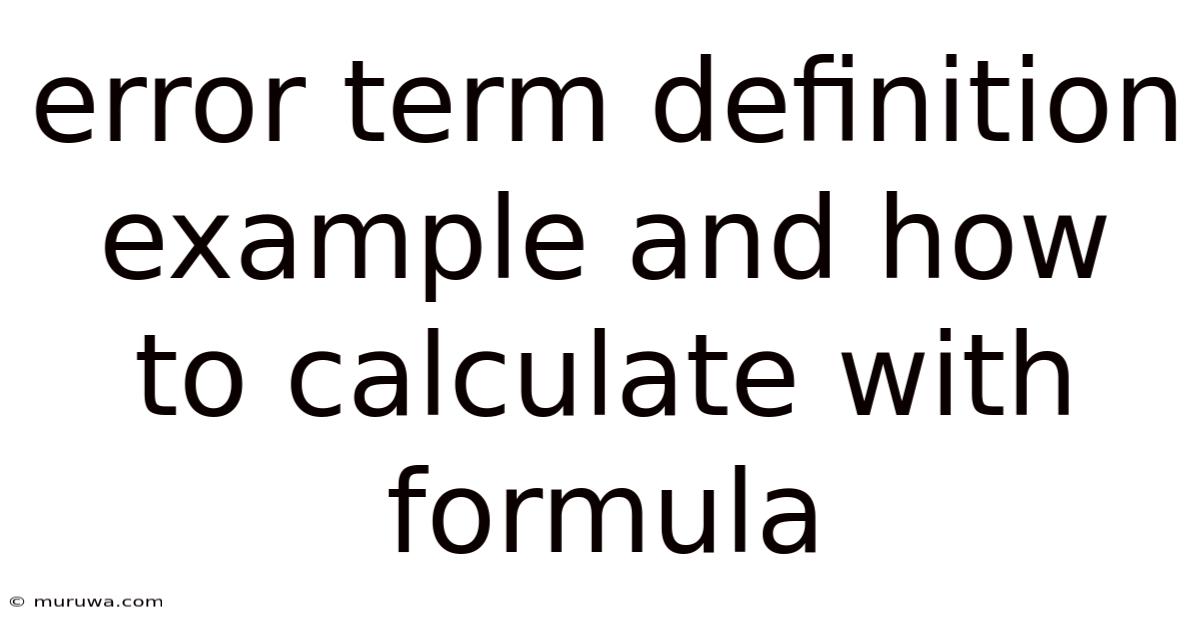
Discover more detailed and exciting information on our website. Click the link below to start your adventure: Visit Best Website meltwatermedia.ca. Don't miss out!
Table of Contents
Unveiling the Enigma: Error Term Definition, Examples, and Calculation
What if the accuracy of our predictions hinges on understanding the elusive error term? This fundamental statistical concept underpins the reliability of countless models and analyses, offering crucial insights into data variability and model limitations.
Editor’s Note: This article provides a comprehensive exploration of the error term, covering its definition, various examples across different statistical contexts, and methods for its calculation (where applicable). We aim to demystify this crucial statistical concept for both students and professionals.
Why the Error Term Matters: Relevance, Practical Applications, and Industry Significance
The error term, often represented as ε (epsilon), is a cornerstone of statistical modeling. It represents the unexplained variation in a dependent variable that is not accounted for by the independent variables in a model. Understanding and analyzing this error is crucial for several reasons:
- Model Accuracy Assessment: The magnitude of the error term directly reflects the model's predictive power. A smaller error suggests a better fit, while a larger error indicates limitations in the model's ability to explain the data.
- Hypothesis Testing: Error terms are fundamental to hypothesis testing procedures, determining the statistical significance of relationships between variables.
- Prediction Interval Estimation: Accurate error term estimation enables the construction of prediction intervals, providing a range within which future observations are likely to fall.
- Model Improvement: Analyzing the pattern and distribution of error terms can reveal potential model inadequacies, leading to improvements in model specification, variable selection, or the inclusion of additional explanatory variables.
- Across Industries: The significance of error terms extends across diverse fields, including finance (predicting stock prices), healthcare (modeling disease progression), engineering (analyzing system performance), and social sciences (examining social trends).
Overview: What This Article Covers
This article provides a detailed explanation of the error term, starting with its fundamental definition and moving through diverse examples in various statistical models. We will illustrate its calculation in different contexts, discuss assumptions related to the error term, and highlight common misconceptions. Finally, we will address frequently asked questions and offer practical tips for interpreting and utilizing error term information effectively.
The Research and Effort Behind the Insights
This article draws upon established statistical literature, including textbooks and research papers, to provide a comprehensive and accurate explanation of the error term. The examples used are carefully selected to represent a range of statistical applications, and the explanations are designed to be accessible to a broad audience.
Key Takeaways:
- Definition and Core Concepts: A precise definition of the error term and its role in different statistical models.
- Types of Error Terms: Examples of error terms in regression analysis, ANOVA, and time series models.
- Assumptions about Error Terms: Understanding the typical assumptions made about error terms, such as independence, normality, and constant variance.
- Calculating Error Terms: Methods for calculating or estimating error terms in different contexts.
- Interpreting Error Terms: Understanding what the magnitude and distribution of error terms tell us about the model and the data.
Smooth Transition to the Core Discussion
Having established the importance of understanding the error term, let’s now delve into a more detailed exploration of its definition, calculation, and interpretation in various statistical contexts.
Exploring the Key Aspects of the Error Term
1. Definition and Core Concepts:
The error term (ε) in a statistical model represents the difference between the observed value of the dependent variable (Y) and the predicted value of the dependent variable (Ŷ) based on the model. Mathematically, this can be expressed as:
ε = Y - Ŷ
In simpler terms, the error term captures the portion of the dependent variable that the model fails to explain. This unexplained variation can stem from various sources, including:
- Omitted Variables: Relevant variables not included in the model.
- Measurement Error: Inaccuracies in measuring the dependent or independent variables.
- Random Fluctuations: Inherent randomness in the data-generating process.
- Model Misspecification: Using an inappropriate model for the data.
2. Applications Across Industries:
The error term finds applications across various domains:
- Linear Regression: In simple linear regression, the error term represents the vertical distance between each data point and the regression line.
- Multiple Regression: In multiple regression, the error term represents the deviation of each observed value from the predicted value based on the multiple independent variables.
- Analysis of Variance (ANOVA): In ANOVA, the error term reflects the variability within each group being compared.
- Time Series Analysis: In time series models, the error term accounts for the unpredictable fluctuations in the data over time.
3. Challenges and Solutions:
A significant challenge in working with error terms is ensuring they meet certain assumptions, primarily:
- Independence: Error terms should be independent of each other. Autocorrelation (correlation between consecutive error terms) violates this assumption.
- Normality: Error terms should ideally follow a normal distribution. This assumption is crucial for hypothesis testing.
- Homoscedasticity: Error terms should have a constant variance across all levels of the independent variables. Heteroscedasticity (non-constant variance) can lead to inefficient and biased estimates.
Addressing these challenges often involves techniques like data transformations, robust regression methods, or the use of generalized linear models.
4. Impact on Innovation:
A deeper understanding of error terms is driving innovation in statistical modeling. New methods are constantly being developed to handle complex error structures, allowing for more accurate and robust analyses. This leads to better predictions, more reliable inferences, and more informed decision-making across diverse fields.
Closing Insights: Summarizing the Core Discussion
The error term is not merely a residual; it's a vital piece of information revealing the limitations and strengths of a statistical model. By carefully examining and addressing issues related to error term assumptions, analysts can significantly enhance the reliability and validity of their findings.
Exploring the Connection Between Model Assumptions and the Error Term
The validity of statistical inferences heavily relies on the assumptions made about the error term. Let's examine this crucial connection:
Roles and Real-World Examples:
- Linearity: The assumption of linearity implies a linear relationship between the independent and dependent variables. Violations can lead to biased estimates and inaccurate predictions. For instance, modeling the relationship between advertising expenditure and sales using linear regression might be inappropriate if the relationship exhibits diminishing returns at higher expenditure levels.
- Normality: The normality assumption allows for the use of standard statistical tests based on the normal distribution. Non-normality can lead to inaccurate p-values and confidence intervals. For example, analyzing income data that is heavily skewed might require transformations to improve normality.
- Homoscedasticity: Constant variance ensures that the precision of the model's predictions is consistent across the range of independent variable values. Heteroscedasticity leads to inefficient estimators and inaccurate standard errors. Consider a study investigating the relationship between house size and price; heteroscedasticity might occur if the variability in price is higher for larger houses.
- Independence: The assumption of independent error terms prevents bias in estimation and inference. Autocorrelation, a common violation, often indicates a missing variable or an inappropriate model. For example, analyzing monthly stock returns without accounting for autocorrelation could lead to incorrect conclusions about risk and returns.
Risks and Mitigations:
Failing to meet error term assumptions can result in biased parameter estimates, inflated or deflated standard errors, and unreliable p-values. Mitigation strategies include:
- Data Transformations: Applying transformations (log, square root) to the dependent or independent variables can often stabilize variance and improve normality.
- Robust Regression: Robust regression methods are less sensitive to violations of normality and homoscedasticity assumptions.
- Generalized Linear Models (GLMs): GLMs are designed to handle non-normal error distributions and non-constant variance.
- Autocorrelation Correction: Techniques like ARIMA modeling can be used to address autocorrelation in time series data.
Impact and Implications:
The consequences of violating error term assumptions extend beyond inaccurate parameter estimates. It can lead to flawed conclusions about the relationships between variables, inaccurate predictions, and poorly informed decision-making. This can have significant implications in various fields, from clinical trials to financial forecasting.
Conclusion: Reinforcing the Connection
The relationship between model assumptions and the error term is fundamental to the reliability of statistical inferences. Understanding these assumptions and implementing appropriate mitigation strategies is crucial for ensuring the validity and usefulness of statistical analyses.
Further Analysis: Examining Model Diagnostics in Greater Detail
Model diagnostic tools play a crucial role in evaluating the validity of error term assumptions. These include:
- Residual Plots: Visual examination of residual plots (plots of residuals against predicted values or independent variables) can reveal patterns indicative of non-linearity, heteroscedasticity, or non-normality.
- Normality Tests: Formal tests (e.g., Shapiro-Wilk test, Kolmogorov-Smirnov test) can assess the normality of the residuals.
- Durbin-Watson Test: This test checks for autocorrelation in the residuals.
- Breusch-Pagan Test: This test assesses the presence of heteroscedasticity.
Careful interpretation of these diagnostic tools is essential for identifying and addressing potential violations of error term assumptions.
FAQ Section: Answering Common Questions About the Error Term
Q: How can I calculate the error term in a simple linear regression?
A: In simple linear regression, the error term for each data point is calculated as the difference between the observed value of the dependent variable and the predicted value from the regression line: εᵢ = Yᵢ - Ŷᵢ, where i represents the ith observation.
Q: What does it mean if the error terms are highly correlated?
A: Highly correlated error terms (autocorrelation) suggest a violation of the independence assumption. This might indicate the omission of relevant variables or the use of an inappropriate model.
Q: How do I handle heteroscedasticity?
A: Strategies to handle heteroscedasticity include data transformations, weighted least squares regression, and the use of robust standard errors.
Practical Tips: Maximizing the Benefits of Understanding Error Terms
- Always check model diagnostics: Before interpreting results, carefully examine residual plots and conduct formal tests to assess the validity of error term assumptions.
- Consider data transformations: If assumptions are violated, consider transforming variables to improve normality and homoscedasticity.
- Explore alternative models: If assumptions cannot be met, consider using models that are more robust to violations of assumptions.
- Understand limitations: Acknowledge that perfect model fit is rarely achievable, and some degree of unexplained variation is expected.
Final Conclusion: Wrapping Up with Lasting Insights
Understanding the error term is fundamental to sound statistical analysis. By recognizing its importance, carefully examining model assumptions, and employing appropriate diagnostic tools, analysts can enhance the accuracy, reliability, and interpretability of their results, ultimately leading to better-informed decisions across all fields. The error term, while often overlooked, is the key to unlocking the true potential of statistical models and their predictive power.
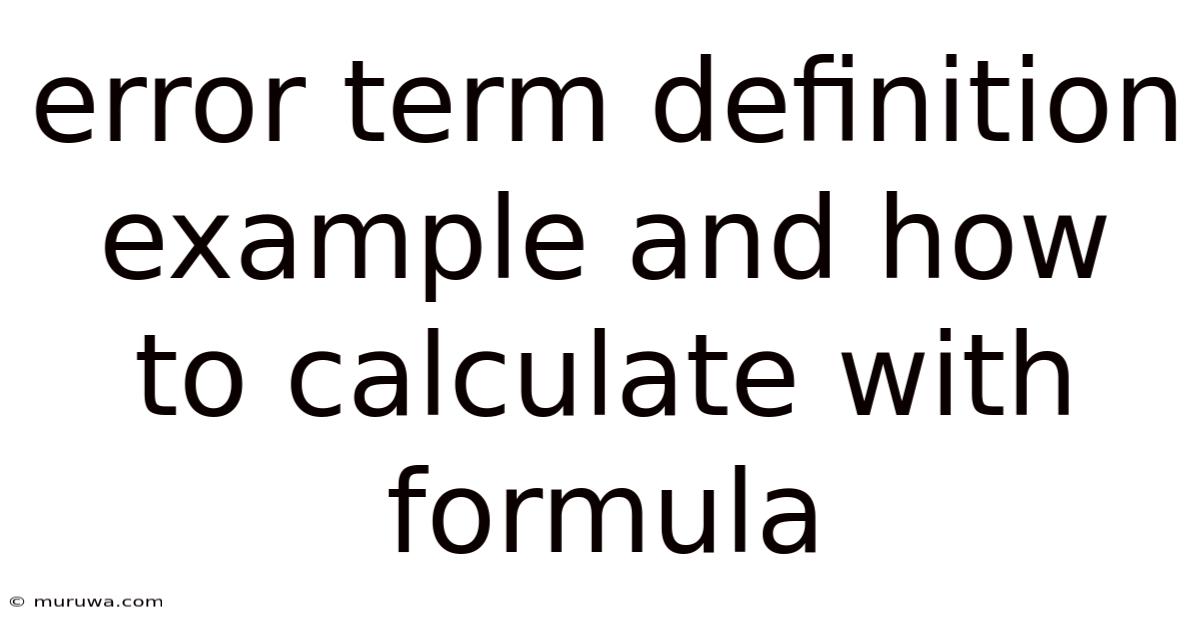
Thank you for visiting our website wich cover about Error Term Definition Example And How To Calculate With Formula. We hope the information provided has been useful to you. Feel free to contact us if you have any questions or need further assistance. See you next time and dont miss to bookmark.
Also read the following articles
Article Title | Date |
---|---|
Equity Commitment Note Ecn Definition | Apr 11, 2025 |
How Much Is Insurance For A Honda Grom | Apr 11, 2025 |
Enduring Purpose Definition And Example | Apr 11, 2025 |
How Does Recoverable Depreciation Work On An Insurance Claim | Apr 11, 2025 |
What Are Exports Definition Benefits And Examples | Apr 11, 2025 |