Endogenous Variable Definition Meaning And Examples
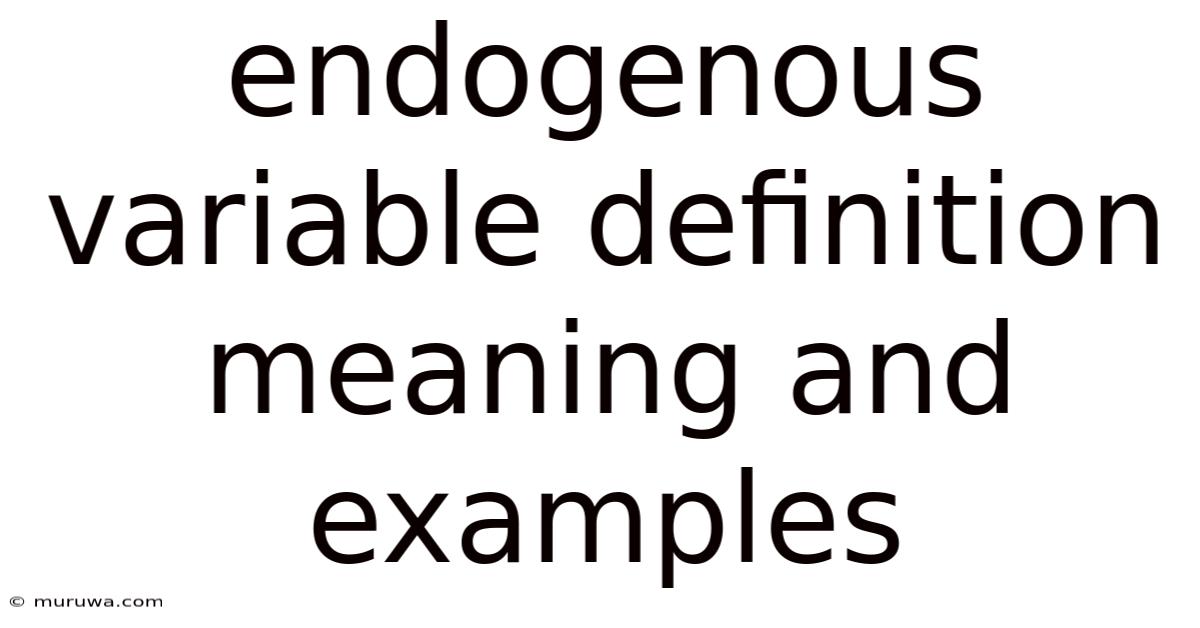
Discover more detailed and exciting information on our website. Click the link below to start your adventure: Visit Best Website meltwatermedia.ca. Don't miss out!
Table of Contents
Understanding Endogenous Variables: Definition, Meaning, and Examples
What if the success of your research hinges on correctly identifying endogenous variables? Failing to account for endogeneity can lead to seriously flawed conclusions and unreliable results.
Editor’s Note: This article on endogenous variables provides a comprehensive overview, explaining their definition, implications, and how to address them in research. It’s designed for researchers, students, and anyone interested in understanding the nuances of causal inference.
Why Endogenous Variables Matter: Relevance, Practical Applications, and Industry Significance
Endogenous variables are a critical concept in econometrics, statistics, and various other fields employing causal modeling. Understanding endogeneity is paramount because it directly impacts the validity and reliability of research findings, particularly when attempting to establish causal relationships. Failing to account for endogenous variables can lead to biased and inconsistent estimates, rendering the research conclusions unreliable and potentially misleading. The implications extend across numerous fields, including economics, epidemiology, political science, and marketing, where causal inference is essential for informed decision-making and policy development. In business, correctly identifying and handling endogenous variables is crucial for accurate forecasting, effective marketing strategies, and sound investment decisions.
Overview: What This Article Covers
This article will delve into the core concepts of endogenous variables, exploring their definition, causes, consequences, and practical solutions. We will examine various techniques used to address endogeneity, providing clear explanations and illustrating each concept with relevant examples. The article aims to equip readers with a comprehensive understanding of endogenous variables, enabling them to conduct more robust and reliable research.
The Research and Effort Behind the Insights
This article synthesizes information from leading econometrics textbooks, peer-reviewed journals, and reputable online resources. The explanations are grounded in established statistical principles and supported by illustrative examples drawn from diverse fields. A structured approach is employed to ensure clarity and facilitate understanding, making complex concepts accessible to a wider audience.
Key Takeaways:
- Definition and Core Concepts: A precise definition of endogenous variables and a detailed explanation of their characteristics.
- Causes of Endogeneity: Identification of various factors contributing to endogeneity in research settings.
- Consequences of Ignoring Endogeneity: An analysis of the potential biases and misleading conclusions that can arise from neglecting endogeneity.
- Methods for Addressing Endogeneity: A comprehensive review of established techniques for dealing with endogenous variables, including instrumental variables (IV), two-stage least squares (2SLS), and control function approaches.
- Examples Across Disciplines: Real-world illustrations of endogeneity from various fields, showcasing the practical implications of this concept.
Smooth Transition to the Core Discussion
Having established the importance of understanding endogenous variables, let's now explore their definition and the factors that contribute to their presence in research models.
Exploring the Key Aspects of Endogenous Variables
Definition and Core Concepts:
An endogenous variable is a variable within a statistical model that is correlated with the error term. This correlation implies that the variable is not independent of the factors influencing the dependent variable, violating a core assumption of many statistical methods, particularly ordinary least squares (OLS) regression. In simpler terms, an endogenous variable is influenced by, or influences, other variables within the model in a way that creates a spurious correlation or masks the true causal effect.
Causes of Endogeneity:
Several factors can lead to endogeneity:
-
Omitted Variable Bias: This occurs when a relevant variable that affects both the independent and dependent variables is excluded from the model. The omitted variable's influence is absorbed into the error term, creating a correlation between the included independent variable and the error term.
-
Simultaneity Bias: This arises when there is a feedback loop between the independent and dependent variables. Each variable influences the other, creating a simultaneous relationship that violates the assumption of independent variables.
-
Measurement Error: Errors in measuring an independent variable can also lead to endogeneity. If the measurement error is correlated with other variables in the model, it will contaminate the error term, leading to biased estimates.
-
Sample Selection Bias: This occurs when the sample used for the analysis is not representative of the population of interest. This can lead to a correlation between the independent variable and the error term if the selection process is related to the dependent variable.
Consequences of Ignoring Endogeneity:
Ignoring endogeneity leads to several serious problems:
-
Inconsistent Estimates: The estimated coefficients of the model will not converge to their true values, even with an infinitely large sample size.
-
Biased Standard Errors: The standard errors of the estimated coefficients will be incorrect, leading to unreliable hypothesis tests and confidence intervals.
-
Misleading Causal Inferences: The estimated relationship between the independent and dependent variables will not accurately reflect the true causal effect, potentially leading to flawed conclusions and inappropriate policy recommendations.
Methods for Addressing Endogeneity:
Several techniques can mitigate the effects of endogeneity:
-
Instrumental Variables (IV) Estimation: This technique involves finding an instrument – a variable that is correlated with the endogenous variable but uncorrelated with the error term. The instrument helps to isolate the effect of the endogenous variable on the dependent variable, eliminating the bias. Two-Stage Least Squares (2SLS) is a common method used to implement IV estimation.
-
Two-Stage Least Squares (2SLS): As mentioned above, this is a specific implementation of the instrumental variables approach. In the first stage, the endogenous variable is regressed on the instrument and other exogenous variables. The predicted values from this regression are then used as a substitute for the endogenous variable in the second stage regression.
-
Control Function Approach: This method involves adding a control variable to the model that captures the correlation between the endogenous variable and the error term. The control variable is typically obtained from a first-stage regression of the endogenous variable on other relevant variables.
-
Panel Data Methods: If data are available over multiple time periods or for multiple individuals, panel data methods can be used to address endogeneity. Fixed effects and random effects models can control for unobserved heterogeneity that might otherwise contribute to endogeneity.
Examples Across Disciplines:
-
Economics: Estimating the effect of education on wages. Ability is an omitted variable that affects both education and wages, creating endogeneity. Instruments such as proximity to a college or compulsory schooling laws can be used to address this.
-
Epidemiology: Studying the effect of smoking on lung cancer. Genetic predisposition to both smoking and lung cancer is an omitted variable that creates endogeneity. Using family history of smoking as an instrument (while accounting for its potential limitations) may be considered.
-
Marketing: Analyzing the effect of advertising expenditure on sales. Simultaneity bias can occur if sales influence advertising expenditure (e.g., high sales leading to increased advertising). Lagged advertising expenditures might be used as an instrument.
-
Political Science: Examining the impact of campaign spending on election outcomes. Candidate quality is an omitted variable that affects both campaign spending and election outcomes. Instruments such as previous election results or donor characteristics could potentially be used, with careful consideration of potential biases.
Exploring the Connection Between Omitted Variable Bias and Endogenous Variables
Omitted variable bias is a significant contributor to endogeneity. It arises when a relevant variable that influences both the independent and dependent variables is not included in the regression model. This omitted variable's effect is absorbed into the error term, creating a correlation between the included independent variable and the error term, thus making the included independent variable endogenous.
Key Factors to Consider:
-
Roles and Real-World Examples: In the wage-education example, ability is a crucial omitted variable. Individuals with higher ability tend to obtain more education and earn higher wages. Failing to account for ability leads to an overestimation of the effect of education on wages.
-
Risks and Mitigations: The primary risk of omitting relevant variables is biased and inconsistent estimates. Mitigation strategies include carefully specifying the model based on theoretical understanding, using richer datasets with more variables, and employing techniques like IV or control functions to address the endogeneity.
-
Impact and Implications: Omitted variable bias can lead to inaccurate conclusions about causal relationships, potentially resulting in misguided policies or ineffective interventions. For instance, overestimating the effect of education on wages might lead to policies that prioritize educational attainment without addressing underlying ability differences.
Conclusion: Reinforcing the Connection
The connection between omitted variable bias and endogeneity highlights the crucial role of careful model specification and the potential pitfalls of neglecting relevant variables. By acknowledging and addressing omitted variable bias, researchers can improve the validity and reliability of their causal inferences.
Further Analysis: Examining Omitted Variable Bias in Greater Detail
Omitted variable bias can manifest in various ways, depending on the nature of the omitted variable and its relationship with the included variables. For instance, the direction of the bias depends on the correlation between the omitted variable and the included independent variable, and the effect of the omitted variable on the dependent variable. Careful consideration of these relationships is essential for understanding the potential impact of omitted variable bias on research findings. Advanced statistical techniques, like sensitivity analysis, can help researchers assess the potential magnitude of the bias.
FAQ Section: Answering Common Questions About Endogenous Variables
-
What is an endogenous variable? An endogenous variable is a variable within a statistical model that is correlated with the error term, implying it's not independent of factors influencing the dependent variable.
-
How can I identify an endogenous variable? Endogeneity can be suspected if regression results are inconsistent across different specifications, standard errors are unusually large, or there is theoretical reason to believe a variable is correlated with omitted factors. Formal tests, such as the Hausman test, can also help.
-
What are the consequences of ignoring endogeneity? Ignoring endogeneity leads to biased and inconsistent estimates, unreliable standard errors, and invalid causal inferences.
-
What techniques can be used to address endogeneity? Instrumental variables (IV) estimation, two-stage least squares (2SLS), control function approaches, and panel data methods are commonly used.
-
How do I choose the appropriate method to address endogeneity? The choice of method depends on the specific context, the nature of the endogeneity, and the availability of data. Careful consideration of the assumptions of each method is essential.
Practical Tips: Maximizing the Benefits of Addressing Endogeneity
-
Thorough Theoretical Understanding: Develop a strong theoretical understanding of the relationships between variables before specifying the model.
-
Data Collection and Measurement: Ensure data are collected accurately and that variables are measured reliably to minimize measurement error.
-
Model Specification: Carefully consider all potentially relevant variables and include them in the model if possible.
-
Diagnostic Testing: Use diagnostic tests to assess the presence of endogeneity and the validity of chosen instruments.
-
Sensitivity Analysis: Conduct sensitivity analysis to evaluate the robustness of the results to alternative assumptions or model specifications.
Final Conclusion: Wrapping Up with Lasting Insights
Understanding endogenous variables is crucial for conducting rigorous and reliable research. The consequences of ignoring endogeneity can be severe, leading to flawed conclusions and potentially misleading policy recommendations. By employing appropriate techniques and careful consideration of the underlying theoretical relationships, researchers can effectively address endogeneity and improve the validity and reliability of their findings across diverse fields of study. The continuous development and application of advanced econometric methods will remain essential in navigating the challenges posed by endogenous variables in causal inference.
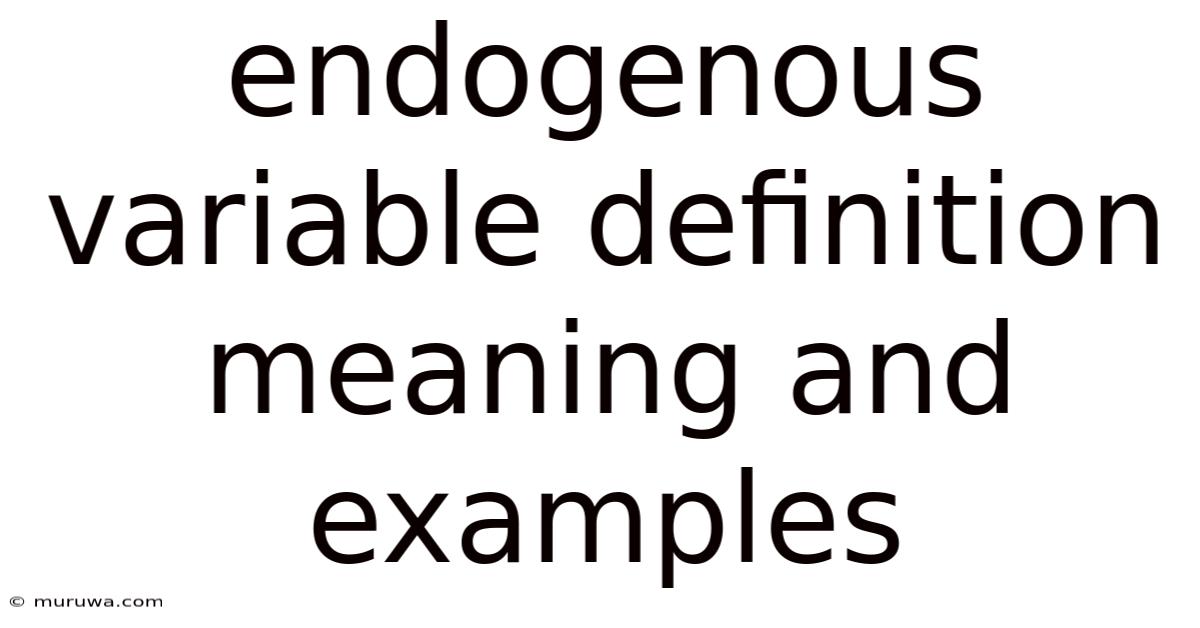
Thank you for visiting our website wich cover about Endogenous Variable Definition Meaning And Examples. We hope the information provided has been useful to you. Feel free to contact us if you have any questions or need further assistance. See you next time and dont miss to bookmark.
Also read the following articles
Article Title | Date |
---|---|
How Long Does It Take To Get Your First Fico Score | Apr 10, 2025 |
What Credit Bureau Does Chase Use In Virginia | Apr 10, 2025 |
What Fico Score Does Navy Federal Use For Auto Loans | Apr 10, 2025 |
When Is Macys Bill Due | Apr 10, 2025 |
What Is Billing Cycle In Medical Billing | Apr 10, 2025 |