Empirical Rule Definition Formula Example How Its Used
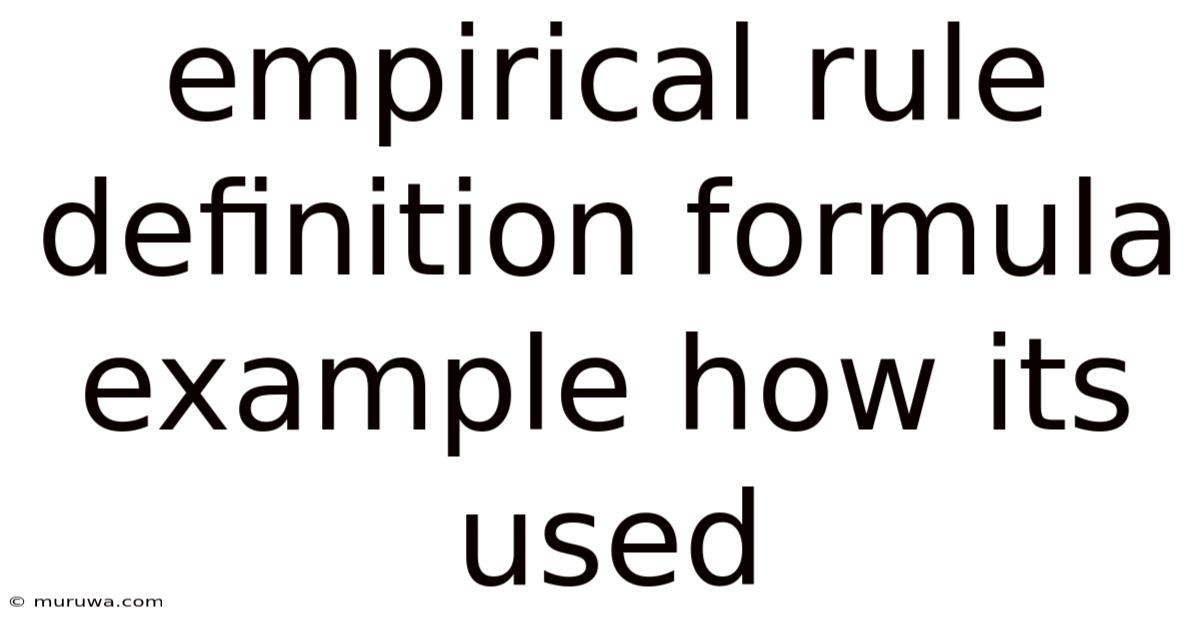
Discover more detailed and exciting information on our website. Click the link below to start your adventure: Visit Best Website meltwatermedia.ca. Don't miss out!
Table of Contents
Decoding the Empirical Rule: Definition, Formula, Examples, and Applications
What if understanding the distribution of data was as simple as following a straightforward rule? The Empirical Rule, also known as the 68-95-99.7 rule, provides a powerful shortcut for interpreting normally distributed data, offering profound insights into probability and statistics.
Editor’s Note: This article on the Empirical Rule provides a comprehensive guide, explaining its definition, formula, applications, and limitations. Updated with the latest insights, it serves as a valuable resource for students, researchers, and anyone working with data analysis.
Why the Empirical Rule Matters:
The Empirical Rule is a cornerstone of descriptive statistics, offering a simplified way to understand the distribution of data within a normal distribution. Its practical applications span numerous fields, from quality control in manufacturing to analyzing financial markets and understanding test scores. The ability to quickly estimate probabilities and data ranges based on the mean and standard deviation is invaluable for efficient data interpretation. This rule allows for immediate estimations, avoiding more complex calculations involving probability density functions and integral calculus.
Overview: What This Article Covers:
This article delves into the core aspects of the Empirical Rule, providing a clear definition, explaining its underlying formula, showcasing real-world examples, and outlining its practical applications across various fields. Readers will gain a comprehensive understanding of this essential statistical tool, including its limitations and when it's appropriate to use.
The Research and Effort Behind the Insights:
This article draws upon established statistical principles and widely accepted methodologies. The explanations are supported by illustrative examples and clear visual representations to ensure a comprehensive understanding of the Empirical Rule. The goal is to present a clear and accessible guide for readers of all backgrounds.
Key Takeaways:
- Definition and Core Concepts: A precise definition of the Empirical Rule and its core principles related to normal distributions.
- Formula and Calculations: Understanding the mathematical basis of the rule and how to perform calculations using the mean and standard deviation.
- Practical Applications: Real-world examples demonstrating the Empirical Rule's use in various fields, such as quality control and standardized testing.
- Limitations and Considerations: A discussion of the situations where the Empirical Rule might not be applicable or require caution.
Smooth Transition to the Core Discussion:
Now that we understand the importance of the Empirical Rule, let's explore its core components, beginning with a precise definition.
Exploring the Key Aspects of the Empirical Rule:
1. Definition and Core Concepts:
The Empirical Rule states that for a normal distribution, approximately:
- 68% of the data falls within one standard deviation of the mean.
- 95% of the data falls within two standard deviations of the mean.
- 99.7% of the data falls within three standard deviations of the mean.
This rule is based on the properties of the normal distribution curve, a symmetrical bell-shaped curve with the mean, median, and mode coinciding at its center. The standard deviation measures the spread or dispersion of the data around the mean. A larger standard deviation indicates a wider spread, while a smaller standard deviation indicates a narrower spread.
2. Formula and Calculations:
The Empirical Rule doesn't have a single formula, per se. Instead, it relies on the mean (µ) and standard deviation (σ) of the data set. To apply the rule, you need to calculate these descriptive statistics. Once you have these values, you can determine the data ranges corresponding to the percentages:
- Within one standard deviation: (µ - σ) to (µ + σ)
- Within two standard deviations: (µ - 2σ) to (µ + 2σ)
- Within three standard deviations: (µ - 3σ) to (µ + 3σ)
3. Applications Across Industries:
The Empirical Rule has wide-ranging applications:
-
Quality Control: In manufacturing, the rule helps determine whether a production process is meeting quality standards. If the measurements of a product consistently fall within the expected range based on the Empirical Rule, the process is considered stable. Conversely, data points falling outside these ranges signal potential problems requiring investigation.
-
Standardized Testing: Standardized test scores are often normally distributed. The Empirical Rule helps interpret individual scores relative to the mean and standard deviation of the entire test population. For instance, knowing the mean and standard deviation, one can determine the percentage of students scoring within a certain range.
-
Finance: Financial analysts often use the Empirical Rule to analyze investment returns. By understanding the distribution of returns, they can assess risk and make informed investment decisions. For instance, understanding the probability of returns falling within a certain range can be crucial in risk management.
-
Healthcare: In healthcare, the rule can be used to analyze patient data, such as blood pressure or cholesterol levels. This helps identify individuals who fall outside the normal range and may require medical attention.
-
Agriculture: Yields in agriculture can be analyzed using this rule. Farmers can assess whether their harvests are within acceptable ranges, aiding in planning and resource allocation.
4. Challenges and Solutions:
The primary challenge lies in the assumption of normality. The Empirical Rule is only accurate for data that follows a normal distribution. If the data is significantly skewed or has outliers, the rule's predictions might be inaccurate. Therefore, it's crucial to assess the normality of your data before applying the Empirical Rule. Techniques like histograms, Q-Q plots, and normality tests can help determine if the data is approximately normally distributed. If normality is not met, other statistical methods should be considered.
5. Impact on Innovation:
The Empirical Rule's simplicity facilitates quick data interpretation, leading to faster decision-making across various fields. This allows for improved efficiency in quality control, enhanced risk assessment in finance, and more effective resource allocation in agriculture. Its ease of understanding also makes it a valuable educational tool, simplifying complex statistical concepts for a broader audience.
Exploring the Connection Between Z-scores and the Empirical Rule:
The Empirical Rule and Z-scores are intrinsically linked. A Z-score represents the number of standard deviations a data point is away from the mean. The formula for calculating a Z-score is:
Z = (x - µ) / σ
Where:
- x = individual data point
- µ = mean
- σ = standard deviation
The Empirical Rule's percentages directly correspond to specific Z-score ranges:
- 68% corresponds to Z-scores between -1 and +1.
- 95% corresponds to Z-scores between -2 and +2.
- 99.7% corresponds to Z-scores between -3 and +3.
This connection underscores the rule's power: by simply calculating a Z-score, one can quickly estimate the probability of observing a particular data point within a normal distribution.
Key Factors to Consider:
-
Roles and Real-World Examples: The Z-score acts as a standardized measure, allowing for comparisons across different datasets with varying means and standard deviations. For instance, comparing test scores from different exams, where the scales might be different, becomes straightforward using Z-scores.
-
Risks and Mitigations: The main risk is misinterpreting the rule when data isn't normally distributed. Mitigating this involves rigorously checking data normality before application.
-
Impact and Implications: The use of Z-scores and the Empirical Rule enhances data analysis efficiency and allows for clearer insights, improving decision-making across diverse sectors.
Conclusion: Reinforcing the Connection:
The strong relationship between Z-scores and the Empirical Rule highlights its effectiveness in simplifying data analysis within normal distributions. By understanding Z-scores, one gains a deeper appreciation for the practical utility and limitations of the Empirical Rule.
Further Analysis: Examining Outliers and Their Impact:
Outliers, data points far removed from the majority of the data, can significantly affect the accuracy of the Empirical Rule. Their presence can skew the mean and standard deviation, leading to inaccurate predictions. Identifying and handling outliers appropriately (e.g., through investigation to ensure data accuracy or removal if justified) is crucial for reliable application of the rule. Techniques like box plots can help visualize potential outliers.
FAQ Section: Answering Common Questions About the Empirical Rule:
Q: What is the Empirical Rule?
A: The Empirical Rule, or 68-95-99.7 rule, is a statistical guideline estimating the proportion of data falling within one, two, and three standard deviations from the mean in a normal distribution.
Q: How is the Empirical Rule applied in real-world scenarios?
A: It's used across various fields, including quality control, standardized testing, finance, and healthcare, to assess data distribution, identify outliers, and make informed decisions.
Q: What are the limitations of the Empirical Rule?
A: The rule only applies to normally distributed data. Significant skewness or the presence of outliers can render its predictions inaccurate.
Q: What if my data isn't normally distributed?
A: If your data isn't normally distributed, the Empirical Rule is not applicable. Other statistical methods, such as non-parametric methods, should be used.
Practical Tips: Maximizing the Benefits of the Empirical Rule:
- Verify Normality: Always assess your data's normality before applying the Empirical Rule.
- Calculate Mean and Standard Deviation: Accurately calculate the mean and standard deviation of your dataset.
- Interpret Results Cautiously: The Empirical Rule provides approximations, not exact values.
Final Conclusion: Wrapping Up with Lasting Insights:
The Empirical Rule serves as a powerful and easily accessible tool for quickly assessing data distributions within normal datasets. By understanding its definition, formula, applications, and limitations, one can significantly improve their data analysis capabilities. While simple in its structure, this rule offers substantial insights that enhance decision-making and problem-solving across numerous disciplines. Remember to always verify data normality before using it, thus ensuring its accurate and effective application.
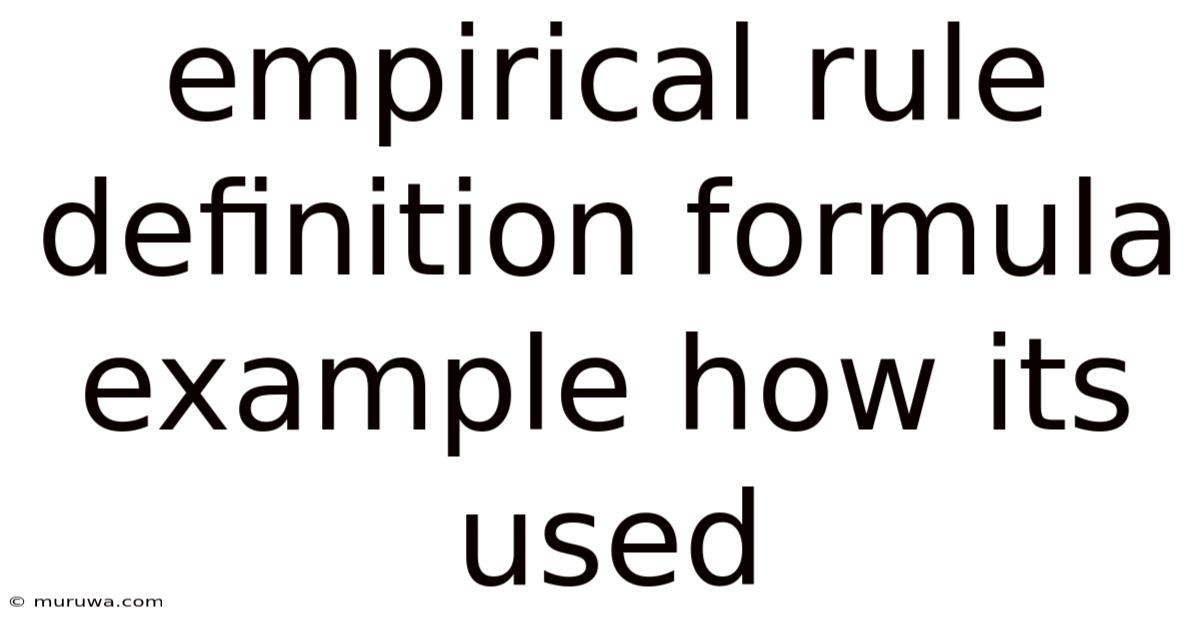
Thank you for visiting our website wich cover about Empirical Rule Definition Formula Example How Its Used. We hope the information provided has been useful to you. Feel free to contact us if you have any questions or need further assistance. See you next time and dont miss to bookmark.
Also read the following articles
Article Title | Date |
---|---|
Why Is My Credit Score Not Showing Up On Experian | Apr 10, 2025 |
When Does Suncoast Credit Union Open | Apr 10, 2025 |
How Long Does It Take To Get A Fico Score After Getting A Credit Card | Apr 10, 2025 |
Which Is Better Debt Relief Or Debt Consolidation | Apr 10, 2025 |
What Is The Billing Cycle Of A Credit Card | Apr 10, 2025 |