Chain Ladder Method Clm Definition Steps To Apply It
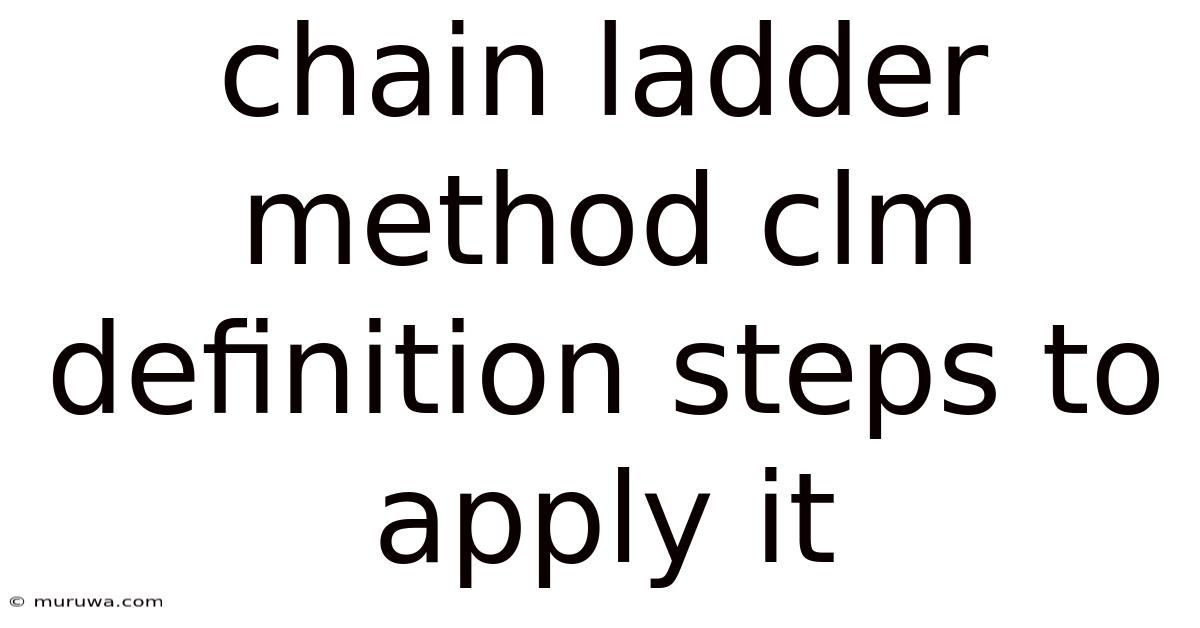
Discover more detailed and exciting information on our website. Click the link below to start your adventure: Visit Best Website meltwatermedia.ca. Don't miss out!
Table of Contents
Unveiling the Chain Ladder Method: A Comprehensive Guide to Loss Reserving
What if accurate loss reserving could significantly improve insurance company solvency and strategic decision-making? The Chain Ladder Method (CLM), a cornerstone of actuarial science, offers a robust and widely used approach to achieve just that.
Editor’s Note: This article provides a comprehensive overview of the Chain Ladder Method (CLM) for loss reserving, updated with the latest insights and practical applications. It's designed for actuaries, insurance professionals, and anyone interested in understanding this crucial technique.
Why the Chain Ladder Method Matters:
The Chain Ladder Method is a fundamental technique for estimating the ultimate cost of insurance claims. Accurate loss reserving is crucial for several reasons:
- Financial Reporting: Insurers need to accurately reflect their liabilities on their balance sheets. Underestimating reserves can lead to insolvency, while overestimating them can hinder profitability.
- Regulatory Compliance: Insurance regulators require accurate loss reserving to ensure solvency and protect policyholders.
- Strategic Planning: Accurate loss reserves inform business decisions, such as pricing strategies, reinsurance purchases, and capital allocation.
- Investment Decisions: Knowing the likely future claims payout allows for more effective investment strategies to meet those obligations.
Overview: What This Article Covers:
This article provides a detailed explanation of the Chain Ladder Method, covering its definition, underlying assumptions, step-by-step application, advantages, limitations, and potential refinements. We will also explore its practical applications and considerations for different types of insurance.
The Research and Effort Behind the Insights:
This article draws upon extensive research from actuarial textbooks, industry publications, and practical experience in loss reserving. The methodology and explanations are grounded in established actuarial principles and best practices.
Key Takeaways:
- Definition and Core Concepts: A clear definition of the Chain Ladder Method and its underlying assumptions.
- Step-by-Step Application: A detailed guide on how to apply the CLM, including data preparation and calculation techniques.
- Advantages and Limitations: An objective assessment of the strengths and weaknesses of the CLM.
- Refined Approaches: Exploration of extensions and modifications to address some of the CLM's limitations.
- Practical Applications and Considerations: Real-world examples and considerations for different insurance lines.
Smooth Transition to the Core Discussion:
Now that we understand the importance of accurate loss reserving and the central role of the CLM, let's delve into the specifics of this powerful technique.
Exploring the Key Aspects of the Chain Ladder Method:
1. Definition and Core Concepts:
The Chain Ladder Method is a non-parametric statistical model used to estimate the ultimate incurred losses for a portfolio of insurance claims. It's based on the historical development of claims over time, assuming that the pattern of development will continue into the future. The core assumption is that the age-to-age development factors remain relatively stable over time. These factors represent the proportion by which claims increase from one development period to the next.
2. Data Requirements:
The CLM requires a run-off triangle, also known as a development triangle or a loss development triangle. This is a matrix showing cumulative incurred losses for each accident year and development year. An accident year represents the year in which the event causing the claim occurred. A development year refers to the time elapsed since the accident year. For example:
Accident Year | Dev. Year 0 | Dev. Year 1 | Dev. Year 2 | Dev. Year 3 | Dev. Year 4 | Dev. Year 5 |
---|---|---|---|---|---|---|
2018 | 100,000 | 120,000 | 135,000 | 140,000 | 142,000 | 143,000 |
2019 | 110,000 | 132,000 | 148,500 | 154,000 | 156,000 | |
2020 | 125,000 | 150,000 | 168,750 | 175,000 | ||
2021 | 130,000 | 156,000 | 175,500 | |||
2022 | 140,000 | 168,000 |
3. Calculating Age-to-Age Factors:
The first step is to calculate the age-to-age development factors. This is done by dividing the cumulative losses at each development year by the cumulative losses at the preceding development year. For example, the development factor from Year 0 to Year 1 for Accident Year 2018 is 120,000 / 100,000 = 1.2. These factors are then typically averaged across all accident years to obtain a single development factor for each age-to-age transition.
4. Projecting Future Losses:
Once the age-to-age factors are calculated and averaged, they are applied to the latest cumulative loss figures for each accident year to project the ultimate incurred losses. For example, if the average factor from Year 4 to Year 5 is 1.007, and the cumulative loss for Accident Year 2020 at Year 4 is 156,000, then the projected ultimate loss for Accident Year 2020 is 156,000 * 1.007 = 157,092. This process is repeated for all accident years with incomplete data.
5. Summing Projected Ultimate Losses:
Finally, the projected ultimate losses for each accident year are summed to obtain the total projected ultimate loss for the entire portfolio.
Exploring the Connection Between Data Quality and Chain Ladder Accuracy:
The accuracy of the Chain Ladder Method is heavily reliant on the quality of the underlying data. Inaccurate or incomplete data can lead to significant errors in the loss reserve estimates. Several key factors need consideration:
- Data Completeness: The run-off triangle should ideally be as complete as possible, with minimal missing data.
- Data Accuracy: Errors in the reported claims data will directly affect the accuracy of the CLM results. Robust data validation and reconciliation processes are crucial.
- Data Consistency: Consistent data reporting methods across accident years are essential to avoid biases in the development factors.
Key Factors to Consider:
-
Roles and Real-World Examples: The impact of data quality is readily visible in real-world applications. Inconsistent reporting of claims, for instance, can lead to inflated or deflated development factors, resulting in inaccurate reserve estimates.
-
Risks and Mitigations: The risk of inaccurate data can be mitigated through rigorous data quality checks, validation procedures, and the use of robust data management systems. Regular audits of the claims data can also identify and correct inconsistencies.
-
Impact and Implications: Inaccurate loss reserves can have serious financial consequences for insurers, potentially leading to regulatory penalties, insolvency, or impaired profitability.
Conclusion: Reinforcing the Connection:
The relationship between data quality and Chain Ladder accuracy is undeniable. Prioritizing data quality is not merely a good practice; it is a fundamental requirement for reliable loss reserving using the CLM.
Further Analysis: Examining Data Smoothing Techniques:
While the basic CLM is straightforward, the age-to-age factors are often volatile, especially with limited data. Smoothing techniques can be applied to improve the stability and reliability of the development factors. Common methods include:
- Moving Averages: These smooth out fluctuations by averaging development factors over several consecutive years.
- Weighted Averages: Similar to moving averages, but with weights assigned to different years to emphasize more recent data.
- Regression Analysis: Regression models can be used to fit a curve to the development factors, providing a smoother estimate.
FAQ Section: Answering Common Questions About the Chain Ladder Method:
-
What is the Chain Ladder Method? The Chain Ladder Method is a widely used actuarial technique for projecting the ultimate incurred losses on insurance claims. It uses historical claims data to estimate future development patterns.
-
What are the limitations of the Chain Ladder Method? The CLM relies on several key assumptions that may not always hold true in practice, such as the stability of age-to-age factors and the independence of development patterns across accident years.
-
How can I improve the accuracy of the Chain Ladder Method? Improving data quality, incorporating external information (e.g., economic trends), and using refined techniques like data smoothing or generalized linear models can improve accuracy.
-
What software is commonly used for Chain Ladder calculations? Many actuarial software packages offer dedicated functionalities for performing Chain Ladder analysis, including specialized statistical packages like R and SAS, as well as commercial actuarial software solutions.
Practical Tips: Maximizing the Benefits of the Chain Ladder Method:
-
Data Validation: Implement robust data validation and reconciliation processes to ensure data accuracy and consistency.
-
Sensitivity Analysis: Perform sensitivity analysis to assess the impact of different assumptions on the reserve estimates.
-
External Information: Incorporate external information, such as economic trends and regulatory changes, into the analysis.
-
Regular Monitoring: Regularly monitor and update the loss reserves as new data becomes available.
Final Conclusion: Wrapping Up with Lasting Insights:
The Chain Ladder Method is a valuable tool for insurance companies to estimate their future claims liabilities. While it has limitations, its simplicity and wide acceptance make it a crucial method for loss reserving. Understanding its assumptions, limitations, and potential refinements is essential for actuaries and insurance professionals to apply it effectively and responsibly. By improving data quality, employing appropriate smoothing techniques, and regularly monitoring the reserves, insurers can utilize the CLM to gain greater accuracy and confidence in their financial projections.
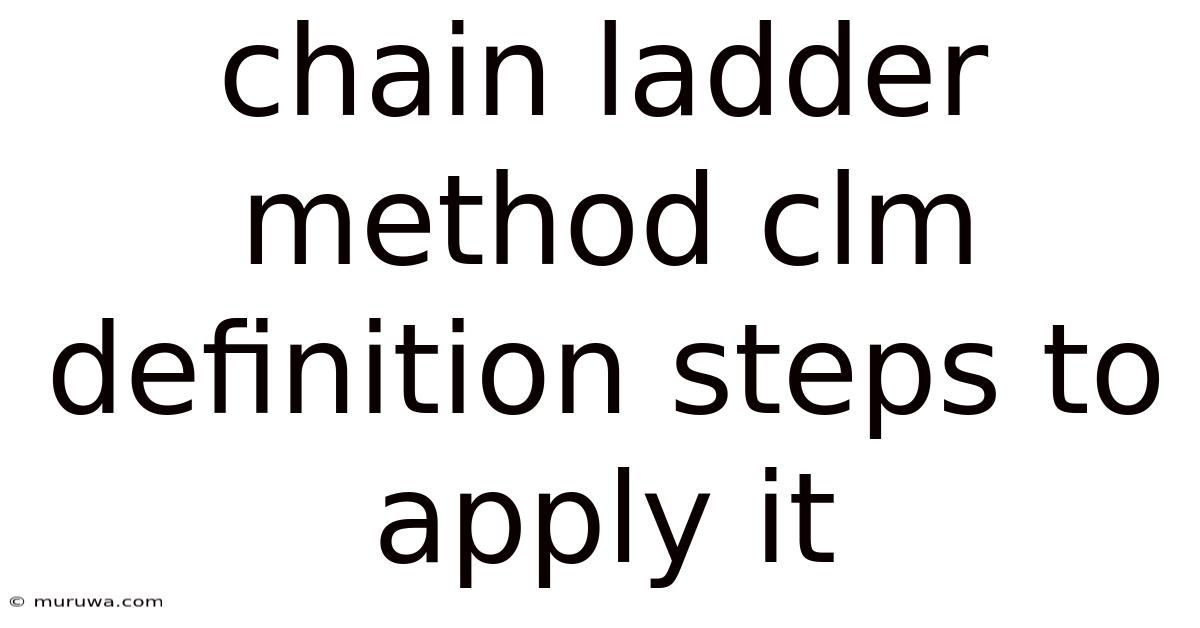
Thank you for visiting our website wich cover about Chain Ladder Method Clm Definition Steps To Apply It. We hope the information provided has been useful to you. Feel free to contact us if you have any questions or need further assistance. See you next time and dont miss to bookmark.
Also read the following articles
Article Title | Date |
---|---|
How To Apply For A Verve Credit Card | Mar 12, 2025 |
Clintonomics Definition | Mar 12, 2025 |
Closed Loop Mrp Definition | Mar 12, 2025 |
Why Is Roku Asking For My Credit Card | Mar 12, 2025 |
How Long Does It Take To Get Approved For A Tractor Supply Credit Card | Mar 12, 2025 |