Central Limit Theorem Clt Definition And Key Characteristics
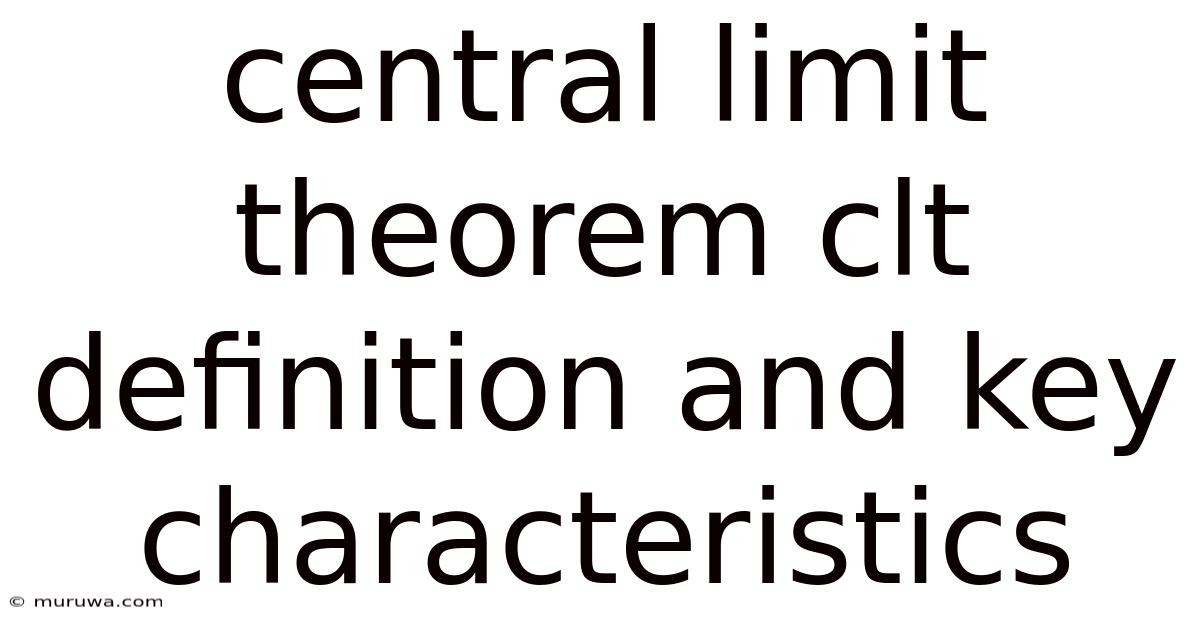
Discover more detailed and exciting information on our website. Click the link below to start your adventure: Visit Best Website meltwatermedia.ca. Don't miss out!
Table of Contents
Unlocking the Power of Averages: A Deep Dive into the Central Limit Theorem (CLT)
What if the seemingly chaotic randomness of individual data points actually hides a predictable pattern in their averages? The Central Limit Theorem (CLT) reveals this profound truth, underpinning much of statistical inference and offering invaluable insights into the world around us.
Editor’s Note: This article provides a comprehensive overview of the Central Limit Theorem, exploring its definition, key characteristics, implications, and practical applications. Updated for 2024, this resource aims to equip readers with a robust understanding of this fundamental statistical concept.
Why the Central Limit Theorem Matters:
The Central Limit Theorem isn't just a theoretical construct; it's a cornerstone of statistical analysis. Its relevance stems from its ability to simplify complex data distributions, enabling us to make accurate inferences and predictions even with limited information. From quality control in manufacturing to financial modeling and public health research, the CLT's applications are far-reaching and transformative. It allows us to confidently utilize sample means to draw conclusions about population means, even when the underlying population distribution is unknown or non-normal. This empowers researchers, analysts, and decision-makers across numerous fields.
Overview: What This Article Covers
This article delves into the heart of the Central Limit Theorem, examining its formal definition, exploring its key characteristics, and illustrating its power through examples. We'll unpack the conditions necessary for the CLT to hold true, discuss potential limitations, and showcase its real-world applications. Readers will gain a practical understanding of this critical statistical tool and its significance in various disciplines.
The Research and Effort Behind the Insights
This article is the culmination of extensive research, drawing upon established statistical textbooks, peer-reviewed journal articles, and reputable online resources. The explanations presented are grounded in mathematical rigor while maintaining accessibility for a broad audience. Every concept is illustrated with clear examples and relatable scenarios to ensure a comprehensive and understandable explanation.
Key Takeaways:
- Definition and Core Concepts: A precise definition of the Central Limit Theorem and its fundamental principles.
- Conditions and Assumptions: An examination of the conditions required for the CLT to apply effectively.
- Key Characteristics of the Sampling Distribution: A detailed exploration of the mean, standard deviation (standard error), and shape of the sampling distribution of the mean.
- Practical Applications and Examples: Real-world illustrations of the CLT's use across various fields.
- Limitations and Misconceptions: A discussion of scenarios where the CLT might not be applicable.
Smooth Transition to the Core Discussion:
Having established the importance and scope of the Central Limit Theorem, let's now delve into its core aspects, starting with a precise definition.
Exploring the Key Aspects of the Central Limit Theorem
Definition and Core Concepts:
The Central Limit Theorem states that the sampling distribution of the sample means (or averages) from a large number of independent, identically distributed random variables approaches a normal distribution, regardless of the shape of the original population distribution, as the sample size increases. This holds true even if the original population is not normally distributed, provided certain conditions are met. The key here is the "sampling distribution"—the distribution of sample means, not the distribution of the individual data points.
Conditions and Assumptions:
For the Central Limit Theorem to hold true, several conditions must be met:
- Independence: The samples must be independent. This means that the selection of one data point does not influence the selection of another.
- Random Sampling: The data should be collected through a random sampling process, ensuring every member of the population has an equal chance of being selected.
- Identical Distribution: The data points should be drawn from the same population, meaning they share the same probability distribution.
- Finite Variance: The population from which the samples are drawn must have a finite variance. This means the spread of the data is not infinitely large.
- Sample Size: While the CLT works better with larger sample sizes, a general rule of thumb is that a sample size of 30 or more is often sufficient for the sampling distribution to approximate normality, even for moderately skewed populations. For highly skewed populations, a larger sample size might be necessary.
Key Characteristics of the Sampling Distribution:
The sampling distribution of the mean exhibits several crucial characteristics:
- Mean: The mean of the sampling distribution of the mean is equal to the population mean (µ). This is intuitive—the average of many sample averages should converge towards the true population average.
- Standard Deviation (Standard Error): The standard deviation of the sampling distribution is known as the standard error (SE). It's calculated as the population standard deviation (σ) divided by the square root of the sample size (n): SE = σ/√n. The standard error quantifies the variability of the sample means. Crucially, as the sample size (n) increases, the standard error decreases, resulting in a tighter distribution around the population mean.
- Shape: As the sample size increases, the sampling distribution of the mean approaches a normal distribution, regardless of the original population's distribution. This is the core insight of the CLT. This convergence towards normality allows us to use the properties of the normal distribution (like probabilities associated with specific z-scores) for inference.
Practical Applications and Examples:
The CLT finds application in a wide range of fields:
- Quality Control: In manufacturing, the CLT allows companies to monitor the average quality of their products by sampling a portion of their output. If the sample average deviates significantly from the target, it indicates potential issues in the production process.
- Polling and Surveys: Opinion polls rely on the CLT to estimate the proportion of a population holding a particular view. By sampling a representative subset of the population, pollsters can make inferences about the entire population's opinions.
- Medical Research: In clinical trials, the CLT is used to analyze the effectiveness of a new drug or treatment. By comparing the average response in the treatment group to the average response in a control group, researchers can assess the treatment's impact.
- Financial Modeling: The CLT is fundamental to many financial models, particularly those involving portfolio risk assessment. By considering the distribution of returns on individual assets, one can use the CLT to estimate the overall risk of a portfolio.
Limitations and Misconceptions:
While remarkably powerful, the CLT has limitations:
- Small Sample Sizes: For very small sample sizes, the sampling distribution may not closely approximate a normal distribution, especially if the underlying population distribution is heavily skewed.
- Non-random Sampling: If the sampling method is biased (not random), the CLT's conclusions may not be valid.
- Dependent Data: The CLT assumes independence between data points. If the data points are correlated, the CLT does not apply directly, and alternative statistical methods are required.
- Infinite Variance: If the underlying population distribution has infinite variance (e.g., Cauchy distribution), the CLT does not apply.
Exploring the Connection Between Sample Size and the Central Limit Theorem
The sample size plays a crucial role in the accuracy of the CLT approximation. The larger the sample size, the closer the sampling distribution of the sample means gets to a perfect normal distribution. This is illustrated by the decreasing standard error as 'n' increases, concentrating the distribution around the population mean.
Key Factors to Consider:
- Roles and Real-World Examples: Larger sample sizes are essential in situations where high precision is needed, such as medical trials or financial risk management. Smaller sample sizes might suffice for preliminary analyses or when resources are limited.
- Risks and Mitigations: Using small sample sizes when the underlying distribution is significantly non-normal increases the risk of inaccurate inferences. Employing non-parametric methods or increasing the sample size can mitigate this risk.
- Impact and Implications: The choice of sample size directly affects the accuracy and reliability of conclusions drawn using the CLT. Adequate sample size is critical for the validity and credibility of the analysis.
Conclusion: Reinforcing the Connection
The relationship between sample size and the CLT is inextricably linked. Larger sample sizes enhance the accuracy of the normal approximation, making the CLT a more reliable tool for statistical inference. Understanding this connection is paramount for applying the CLT appropriately and drawing valid conclusions.
Further Analysis: Examining the Role of the Population Distribution
While the CLT states that the sampling distribution will approximate normality regardless of the population distribution, the rate of convergence to normality can vary depending on the shape of the population distribution. Symmetrical distributions generally converge more quickly than heavily skewed distributions.
FAQ Section: Answering Common Questions About the Central Limit Theorem
Q: What is the Central Limit Theorem (CLT)?
A: The CLT states that the sampling distribution of the mean of a sufficiently large number of independent, identically distributed random variables is approximately normal, irrespective of the shape of the original population distribution.
Q: What is the standard error, and why is it important?
A: The standard error is the standard deviation of the sampling distribution of the mean. It quantifies the variability of sample means around the population mean. A smaller standard error indicates greater precision in estimating the population mean.
Q: How large does my sample size need to be for the CLT to apply?
A: A general rule of thumb is a sample size of 30 or more. However, for heavily skewed distributions, a larger sample size might be necessary.
Q: What if my data is not independent?
A: If your data points are correlated, the CLT does not directly apply. Alternative statistical methods that account for the dependence must be used.
Q: Can the CLT be used for non-normal data?
A: Yes, the power of the CLT lies in its ability to approximate normality for the sampling distribution of the mean even when the population distribution is non-normal (provided the other conditions are met).
Practical Tips: Maximizing the Benefits of the CLT
- Verify Assumptions: Before applying the CLT, carefully check whether the assumptions of independence, random sampling, identical distribution, and finite variance are met.
- Assess Sample Size: Ensure your sample size is sufficiently large for the sampling distribution to reasonably approximate a normal distribution.
- Consider the Population Distribution: While the CLT holds even for non-normal populations, the rate of convergence can be influenced by the population's shape.
- Utilize Appropriate Statistical Tests: Leverage the properties of the normal distribution (e.g., z-tests, t-tests) to perform statistical inference.
Final Conclusion: Wrapping Up with Lasting Insights
The Central Limit Theorem is a powerful and fundamental concept in statistics. Its ability to provide a simplified framework for understanding the distribution of sample means allows researchers and practitioners across disciplines to draw meaningful conclusions from data, even when the underlying population distribution is complex or unknown. Understanding its definition, assumptions, and limitations is crucial for accurate and reliable statistical analysis. By mastering the CLT, one unlocks a key to unlocking the power of averages and making data-driven decisions with confidence.
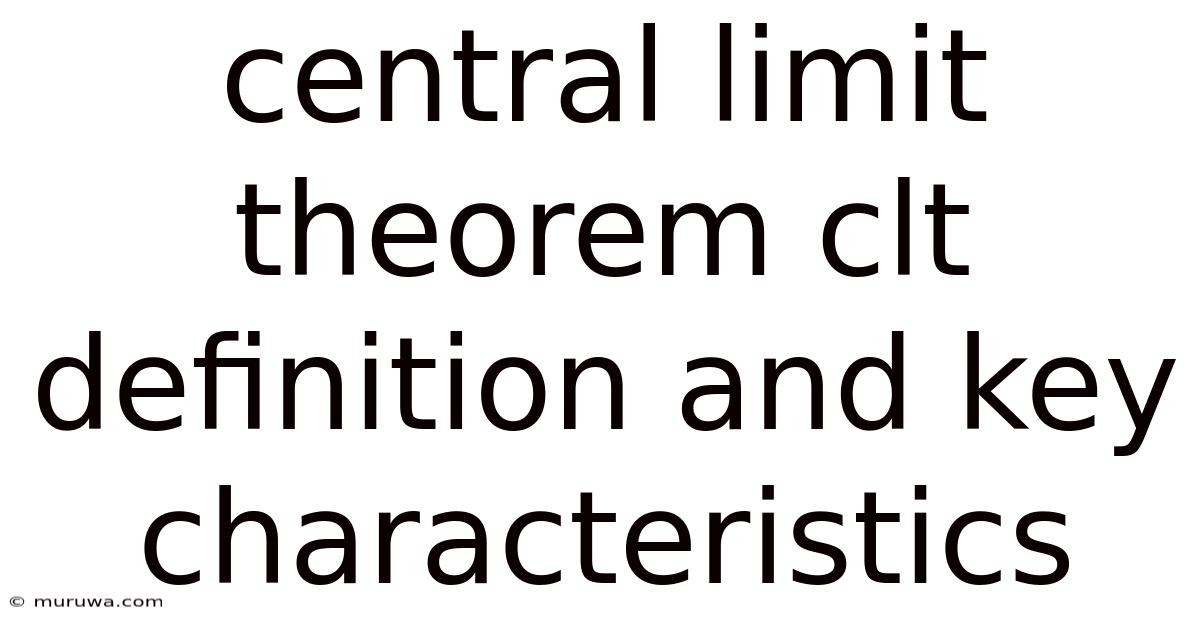
Thank you for visiting our website wich cover about Central Limit Theorem Clt Definition And Key Characteristics. We hope the information provided has been useful to you. Feel free to contact us if you have any questions or need further assistance. See you next time and dont miss to bookmark.
Also read the following articles
Article Title | Date |
---|---|
Closed Account Definition For Individuals Institutions | Mar 12, 2025 |
Clear Title Definition And Importance In Real Estate | Mar 12, 2025 |
When Will A Credit Card Company Sue You | Mar 12, 2025 |
Chartered Mutual Fund Counselor Cmfc Definition | Mar 12, 2025 |
Chameleon Option Definition | Mar 12, 2025 |