Box Jenkins Model Definition Uses Timeframes And Forecasting
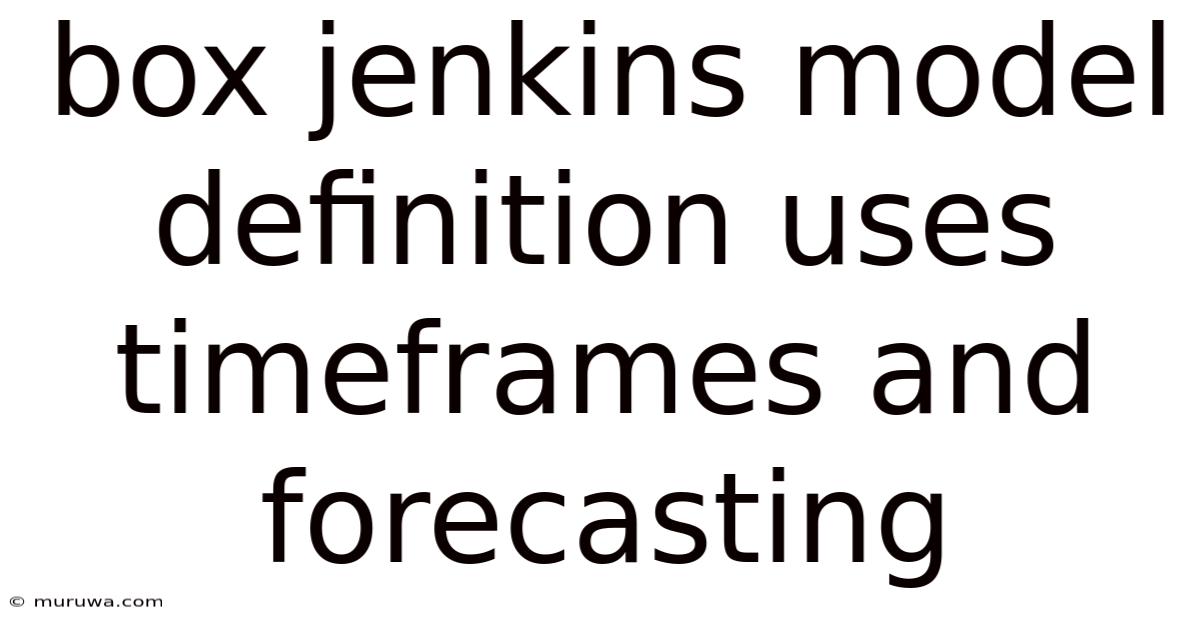
Discover more detailed and exciting information on our website. Click the link below to start your adventure: Visit Best Website meltwatermedia.ca. Don't miss out!
Table of Contents
Unlocking the Power of Prediction: A Deep Dive into Box-Jenkins Modeling
What if we could accurately predict the future, mitigating risks and maximizing opportunities? The Box-Jenkins methodology offers a powerful framework for time series forecasting, enabling businesses and researchers to anticipate trends and make data-driven decisions.
Editor’s Note: This article provides a comprehensive overview of Box-Jenkins modeling, exploring its definition, uses, relevant timeframes, and forecasting capabilities. The information presented is intended to be informative and accessible to a broad audience, offering both theoretical understanding and practical applications.
Why Box-Jenkins Modeling Matters
In a world driven by data, the ability to accurately forecast future trends is invaluable. Box-Jenkins modeling, a statistical approach to time series analysis, provides a robust framework for identifying patterns, understanding underlying processes, and generating reliable forecasts. Its applications span various fields, including finance (predicting stock prices), economics (forecasting economic growth), meteorology (weather prediction), and supply chain management (optimizing inventory levels). Understanding and utilizing Box-Jenkins models can provide a competitive edge by enabling proactive decision-making and resource allocation.
Overview: What This Article Covers
This article will delve into the core principles of Box-Jenkins modeling, exploring its foundational concepts, step-by-step process, suitable timeframes, and practical applications. Readers will gain a thorough understanding of how this powerful technique can be used to generate accurate forecasts and inform strategic planning. We will also examine the limitations and considerations when employing this method.
The Research and Effort Behind the Insights
This article draws upon extensive research, incorporating concepts from leading statistical textbooks, peer-reviewed publications, and real-world case studies. The explanations provided are supported by evidence and aim to provide a clear, accurate, and accessible understanding of Box-Jenkins modeling for a diverse readership.
Key Takeaways:
- Definition and Core Concepts: A precise explanation of Box-Jenkins methodology and its underlying principles.
- Model Identification and Selection: A detailed walkthrough of the process of identifying and selecting the appropriate ARIMA model.
- Parameter Estimation: An explanation of how model parameters are estimated and validated.
- Forecasting and Evaluation: Techniques for generating forecasts and assessing their accuracy.
- Timeframe Considerations: Guidance on selecting appropriate timeframes for different applications.
- Advantages and Limitations: An honest assessment of the strengths and weaknesses of Box-Jenkins modeling.
Smooth Transition to the Core Discussion:
With a foundational understanding of the significance of Box-Jenkins modeling, let's delve into its core aspects, exploring its methodology and practical applications.
Exploring the Key Aspects of Box-Jenkins Modeling
1. Definition and Core Concepts:
The Box-Jenkins methodology, named after George Box and Gwilym Jenkins, is a powerful statistical technique used for analyzing and forecasting time series data. A time series is a sequence of data points collected over time. The Box-Jenkins approach focuses on building autoregressive integrated moving average (ARIMA) models, which capture the underlying patterns and dependencies within the data.
ARIMA models are characterized by three parameters:
- p (Autoregressive order): Represents the number of lagged values of the time series used as predictors. An AR(p) model suggests that the current value is a linear combination of past values.
- d (Differencing order): Represents the number of times the time series needs to be differenced to achieve stationarity. Stationarity implies that the statistical properties of the time series (mean, variance, autocorrelation) remain constant over time. Differencing is a crucial step in preparing the data for modeling.
- q (Moving average order): Represents the number of lagged forecast errors used as predictors. An MA(q) model suggests that the current error is a linear combination of past errors.
An ARIMA(p, d, q) model combines these three components to capture the complex dynamics of the time series.
2. Model Identification and Selection:
Identifying the appropriate ARIMA model is a crucial step. This involves analyzing the autocorrelation function (ACF) and partial autocorrelation function (PACF) plots of the differenced time series. These plots reveal the correlation between the time series and its lagged values, helping determine the appropriate values of 'p' and 'q'. The 'd' value is determined by the degree of differencing needed to achieve stationarity.
3. Parameter Estimation:
Once the ARIMA model is identified, its parameters (coefficients for AR and MA terms) are estimated using statistical methods such as maximum likelihood estimation. These estimates quantify the strength and direction of the relationships between the current value and its past values and errors.
4. Forecasting and Evaluation:
Once the model is fitted, forecasts can be generated for future time periods. The accuracy of these forecasts is evaluated using metrics such as mean absolute error (MAE), root mean squared error (RMSE), and mean absolute percentage error (MAPE). These metrics assess the difference between the forecasted values and the actual values. Residual analysis is also crucial to check if the model assumptions are met and the residuals are random.
5. Timeframe Considerations:
The appropriate timeframe for Box-Jenkins modeling depends on the specific application and the nature of the time series. Short-term forecasts might use daily or weekly data, while long-term forecasts might utilize monthly or yearly data. The choice of timeframe is influenced by the frequency of data collection and the forecasting horizon.
6. Advantages and Limitations:
Advantages:
- Flexibility: Handles various types of time series data, including stationary and non-stationary series.
- Accuracy: Can generate relatively accurate forecasts, especially for short to medium-term horizons.
- Interpretability: Provides insights into the underlying patterns and dependencies within the time series.
Limitations:
- Stationarity Assumption: Requires data to be stationary or made stationary through differencing.
- Model Complexity: Selecting the appropriate model can be challenging, requiring expertise in time series analysis.
- Sensitivity to Outliers: Outliers can significantly affect model estimation and forecasting accuracy.
- Limited Handling of External Factors: Primarily focuses on the inherent patterns within the time series, ignoring external factors that might influence the data.
Exploring the Connection Between Data Preprocessing and Box-Jenkins Modeling
Data preprocessing plays a vital role in the success of Box-Jenkins modeling. Accurate and reliable forecasts depend heavily on the quality and preparation of the input data. Let's explore this crucial connection.
Key Factors to Consider:
1. Roles and Real-World Examples:
Data preprocessing involves several steps, including:
- Data Cleaning: Handling missing values, outliers, and inconsistencies in the data. For example, in financial forecasting, outliers caused by unexpected market events need careful handling.
- Data Transformation: Applying transformations like logarithmic or Box-Cox transformations to stabilize variance and ensure normality. This is crucial for financial time series that often exhibit heteroscedasticity (non-constant variance).
- Data Smoothing: Removing noise and irregularities from the data using techniques like moving averages. This is beneficial when dealing with noisy sensor data.
- Stationarity Check and Differencing: Confirming the stationarity of the time series and applying differencing as necessary to achieve stationarity. This step is essential for applying ARIMA models.
2. Risks and Mitigations:
Ignoring data preprocessing steps can lead to inaccurate model estimations and unreliable forecasts. For instance, not handling outliers can result in biased model parameters and poor forecast accuracy. Similarly, failure to address non-stationarity will render the ARIMA model inappropriate.
Mitigation strategies include thorough data inspection, appropriate transformation techniques, robust outlier detection and treatment, and careful consideration of differencing techniques.
3. Impact and Implications:
Proper data preprocessing significantly improves the accuracy and reliability of Box-Jenkins forecasts. Accurate forecasts translate to better decision-making, optimized resource allocation, and reduced risks in various applications. Conversely, neglecting data preprocessing can lead to inaccurate forecasts with significant economic or operational consequences.
Conclusion: Reinforcing the Connection
The relationship between data preprocessing and Box-Jenkins modeling is synergistic. Effective data preprocessing ensures the model's assumptions are met, enhancing the reliability and accuracy of the resulting forecasts. By addressing data quality issues and ensuring stationarity, businesses can leverage the power of Box-Jenkins modeling to make informed decisions and improve their operations.
Further Analysis: Examining Stationarity in Greater Detail
Stationarity, a crucial assumption in Box-Jenkins modeling, means that the statistical properties of the time series remain constant over time. Non-stationary data often exhibits trends, seasonality, or cyclical patterns, violating the assumption of constant mean and variance. Addressing non-stationarity is vital for accurate modeling. Techniques like differencing are commonly employed to transform non-stationary data into stationary data. The order of differencing ('d' in ARIMA) is determined based on the characteristics of the ACF and PACF plots. Achieving stationarity is crucial for reliable model fitting and forecasting.
FAQ Section: Answering Common Questions About Box-Jenkins Modeling
Q: What is the difference between AR, I, and MA components in ARIMA models?
A: AR (Autoregressive) models use past values of the time series to predict the current value. I (Integrated) represents the order of differencing needed to achieve stationarity. MA (Moving Average) models use past forecast errors to predict the current value.
Q: How do I determine the appropriate ARIMA model order (p, d, q)?
A: Analyze the ACF and PACF plots of the differenced time series. The patterns in these plots suggest appropriate values for p and q. The value of d is determined by the order of differencing needed for stationarity.
Q: What are some common evaluation metrics for Box-Jenkins forecasts?
A: Common metrics include MAE (Mean Absolute Error), RMSE (Root Mean Squared Error), MAPE (Mean Absolute Percentage Error), and R-squared.
Q: Can Box-Jenkins models handle seasonality?
A: Yes, seasonal ARIMA (SARIMA) models extend the ARIMA framework to explicitly incorporate seasonal patterns.
Q: What software can be used for Box-Jenkins modeling?
A: Popular software packages include R, Python (with libraries like statsmodels), and specialized statistical software like SPSS and SAS.
Practical Tips: Maximizing the Benefits of Box-Jenkins Modeling
- Thorough Data Exploration: Begin with a comprehensive analysis of your time series data, identifying patterns, trends, and potential issues.
- Appropriate Data Preprocessing: Apply necessary transformations, handle missing values, and address non-stationarity.
- Model Selection: Carefully select the ARIMA model order based on ACF and PACF analysis.
- Model Validation: Use appropriate metrics to evaluate model accuracy and ensure assumptions are met.
- Iterative Approach: Model building is often iterative. Refine the model and parameters as needed based on evaluation results.
Final Conclusion: Wrapping Up with Lasting Insights
Box-Jenkins modeling offers a powerful framework for time series analysis and forecasting. By understanding its principles, employing appropriate data preprocessing, and carefully selecting and evaluating models, businesses and researchers can unlock valuable insights and make data-driven decisions. While the methodology has limitations, its flexibility and ability to generate accurate forecasts make it a valuable tool across various disciplines. Continued advancements in time series analysis and computing power will only enhance the capabilities and applications of Box-Jenkins modeling in the years to come.
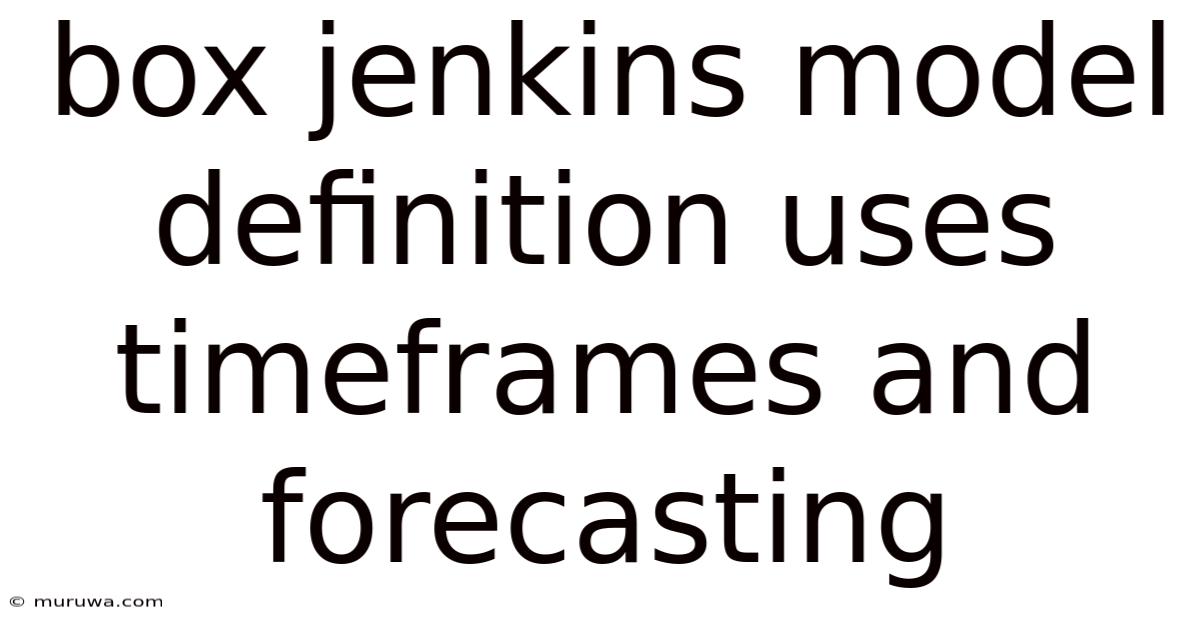
Thank you for visiting our website wich cover about Box Jenkins Model Definition Uses Timeframes And Forecasting. We hope the information provided has been useful to you. Feel free to contact us if you have any questions or need further assistance. See you next time and dont miss to bookmark.
Also read the following articles
Article Title | Date |
---|---|
Branch Manager Definition Job Description And Salary | Apr 22, 2025 |
Bosnia Herzegovina Convertible Mark Bam Definition | Apr 22, 2025 |
Block Trade Definition How It Works And Example | Apr 22, 2025 |
What Is A Good Roi For Investment Property | Apr 22, 2025 |
What Is An Investment Consultant | Apr 22, 2025 |