Beneish Model Definition Examples M Score Calculation
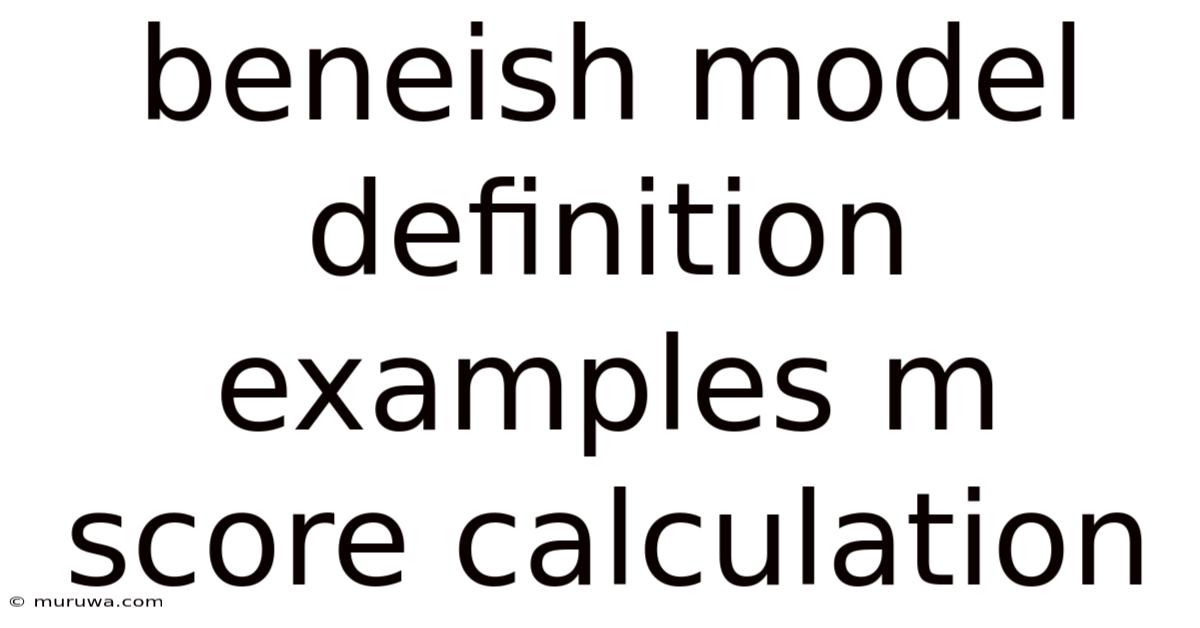
Discover more detailed and exciting information on our website. Click the link below to start your adventure: Visit Best Website meltwatermedia.ca. Don't miss out!
Table of Contents
Unveiling Financial Fraud: A Deep Dive into the Beneish Model
What if the early detection of financial statement fraud could significantly mitigate investment risks? The Beneish Model, a powerful predictive tool, offers just that, providing insights into the likelihood of a company manipulating its financial reports.
Editor’s Note: This article provides a comprehensive overview of the Beneish Model, including its definition, calculation, interpretation, and limitations. It offers practical examples and explores its applications in fraud detection and risk assessment. The information presented is for educational purposes and should not be considered financial advice.
Why the Beneish Model Matters:
The Beneish Model holds significant relevance in today's complex financial landscape. Accurate financial reporting is the cornerstone of investor confidence and market stability. However, instances of financial statement fraud continue to occur, leading to substantial financial losses and reputational damage. The Beneish Model provides a quantitative approach to assess the likelihood of such fraudulent activities, aiding investors, auditors, and credit analysts in making informed decisions. Its application extends beyond mere fraud detection; it helps in proactively managing risk and identifying potentially problematic companies before significant damage occurs. Understanding this model is crucial for navigating the intricacies of financial analysis and mitigating investment risks.
Overview: What This Article Covers:
This in-depth analysis explores the Beneish Model's core components, providing a step-by-step guide to its calculation and interpretation. We will dissect each variable, illustrate the calculation with practical examples, and discuss the limitations of the model. Furthermore, we will examine the relationship between the M-score and the probability of financial statement manipulation, offering actionable insights for risk management.
The Research and Effort Behind the Insights:
This article is the result of extensive research, drawing upon academic literature, empirical studies on the Beneish Model, and practical applications in financial analysis. The information presented is supported by credible sources and aims to provide a clear, accurate, and insightful understanding of this vital tool for fraud detection.
Key Takeaways:
- Definition and Core Concepts: A clear explanation of the Beneish Model and its underlying principles.
- M-Score Calculation: A step-by-step guide to calculating the M-score using publicly available financial data.
- Interpretation of the M-Score: Understanding the significance of the M-score and its implications for fraud detection.
- Examples and Case Studies: Real-world applications of the Beneish Model and its effectiveness in identifying fraudulent activities.
- Limitations and Considerations: Acknowledging the inherent limitations and potential biases of the model.
Smooth Transition to the Core Discussion:
Having established the importance and scope of the Beneish Model, let's delve into its core components and mechanics.
Exploring the Key Aspects of the Beneish Model:
The Beneish Model is a multivariate discriminant analysis model designed to predict the likelihood of financial statement manipulation. It uses eight financial ratios as predictors, creating a composite score known as the M-score. A higher M-score suggests a greater probability of earnings manipulation.
1. Definition and Core Concepts:
The Beneish Model is a statistical model developed by Messod Beneish in his 1999 paper, "The Detection of Earnings Manipulation." It's based on the premise that companies engaging in earnings manipulation exhibit specific patterns in their financial ratios. These patterns are subtle and difficult to detect through traditional financial statement analysis alone. The model cleverly identifies these subtle anomalies, giving analysts a quantitative measure of the likelihood of earnings manipulation.
2. The Eight Financial Ratios and their Interpretation:
The Beneish Model incorporates eight financial ratios, each designed to capture a different aspect of earnings manipulation:
- Days Sales Receivable Index (DSRI): Measures the change in the number of days it takes a company to collect receivables. A higher value suggests potential revenue inflation.
- Gross Margin Index (GMI): Reflects the change in gross margins. A decreasing GMI may indicate cost-cutting measures or revenue recognition issues.
- Asset Quality Index (AQI): Indicates changes in the proportion of non-current assets. A deteriorating AQI can signify attempts to inflate assets.
- Sales Growth Index (SGI): Measures the growth rate of sales. Rapid sales growth can be a sign of aggressive revenue recognition practices.
- Depreciation Index (DEPI): Reflects changes in depreciation policies. A lower DEPI can mask declining asset quality.
- Leverage Index (LVGI): Measures changes in the company's leverage. An increasing LVGI can be a signal of financial distress and potential manipulation.
- Total Accruals to Total Assets (TATA): A crucial indicator, this ratio reflects the magnitude of accruals relative to total assets. High accruals often represent accounting manipulation.
- Return on Assets (ROA): A key measure of profitability, a declining ROA can be a red flag and, in conjunction with other indicators, point towards potential manipulation.
Each of these indices is calculated by dividing the current year's ratio by the previous year's ratio. A value greater than 1 generally signifies a negative trend, while values below 1 typically suggest a positive trend.
3. M-Score Calculation:
The M-score is a weighted average of the eight ratios, calculated as follows:
M-Score = -4.84 + 0.920 * DSRI + 0.528 * GMI + 0.404 * AQI + 0.892 * SGI + 0.115 * DEPI - 0.172 * LVGI + 4.679 * TATA - 0.327 * ROA
Where each variable represents the respective index calculated using the company's financial statements.
4. Interpretation of the M-Score:
The M-score is a continuous variable. A higher M-score indicates a greater probability of earnings manipulation. Generally:
- M-score > -1.78: Suggests a high probability of earnings manipulation.
- -1.78 > M-score > -2.22: Suggests a probability of earnings manipulation that requires further investigation.
- M-score < -2.22: Suggests a low probability of earnings manipulation.
5. Examples and Case Studies:
Let's illustrate the calculation with a simplified example. Assume a company has the following financial ratios for the current and previous years:
Ratio | Current Year | Previous Year | Index |
---|---|---|---|
DSRI | 1.2 | 1 | 1.2 |
GMI | 0.9 | 1 | 0.9 |
AQI | 1.1 | 1 | 1.1 |
SGI | 1.5 | 1 | 1.5 |
DEPI | 0.8 | 1 | 0.8 |
LVGI | 1.1 | 1 | 1.1 |
TATA | 0.2 | 0.1 | 2 |
ROA | 0.05 | 0.1 | 0.5 |
Plugging these values into the M-score equation:
M-Score = -4.84 + 0.920 * 1.2 + 0.528 * 0.9 + 0.404 * 1.1 + 0.892 * 1.5 + 0.115 * 0.8 - 0.172 * 1.1 + 4.679 * 2 - 0.327 * 0.5
M-Score ≈ 4.23
In this hypothetical case, the M-score of approximately 4.23 indicates a high probability of earnings manipulation. This would warrant a thorough investigation into the company's financial practices. Real-world applications require more complex data analysis and may involve multiple years of financial data to increase accuracy and reliability. Numerous academic studies have validated the efficacy of the Beneish Model in detecting fraudulent financial reporting, although it’s not foolproof.
6. Exploring the Connection Between Total Accruals and the Beneish Model:
The Total Accruals to Total Assets (TATA) ratio plays a crucial role within the Beneish Model. Accruals represent the difference between a company's reported earnings and its cash flows. Companies manipulating their earnings often engage in aggressive accrual accounting, artificially inflating their profits. The TATA ratio captures this aspect, making it a powerful predictor in the M-score calculation. The high weighting given to TATA within the M-score equation highlights its significance in identifying potential fraudulent activities.
Key Factors to Consider:
- Roles and Real-World Examples: The TATA ratio's role is demonstrated in numerous real-world cases of accounting scandals where companies used aggressive accrual accounting to inflate earnings.
- Risks and Mitigations: While the TATA ratio is a valuable indicator, it is not immune to manipulation itself. Sophisticated fraudsters may employ techniques to obscure aggressive accruals.
- Impact and Implications: The use of the TATA ratio, and the Beneish Model more broadly, has significant implications for investors, regulators, and auditors alike, providing a proactive tool for fraud detection and risk mitigation.
7. Conclusion: Reinforcing the Connection:
The connection between total accruals and the Beneish Model underscores the importance of analyzing a company's accounting practices beyond the surface level. Understanding accruals and their potential for manipulation is crucial for correctly interpreting the M-score and making informed investment decisions.
Further Analysis: Examining Total Accruals in Greater Detail:
Understanding accruals requires a deeper understanding of accounting practices. Accruals can be both legitimate and manipulative. Legitimate accruals arise from the timing differences between when revenue is earned and when cash is received (or when expenses are incurred and when cash is paid). Manipulative accruals, on the other hand, involve deliberate misrepresentation of accounting figures to create an artificially improved picture of a company’s financial position. Analyzing accrual quality, separating legitimate and manipulative accruals, is a complex task often requiring expert accounting knowledge. The Beneish Model, while providing a valuable overall measure of manipulation risk, doesn't directly distinguish between these types.
8. Limitations and Considerations:
While the Beneish Model offers a valuable tool for fraud detection, it’s crucial to acknowledge its limitations:
- Data Dependence: The model relies heavily on the accuracy and reliability of the financial data used in its calculation. Inaccurate or manipulated financial statements will lead to inaccurate M-scores.
- Model Complexity: The model's complexity may be challenging for those without a strong financial background.
- Not a Foolproof Indicator: The M-score is a probability indicator, not a definitive measure of fraud. A high M-score should trigger further investigation, not automatically conclude fraud.
- Industry-Specific Variations: The effectiveness of the Beneish Model may vary across industries due to differences in accounting practices and financial reporting standards.
FAQ Section:
Q: What is the Beneish Model?
A: The Beneish Model is a statistical model that uses financial ratios to predict the likelihood of earnings manipulation.
Q: How accurate is the Beneish Model?
A: The accuracy of the Beneish Model varies. While studies have shown it to be effective in identifying potentially manipulative companies, it's not a foolproof measure and should be used in conjunction with other analytical methods.
Q: Can I use the Beneish Model to detect all types of financial fraud?
A: No, the Beneish Model primarily focuses on earnings manipulation. It may not be effective in detecting other types of financial fraud, such as asset misappropriation.
Q: What are the limitations of the Beneish Model?
A: The Beneish Model has limitations such as its reliance on accurate financial data, its complexity, and its potential for variations across industries.
Practical Tips:
- Use the Beneish Model in conjunction with other fraud detection techniques, such as qualitative analysis and forensic accounting.
- Verify the data used in the calculation and ensure its reliability.
- Consider the industry context when interpreting the M-score.
- Do not rely solely on the M-score to make investment decisions.
Final Conclusion:
The Beneish Model represents a significant advancement in financial statement analysis, providing a quantitative framework for assessing the probability of earnings manipulation. By understanding its components, limitations, and applications, investors, auditors, and analysts can utilize this powerful tool to enhance their risk management strategies and make more informed decisions in a complex and often opaque financial environment. However, the model should be viewed as one piece of the puzzle in a thorough and holistic assessment of a company's financial health and integrity. Continuous vigilance and a multi-faceted approach are essential for effective fraud detection and mitigation.
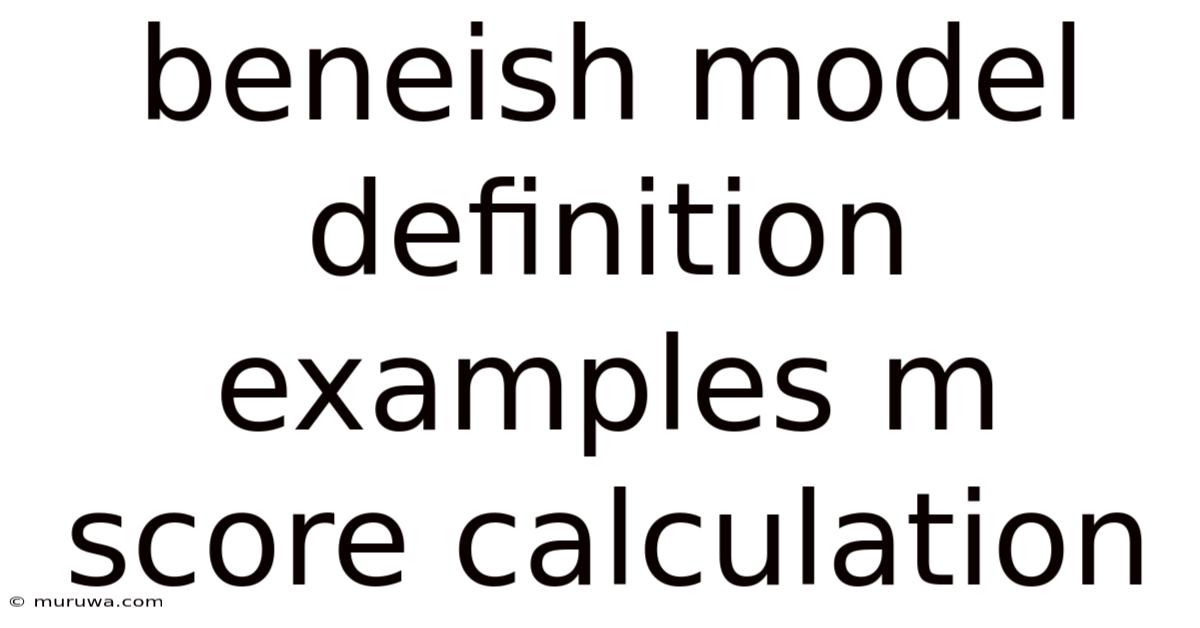
Thank you for visiting our website wich cover about Beneish Model Definition Examples M Score Calculation. We hope the information provided has been useful to you. Feel free to contact us if you have any questions or need further assistance. See you next time and dont miss to bookmark.
Also read the following articles
Article Title | Date |
---|---|
What Is Big Data Definition How It Works And Uses | Apr 21, 2025 |
What Is An Lp Investment | Apr 21, 2025 |
What Is An Endowment Life Insurance Policy | Apr 21, 2025 |
How To Remove Keratin Bonds At Home | Apr 21, 2025 |
Below Market Interest Rate Bmir Definition | Apr 21, 2025 |