Benchmark For Correlation Values Definition
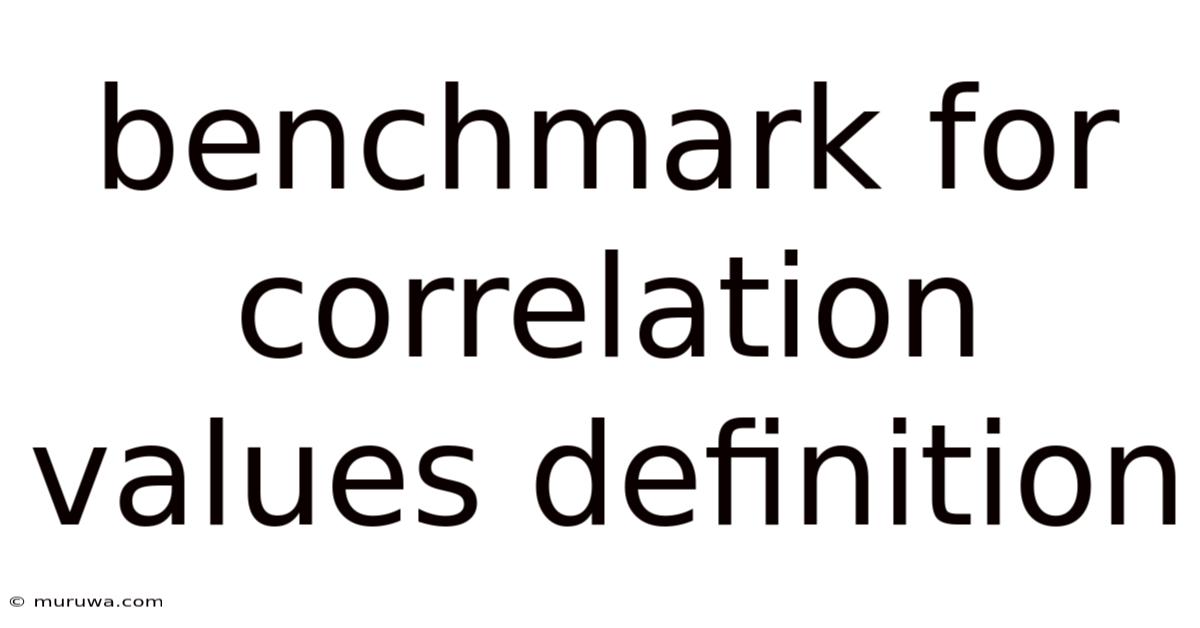
Discover more detailed and exciting information on our website. Click the link below to start your adventure: Visit Best Website meltwatermedia.ca. Don't miss out!
Table of Contents
Benchmarking Correlation Values: Defining Meaningful Thresholds in Data Analysis
What if the seemingly arbitrary nature of correlation coefficients hides crucial insights into the strength of relationships? Establishing clear benchmarks for correlation values is not merely a statistical formality; it’s a gateway to unlocking the true meaning and practical implications of your data.
Editor’s Note: This article on benchmarking correlation values provides a comprehensive guide for interpreting correlation coefficients across various disciplines. Updated research and examples are included to ensure relevance and practicality.
Why Benchmarking Correlation Values Matters:
Understanding the strength of relationships between variables is fundamental to numerous fields. In finance, it helps predict market movements. In healthcare, it assists in identifying risk factors for diseases. In engineering, it aids in optimizing designs. However, simply calculating a correlation coefficient (like Pearson's r, Spearman's rho, or Kendall's tau) is insufficient. Without benchmarks, a correlation of 0.3 might be considered strong in one context but weak in another. Benchmarking provides a standardized framework for interpreting these values, leading to more robust and reliable conclusions. The lack of universally accepted benchmarks often leads to misinterpretations, hindering effective decision-making. This article aims to address this gap by providing a clear understanding of how to interpret correlation coefficients within their respective contexts.
Overview: What This Article Covers:
This article will explore the definition and interpretation of various correlation coefficients. It will then delve into the challenges of establishing universal benchmarks, focusing on context-specific interpretations and the influence of sample size and data distribution. We'll examine the practical implications of misinterpreting correlation, and provide guidelines for setting appropriate benchmarks in different fields. Finally, we'll explore common misconceptions and offer actionable advice for researchers and data analysts.
The Research and Effort Behind the Insights:
This article synthesizes information from numerous statistical textbooks, research articles, and industry best practices. It draws upon decades of statistical research to present a balanced and nuanced perspective on interpreting correlation coefficients. The examples provided are drawn from real-world applications to illustrate the practical implications of proper benchmarking.
Key Takeaways:
- Definition and Core Concepts: Understanding the different types of correlation coefficients and their limitations.
- Context-Specific Benchmarks: Recognizing that benchmarks vary across disciplines and research questions.
- Sample Size and Data Distribution: The impact of these factors on correlation strength and interpretation.
- Practical Applications: Illustrative examples from various fields showcasing the importance of proper interpretation.
- Common Misconceptions: Debunking common misunderstandings surrounding correlation and causation.
Smooth Transition to the Core Discussion:
With a foundational understanding of the importance of benchmarking correlation values, let’s now delve into the specific nuances of interpretation and the challenges of establishing universally applicable thresholds.
Exploring the Key Aspects of Benchmarking Correlation Values:
1. Definition and Core Concepts:
Correlation analysis quantifies the strength and direction of a linear relationship between two or more variables. Several coefficients exist, each suitable for different data types:
-
Pearson's r: Measures the linear relationship between two continuous variables. Ranges from -1 (perfect negative correlation) to +1 (perfect positive correlation), with 0 indicating no linear relationship.
-
Spearman's rho: Measures the monotonic relationship between two ordinal or continuous variables. Also ranges from -1 to +1, but is less sensitive to outliers than Pearson's r.
-
Kendall's tau: Another measure of monotonic relationship, less sensitive to outliers than Spearman's rho, often preferred for smaller datasets.
2. Context-Specific Benchmarks:
There's no single universally agreed-upon benchmark for interpreting correlation coefficients. The strength of a correlation is heavily dependent on the field of study and the specific research question. For instance:
-
Social Sciences: A correlation of 0.3 might be considered moderately strong, reflecting the complexity of human behavior and the influence of numerous confounding variables.
-
Physics/Engineering: A correlation of 0.8 or higher might be necessary to establish a reliable relationship between physical quantities, given the precise nature of measurements and the expectation of strong deterministic relationships.
-
Finance: In high-frequency trading, even small correlations (e.g., 0.1) can be significant due to the volume and speed of transactions.
3. Sample Size and Data Distribution:
The sample size significantly influences the reliability of a correlation coefficient. With smaller samples, even a strong correlation might be due to chance. Large sample sizes increase statistical power, leading to smaller correlations being statistically significant. Furthermore, the distribution of the data can impact the correlation. Non-normal distributions can inflate or deflate correlation coefficients, necessitating careful consideration.
4. Impact on Innovation:
Proper benchmarking facilitates innovation by providing a clearer understanding of variable relationships. In machine learning, for instance, feature selection relies heavily on correlation analysis. By accurately benchmarking, researchers can select the most relevant features, leading to improved model accuracy and efficiency.
Closing Insights: Summarizing the Core Discussion:
Benchmarking correlation values is a crucial step in data analysis. Without context-specific interpretation, correlation coefficients can be easily misinterpreted, leading to flawed conclusions. The interplay of sample size, data distribution, and the field of study must be carefully considered when establishing meaningful thresholds.
Exploring the Connection Between Statistical Significance and Practical Significance:
Statistical significance, indicated by a p-value, simply tells us whether a correlation is likely due to chance. However, practical significance assesses the magnitude and real-world impact of the correlation. A statistically significant correlation might be practically insignificant if the correlation coefficient is very small. For example, a correlation of 0.05 might be statistically significant with a very large sample size but holds little practical value. The interplay between statistical and practical significance is crucial for responsible data interpretation.
Key Factors to Consider:
-
Roles and Real-World Examples: Consider a medical study investigating the correlation between blood pressure and cholesterol levels. A statistically significant but weak correlation (e.g., r = 0.2) might not be clinically meaningful, while a strong correlation (e.g., r = 0.7) would have substantial clinical implications.
-
Risks and Mitigations: Overreliance on a single correlation coefficient without considering other factors (e.g., confounding variables, sample size) can lead to incorrect inferences. Careful study design and robust statistical methods are essential to mitigate these risks.
-
Impact and Implications: Misinterpreting correlations can have significant consequences. In financial modeling, for instance, faulty correlation assumptions can lead to inaccurate risk assessments and potentially catastrophic losses.
Conclusion: Reinforcing the Connection:
The relationship between statistical significance and practical significance is paramount in interpreting correlation coefficients. Simply relying on p-values is insufficient. Researchers and analysts must carefully assess the magnitude of the correlation coefficient, considering the context, sample size, and potential confounding variables to determine its practical relevance.
Further Analysis: Examining the Role of Confounding Variables:
Confounding variables are factors that influence both variables being correlated, creating a spurious association. For example, ice cream sales and drowning incidents are positively correlated, but this doesn't mean ice cream causes drowning. The confounding variable is summer weather—higher temperatures lead to increased ice cream sales and more swimming, resulting in more drownings. Controlling for confounding variables through techniques like regression analysis is essential for accurate interpretation of correlations.
FAQ Section: Answering Common Questions About Benchmarking Correlation Values:
Q: What is the best benchmark for correlation values?
A: There is no single "best" benchmark. The appropriate benchmark depends heavily on the context, field of study, sample size, and research question.
Q: How do I determine the practical significance of a correlation?
A: Consider the magnitude of the correlation coefficient in relation to the context. A small correlation might be practically insignificant in some fields but highly relevant in others. Also, consider the potential impact of the relationship.
Q: What are some common errors in interpreting correlation coefficients?
A: Common errors include assuming causation from correlation, ignoring confounding variables, and neglecting the influence of sample size and data distribution.
Practical Tips: Maximizing the Benefits of Correlation Analysis:
-
Clearly define your research question: This will help you select the appropriate correlation coefficient and establish relevant benchmarks.
-
Assess your data: Check for outliers, non-normality, and potential confounding variables.
-
Consider sample size: Larger samples provide more reliable results.
-
Interpret correlations in context: Don't rely solely on numerical values; consider the field of study and practical implications.
-
Consult with a statistician: For complex analyses, seeking expert advice is crucial.
Final Conclusion: Wrapping Up with Lasting Insights:
Benchmarking correlation values requires a nuanced understanding of statistical principles, contextual factors, and practical implications. By carefully considering these elements, researchers and data analysts can draw meaningful conclusions from correlation analyses, leading to more informed decision-making and advancements across various disciplines. The seemingly simple act of interpreting a correlation coefficient is, in reality, a complex process requiring careful consideration and a critical eye. A thorough understanding of these principles is essential for responsible data analysis and the extraction of valuable insights from complex datasets.
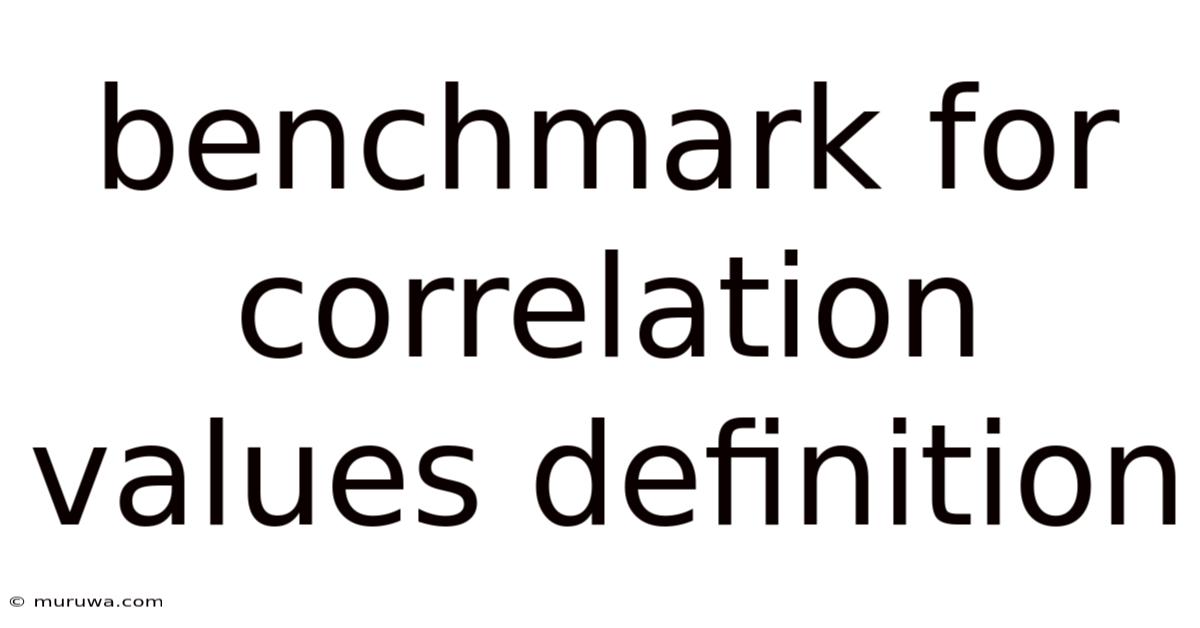
Thank you for visiting our website wich cover about Benchmark For Correlation Values Definition. We hope the information provided has been useful to you. Feel free to contact us if you have any questions or need further assistance. See you next time and dont miss to bookmark.
Also read the following articles
Article Title | Date |
---|---|
What Is Single Premium Life Insurance | Apr 21, 2025 |
What Is The Main Disadvantage Of Having Whole Life Insurance | Apr 21, 2025 |
Base Currency Definition Example Vs Quote Currency | Apr 21, 2025 |
How Much Is A Fidelity Bonds Cost | Apr 21, 2025 |
How Long Does A Life Insurance Company Have To Investigate A Claim | Apr 21, 2025 |