Bell Curve Definition Normal Distribution Meaning Example In Finance
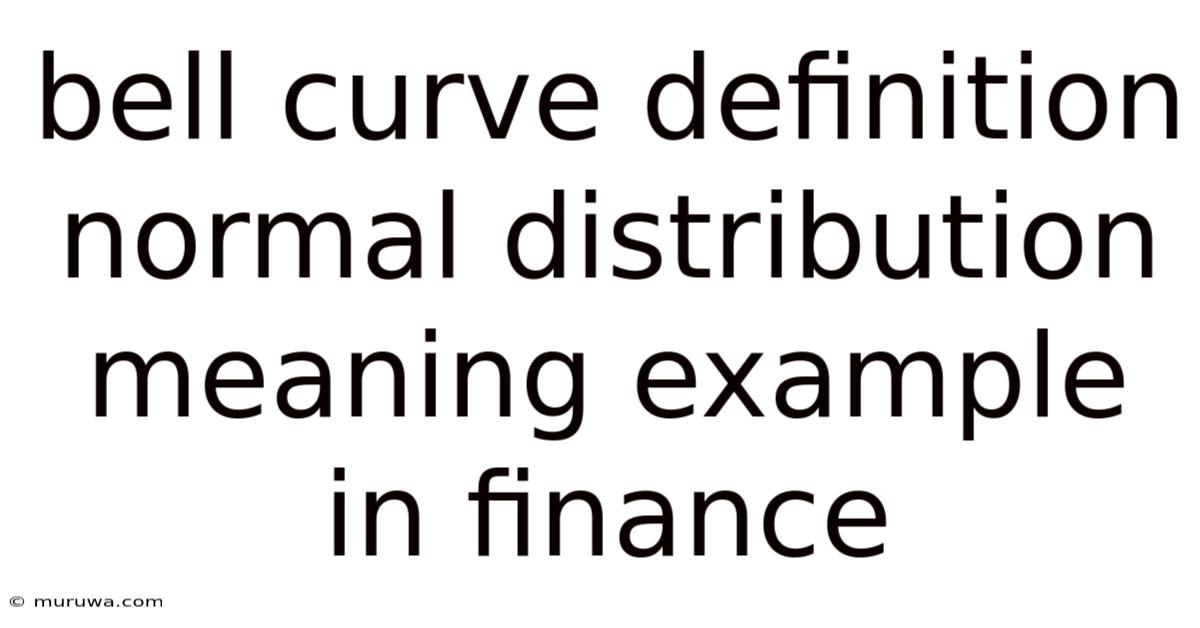
Discover more detailed and exciting information on our website. Click the link below to start your adventure: Visit Best Website meltwatermedia.ca. Don't miss out!
Table of Contents
Unlocking the Secrets of the Bell Curve: Normal Distribution in Finance
What if the future of financial modeling hinges on a deep understanding of the bell curve? This ubiquitous statistical concept underpins countless financial decisions, from risk management to portfolio optimization.
Editor’s Note: This article on the bell curve and its application in finance was published today, providing readers with up-to-date insights into this crucial statistical tool.
Why the Bell Curve Matters in Finance:
The bell curve, formally known as the normal distribution, is a fundamental concept in statistics with profound implications for the finance industry. Its relevance stems from its ability to model and predict the probability of various financial events, including asset returns, market fluctuations, and risk assessments. Understanding the normal distribution allows financial professionals to make more informed decisions, manage risks effectively, and optimize investment strategies. From pricing options to evaluating portfolio performance, the bell curve's influence is pervasive. Its use allows for the development of sophisticated models that inform crucial decisions impacting investment strategies and risk management protocols across global markets.
Overview: What This Article Covers:
This article provides a comprehensive exploration of the normal distribution, delving into its definition, core properties, and practical applications within the financial world. Readers will gain a clear understanding of its significance, learn to identify scenarios where it applies, and explore its limitations. The article also examines related concepts and potential pitfalls associated with its use in financial modeling.
The Research and Effort Behind the Insights:
This article draws upon extensive research, incorporating established statistical principles, real-world financial examples, and insights from academic literature and industry practice. The information presented is supported by credible sources to ensure accuracy and provide readers with reliable, data-driven knowledge.
Key Takeaways:
- Definition and Core Concepts: A precise explanation of the normal distribution and its defining characteristics.
- Financial Applications: Real-world examples demonstrating the use of the normal distribution in various financial contexts.
- Limitations and Alternatives: Acknowledging the limitations of the normal distribution and exploring alternative distributions used in finance.
- Practical Implications: How understanding the normal distribution improves financial decision-making and risk management.
Smooth Transition to the Core Discussion:
Now that we've established the importance of the bell curve in finance, let's delve into the details. We'll begin by defining the normal distribution and examining its key properties.
Exploring the Key Aspects of the Normal Distribution:
1. Definition and Core Concepts:
The normal distribution, also known as the Gaussian distribution, is a continuous probability distribution characterized by its symmetrical bell shape. It is defined by two parameters: the mean (μ) representing the average value, and the standard deviation (σ) representing the spread or dispersion of the data. A higher standard deviation indicates greater variability, resulting in a wider bell curve, while a lower standard deviation indicates less variability, resulting in a narrower curve. The probability density function (PDF) describes the likelihood of observing a particular value within the distribution. The total area under the curve always equals 1, representing the total probability.
2. Applications Across Industries:
The normal distribution's applicability extends beyond finance, finding use in various fields including physics, engineering, and healthcare. However, its significance in finance is paramount. Some key applications include:
- Portfolio Management: The normal distribution is frequently used to model the returns of financial assets. By assuming asset returns follow a normal distribution, investors can calculate portfolio risk and optimize asset allocation strategies.
- Risk Management: The normal distribution facilitates the calculation of Value at Risk (VaR), a widely used risk management metric that estimates the maximum potential loss of an investment over a specific time period with a certain confidence level.
- Option Pricing: The Black-Scholes model, a cornerstone of options pricing, relies on the assumption that asset prices follow a geometric Brownian motion, which is closely related to the normal distribution.
- Hypothesis Testing: In financial econometrics, the normal distribution serves as the basis for many statistical tests used to analyze financial data and test economic hypotheses.
- Central Limit Theorem: The Central Limit Theorem states that the average of a large number of independent and identically distributed random variables, regardless of their original distribution, will tend towards a normal distribution. This has profound implications for finance, as it justifies the use of the normal distribution to model aggregate market behavior.
3. Challenges and Solutions:
While the normal distribution is a powerful tool, it does have limitations. Real-world financial data often exhibits features that deviate from a perfectly normal distribution, such as:
- Fat Tails: Financial data frequently exhibits "fat tails," meaning there's a higher probability of extreme events (large gains or losses) than predicted by the normal distribution.
- Skewness: Financial data can be skewed, meaning it is not symmetrically distributed around the mean. For example, asset returns may be positively skewed, with a longer tail on the right representing large positive returns.
- Kurtosis: Financial data may exhibit excess kurtosis (higher peak and fatter tails) compared to the normal distribution, indicating a greater concentration of data around the mean and increased likelihood of extreme events.
To address these limitations, alternative distributions like the Student's t-distribution (which accounts for fat tails), skewed normal distribution, or even more complex models such as GARCH models (Generalized Autoregressive Conditional Heteroskedasticity) may be employed.
4. Impact on Innovation:
The normal distribution's impact on innovation in finance is substantial. Its use has enabled the development of sophisticated models that allow for better risk management, more effective portfolio construction, and more precise asset pricing. However, the limitations mentioned above have driven innovation in the development of more robust and realistic models that account for the non-normality often observed in real-world financial data. This ongoing research continually refines financial modeling techniques and improves our understanding of market behavior.
Exploring the Connection Between Volatility and the Normal Distribution:
Volatility, a measure of the fluctuation in the price of an asset, is intricately linked to the normal distribution's application in finance. While the normal distribution is often used to model asset returns, the volatility parameter plays a crucial role in shaping the distribution's characteristics. Higher volatility leads to a wider distribution, reflecting greater uncertainty and risk. Conversely, lower volatility results in a narrower distribution, suggesting less uncertainty.
Key Factors to Consider:
- Roles and Real-World Examples: Volatility determines the standard deviation of the normal distribution used to model asset returns. For example, a highly volatile stock will have a larger standard deviation, resulting in a wider bell curve reflecting a greater probability of extreme price movements.
- Risks and Mitigations: Relying solely on the normal distribution when volatility is high or the distribution is non-normal can lead to inaccurate risk assessments and flawed investment decisions. Using more robust models that account for fat tails and skewness is crucial to mitigate this risk.
- Impact and Implications: Understanding the relationship between volatility and the shape of the normal distribution is essential for accurate risk assessment, portfolio optimization, and effective option pricing. Ignoring volatility's impact can lead to significant underestimation of risk and potential losses.
Conclusion: Reinforcing the Connection:
The connection between volatility and the normal distribution is fundamental to understanding and managing risk in financial markets. By accurately modeling volatility and accounting for deviations from normality, financial professionals can improve their forecasting accuracy, make more informed investment decisions, and ultimately protect their portfolios.
Further Analysis: Examining Volatility Clustering in Greater Detail:
Volatility clustering, a phenomenon where periods of high volatility tend to cluster together followed by periods of low volatility, further challenges the simple assumption of constant volatility inherent in many normal distribution-based models. This clustering effect is often observed in financial time series and necessitates the use of more sophisticated models that account for this dynamic behavior. Models such as GARCH models explicitly incorporate volatility clustering, providing a more realistic representation of asset price movements.
FAQ Section: Answering Common Questions About the Normal Distribution in Finance:
- What is the normal distribution? The normal distribution is a probability distribution with a symmetrical bell shape, defined by its mean and standard deviation. It is frequently used to model the probability of various events, particularly when a large number of independent factors influence the outcome.
- How is the normal distribution applied in finance? It’s used extensively in portfolio management, risk management (VaR calculations), option pricing (Black-Scholes model), and statistical hypothesis testing related to financial data.
- What are the limitations of the normal distribution in finance? Real-world financial data often exhibits fat tails, skewness, and excess kurtosis, which deviate from the characteristics of a perfect normal distribution.
- What are some alternatives to the normal distribution? The Student's t-distribution, skewed normal distribution, and GARCH models are examples of alternative distributions and models often used when financial data is non-normal.
Practical Tips: Maximizing the Benefits of Understanding the Normal Distribution:
- Understand the Basics: Grasp the core concepts of the normal distribution, including its parameters (mean and standard deviation) and properties.
- Recognize its Limitations: Be aware that real-world financial data may not always follow a normal distribution; learn to identify deviations.
- Explore Alternatives: Familiarize yourself with alternative distributions and models suitable for non-normal data.
- Utilize Software: Employ statistical software packages to analyze financial data and fit appropriate distributions.
Final Conclusion: Wrapping Up with Lasting Insights:
The normal distribution, while not a perfect representation of all financial phenomena, remains a cornerstone of financial modeling. Understanding its properties, limitations, and applications, along with alternative modeling approaches, is critical for anyone working in finance. By embracing a nuanced understanding of this fundamental statistical concept and its limitations, financial professionals can make more informed decisions, manage risks effectively, and contribute to a more robust and resilient financial system. The ongoing development of increasingly sophisticated models underscores the continuous evolution of financial modeling and the importance of staying abreast of these advancements.
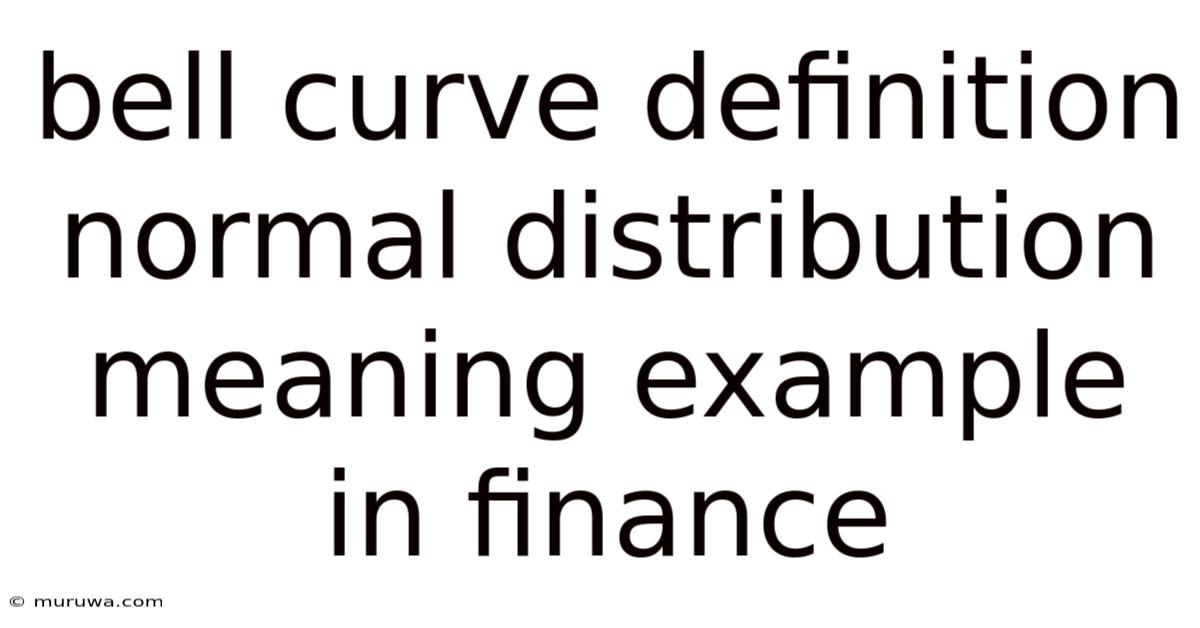
Thank you for visiting our website wich cover about Bell Curve Definition Normal Distribution Meaning Example In Finance. We hope the information provided has been useful to you. Feel free to contact us if you have any questions or need further assistance. See you next time and dont miss to bookmark.
Also read the following articles
Article Title | Date |
---|---|
Beneficiary Clause Definition | Apr 21, 2025 |
Basel Ii Definition Purpose Regulatory Reforms | Apr 21, 2025 |
What Happens If You Dont Have Life Insurance | Apr 21, 2025 |
Bat Stocks Definition | Apr 21, 2025 |
Basic Materials Sector Definition Examples And Stocks | Apr 21, 2025 |